AI Principles in Practice with Ansgar Koene
[MUSIC PLAYING] KIMBERLY NEVALA: Welcome to Pondering AI. My name is Kimberly Nevala. I'm a strategic advisor at SAS and your host this season as we contemplate the imperative for responsible AI. Each episode will be joined by an expert to explore a different aspect of the ongoing quest to ensure that artificial intelligence is deployed fairly, safely and justly, now and in the future. Today I'm so excited to be joined by Dr. Ansgar Koene. Ansgar is the global AI ethics and regulatory leader at Ernst Young, also known as EY. He is also a senior research fellow at the University of Nottingham and chair of the working group for IEEE's P7003 standard for algorithm bias considerations. Welcome, Ansgar.
ANSGAR KOENE: Great to be on the call.
KIMBERLY NEVALA: Now, your interests have ranged from cybersecurity to data privacy for children to the impacts of AI on public service and the changing environment for news, social media and journalism. What was the initial spark that got you involved in this topic overall, and is there a common thread that ties all of these interests together?
ANSGAR KOENE: So I actually started my research career. For a long time up until two years ago I was purely an academic, and the research was really on the intersection between psychology neuroscience and robotics.
KIMBERLY NEVALA: Wow.
ANSGAR KOENE: So doing biologically inspired robots and using robotics computational modeling to test our ideas about how the brain works. After a number of years, especially working on this intersection between domains, I was observing sort of the challenges of accessing the kinds of data roboticists would really love to be able to get better information about, things like how children learn to walk and those kinds of things, which child psychologists and behavioral scientists have data about, but they publish in different kinds of journals than roboticists, et cetera. So we're starting to look at information sharing between disciplines and those kinds of things, and that got me interested into computational social science, the use of online data for trying to understand and model human behavior, but that fairly rapidly then led to the questions about the ethics around this.
It is great if as a linguist you can access Twitter data to follow the way in which a person is using their language and create a model about them as coming from a certain region or something like that, but if you sit back and you think, the person who actually created this data, the person who was having a conversation on Twitter, how do they actually feel about you having done that kind of a potentially intimate analysis of them as a person? If you're communicating on Twitter, it's a bit like communicating to somebody in a pub. You are aware the people around you can hear this. That's fine, but would you feel comfortable if somebody was sitting behind you with a tape recorder, recording everything that you're saying and then going to do some kind of analysis? Probably not.
So that really led to thinking about the ethics around the use of online data, but while we were looking at this and specifically looking at the ethics review process within universities and the fact that if you're doing the same project in the psychology department or in the computer science department, or in the business school, it might go through a very different review processes. While looking at this, and especially I was at a conference where they were talking about recommender systems, I started thinking about also the way, of course, how with recommender systems and machine learning and these kinds of approaches the data that you're producing is actually also shaping the online world that you're being exposed to. And so that led to a research project around bias and algorithmic systems, and really, bias, there are not necessarily the strictly negative impacts, but more it's pushing you in a certain kind of direction that you might not be aware of, kind of concept.
KIMBERLY NEVALA: You're right. We do often talk about bias as if it's a blunt instrument, and one that is wielded only or primarily in the interest of overtly negative outcomes. So how did you approach the issue of bias differently in this study?
ANSGAR KOENE: Well, the key question was really about, how do people feel about the way in which these things operate? So things around algorithmic literacy, things around, what are the concerns especially of younger people, the so-called digital literate? I mean, they've grown up with digital technologies, they're great at using it, but that doesn't necessarily mean that they understand what's going on behind the scenes. And so we really engage with 13 to 17-year-olds about how they are using this technology and what they would like to see different. And really it was about giving them the voice as opposed to adults talking down to them, which raised very interesting discussions between them.
There were some teenagers having very deep discussions about, does Snapchat hold your data for longer than 24 hours if it's been supposedly deleted, and those kinds of things. And they'd both done their own research and come to different conclusions, and therefore were having an interesting discussion about it. So that was an area, but when we did this research project, we wanted to make sure that the kind of findings would not stay in the academic bubble.
We wanted to make sure that we can communicate this to policymakers and to industry. And so that's how I got involved in the IEEE standard that you mentioned, the algorithmic bias considerations standard, which is really looking to address these kinds of questions around algorithmic bias, not from a, industry is doing this wrong, it's doing that wrong, but rather from a, but what should you actually be doing, kind of perspective. What would be the process that you should follow in order to make sure that the system that you're building is going to take into account the particular sensitivities of the stakeholders that you're going to be dealing with, is going to know which data do I actually need to be collecting in order for it to be representative, given the particular use case?
Because the context specificity, that is always the big challenge when it comes to the ethics kinds of dimensions of this kind of technology. You can't really say, don't do this, but it's generally a, don't do this if that's where you're intending to be using it and if those are the ones that are going to be impacted by it. You need to be thinking in that kind of bigger picture perspective, and that's also where a lot of the challenges come from for implementing it. So you need that multidisciplinary kind of approach to it. It's been great with the standards development working group that we have a very multidisciplinary group.
KIMBERLY NEVALA: There's certainly a lot of focus on the need for diversity in all aspects of AI. So what does a multidisciplinary, diverse approach look like in this context?
ANSGAR KOENE: We did a little kind of survey. We have about one third, comes from industry, one third from academia, one third more from sort of the NGO civil society space. We have people from computer science. We have people from the humanities. We have people from business kind of dimensions, et cetera.
So it's a great mix. Obviously, that raises certain challenges from, how do we communicate with each other? Every domain has its own language, but it generates this kind of perspective of, actually these kinds of things are cultural-specific, or so we need to be raising awareness around cultural specificity, so we have an annex regarding that kind of thing, et cetera. And you know, that goes into that kind of question about, how do we actually build the right team if we're going to be working on a thing?
We need to have a diverse team, but what does diversity mean? Sure, we need to not only be men on the team, but do we need to make sure that we have representatives from all races on the globe? Or maybe we can think about, actually this is going to be used in this space, and we just need to make sure that we really understand those stakeholders. And we won't always be able to have all of those people on our team, so what kind of process can we put in place to engage with people who know what the sensitivities are of the stakeholders if we couldn't get them onto our team? Those kinds of questions.
KIMBERLY NEVALA: You talked about the ability for these systems to really shape the environment, like the political, the social environment that we work in, and how they are really even driving and, in some cases, actively engaging with the evolution of things like, how do we actually deliver and consume news? Or what does journalism really look like? Now, certainly that particular space doesn't have a corner on all of the ethical issues that can arise with AI, but it certainly does shine a light on many of the more, perhaps, intractable or inherent ones. Are there some examples in that space you can share that highlight some of the thornier issues that really are arising with artificial intelligence today?
ANSGAR KOENE: Sure, so one of the big challenges when it comes to things like how recommender systems are nudging people into a certain kind of space of information that they're receiving is, well, it's obviously connected to the issue of the filter bubble idea. But the real challenge when it comes to trying to regulate this is that it's not the single instance that you can look at. You can't say, well, this particular output of the system caused the problem, but it's the accumulation over time, over the multiple kinds of recommendations that you're getting that is gradually nudging you in a certain kind of way because you've seen these types of things. You respond in a certain kind of way, and that nudges you even further, and that's sort of driving this further polarization of the information spheres that people find themselves in. But you can't really say, it was this particular point.
And for instance, if we look at the really well-crafted piece of new proposed legislation from the European Commission around AI, which is all around risks and identifying the risk kind of thing, how do you identify the risk of something when it is an accumulation over time that is the thing that is actually causing the problem, where you can't say, this particular? If you're doing a credit assessment, that's one point in time at which you can say, the denial of that loan had an immediate impact on somebody's life abilities, but if it's the general flow of what you are being exposed to then where is that? And of course we get into the very thorny kind of questions. Yes, but who should be making the decision? What is the right kind of information that you should have been seeing?
What is clear, however, I think, is that you cannot position yourself as an organization that is in this space of communicating media and say, but we didn't produce the content, all we're doing is having an algorithm that flags up the things you seem to be interested in, and then claim that you don't have any editorial responsibility for what is going on. So I was writing about this back in 2006, that editorial responsibility of social media organizations by the fact that maybe you're not deleting the content, but you are choosing what goes in the front page of the newspaper, for those people who still read paper-based newspapers.
KIMBERLY NEVALA: I do miss a good book in my hands, I have to say. I'll shuffle them just for that purpose. So can we extend that concept also to-- and certainly the conversation has evolved-- where organizations are saying, who should be responsible or accountable for the output of any algorithm, maybe outside of this realm, but in something that is recommending credit or no credit, or is making a suggestion about a product and/or deciding, right, who and what gets the different kinds of treatments for public services?
When we think about that accountability, there's a tendency to say, well, maybe we're not sure. We don't know what the inner workings are. This is just all the information that went into it, and this conversation about who should be accountable has gone around and around a few times. And in your mind, who ultimately needs to be accountable, and what do some of these issues tell us about the balance of power, for lack of a better word, or agency between, for instance, consumers and creators of these types of systems?
ANSGAR KOENE: Sure, I think that word, power, is a very important one because ultimately accountability needs to lie there where there was an ability to make a different choice to affect something around this. So if we're talking about who has accountability within the organization, we cannot say the accountability lies with the guy in the data science team who happen to write this one piece of line of code if that person didn't have any power around what this system was supposed to be built for, where it's going to be deployed, and those kinds of questions. Accountability must be held by that kind of level within the organizations that has the option of saying, you know what, we've actually thought about this system. It's not actually possible to build this in the right kind of way. We shouldn't be doing this, and that kind of position.
But then also, of course, a large part is the supply chain kind of question. A lot of organizations aren't building their own AI systems, certainly not from scratch. They are using third party tools that may be tuned. They may be trained differently for their particular application space, but a lot of the way in which the system behaves has actually been built by somebody else. Nevertheless, it is this final sort of point in the supply chain that has the access to what is the intended use behind this, actually. I mean, I think that is one of the nice concepts within the AI regulation that the commission came out with, is really framing it around intended use.
Obviously there is the challenge of the potential that actual use might diverge from intended use, but intended use also by the user, not just builder of the system, is I think a key part because that gets back to this, the context specificity. So a big part of the accountability is going to have to be with whoever it that is putting it into the use. Now obviously, that party needs to have the relevant information to be able to make that assessment, and so that's where things go down the supply chain, providing the sufficient information about, yes, we built it for this particular context. We did an assessment in this particular context.
So when we use whatever standard it is that's going to help us to minimize bias, for instance, we did that in a particular context. So if you're going to use it now, if you're going to put into your application, if you use it in that context, we're giving you certain guarantees. But if you're going to use it in a different context, sorry, but actually that's not what it was built for. So we can't give you any guarantees for that. It will need to be reassessed, and that's going to be a key part of the whole informity assessment, and those kinds of aspects to these things.
And then where did we get the data? Was this data actually produced for the use that you're going to be putting it to? What we're seeing a lot with AI systems at the moment is because they need so much data, they're just acquiring the data from wherever you can, as opposed to collecting it specifically for that particular purpose.
Now, this often doesn't necessarily cause a problem, certainly not if there are enough potential sources where you can get it from so that you can find one that matches your use case. But sometimes it doesn't produce those kinds of data biases because it wasn't actually for that purpose. If we're just going to pull in data from whatever photo sharing site we happen to be able to get access to, and that photo sharing site is used in a particular country primarily, therefore it's going to have mostly faces representative of that kind of a demographic. And if you're going to then apply this in a different kind of context, it's not going to work for face recognition kinds of thing, for instance. So really understanding the specificities of what the data meant and what the system was built for, and making sure that matches the intended use.
And then of course, making sure that also we don't get final end users who are using it for completely different purposes and potentially harmful to them without knowing it. Communication as always is a key element here. The different parties need to communicate what they've done and be transparent about where this is supposed to work and how it's supposed to work, and that includes communicating to the end user, to tell them, if you're going to use this for something else, sorry, but that's not what it's meant for, and it could potentially harm you. So really shouldn't.
KIMBERLY NEVALA: Now, that seems like an easier conversation when the end user is an employee or a partner, or somebody that we're engaging with, but you mentioned earlier the research you've done with citizens and particularly younger individuals where I wonder in some cases, if as they're engaging, for instance, with Twitter, and we are eavesdropping on that conversation, as you said earlier, if they're really translating that. In their mind, that's like the conversation in the cafe, and they're not necessarily translating to how that really works behind the scenes in the digital realm. I believe you or one of your colleagues said that you found that they were digitally engaged, but not necessarily digitally informed. So how important is it, and how do we go about making sure that our understood intent in context is, in fact, the same understanding that the end user has, particularly when we're talking about citizens or individuals completely outside of the organization? And maybe we have good intention to serve them, maybe we don't, but let's assume we do. How do we actually ensure that? And then it seems obvious that it's nice to talk to Shalini Kantayya in the last episode, and she said to use my face to buy a candy bar. That's convenient at the moment, but to your point about accumulated effects, right, it's not just about that one transaction. It's about accumulation. How do we actually communicate and ensure there is an equal understanding and agreement with this audience?
ANSGAR KOENE: Sure, these are very important challenges. One thing is, structurally, we need to be generating a better algorithmic literacy amongst the citizenry. Finland is doing some really interesting work in that space.
As part of the research project with young people, we also developed a toolkit around algorithmic awareness raising, taking a very simple and a very low tech approach using a deck of cards, actually. But just little games that are asking a question like, imagine you are the algorithm and you're trying to do something, just gets you to think about, actually I need these pieces of data in order to be able to do the kind of task. But if I'm doing that, I can go wrong easily because of this and this, those types of understanding the fundamentals behind.
But also raising the question about, what are the business models behind these kinds of things? If you're apparently getting something for free, how is this being maintained? There must be something where it isn't free anymore, otherwise how would they be able to pay for the upkeep and running this kind of system, those kinds of questions?
But there's only so far that you can go with something like literacy for the population. No matter how good you try to do, you cannot expect the 10-year-old to be thinking, big picture, what are the implications of my using a social media and putting certain pieces of information into that kind of a space? And it's similar to what we say in the offline world. Adults need to take certain kinds of responsibility for the young, and we need to translate adult in this case to those who have the ability to understand what's going on.
And we've seen this now in the UK with the Age Appropriate Design act that is saying, we recognize actually that putting all of the obligation on the end user to decide whether or not to consent to something doesn't work, especially with these kinds of age related vulnerabilities. And so therefore if you are the provider, you actually need to take certain kinds of responsibilities for that, and you need to be monitoring. Even I said the intended user of my system is 13 years or older, but I am completely aware that a huge percentage of my users are actually below that age, I cannot simply lift my hands and say, but they weren't the intended users. I am serving them, and to a large extent I'm even using them deliberately to provide them certain types of advertising, et cetera, that I know is targeted to a younger audience. So then I need to say, actually I am serving this kind of younger audience, so I will have to provide them with the kinds of protections that we as a society have generally said they should be entitled to.
If the intended use is something, there Is a certain unintended use that is possible, and that certain unintended use has potentially significant impact, I need to make an effort to stop that from being possible. Put in place safeguards around, guardrails. We do guardrails on so many things, including just stairs that have a deep fall on the side of them.
So it's part of the bigger kind of sense of, in software, in digital, we need to be taking the same kinds of responsibilities for what we are doing. We can't say, but it's all just numbers, and we can always put out a patch later. Well, we are now applying these technologies to things that have significant, immediate, potentially, impacts on people's lives. If you role out the patch next week, you still have a certain number of people who've actually had direct significant impacts on them.
KIMBERLY NEVALA: So it sounds like it's really not enough anymore to say, that's not what I intended. That's not good enough. We also have to really prove and think that we've thought mindfully through what might have happened that we didn't intend, right?
Either way there's accountability. Now, it can get pretty complicated, right? The breadth of issues and things that we talk about, right, it's fairness, bias, privacy, self-determination, basic system safety and resilience, and that's just to name a few.
So maybe we come back to the importance of language in a minute as well. But is there a way we can help simplify this picture and boil it down to a couple common core causes, so as organizations and teams are thinking about this, you can kind of say, here's the top X things where maybe errors or these issues can arise, where here are some fundamental questions that you can use to just inform better decisions about where and how you're deploying AI? Data is the obvious one. We talk about that a lot, but in addition to data, are there other core elements to be considered?
ANSGAR KOENE: Well, the way that we've formulated also in the IEEE standard is that the core issues are really, do I understand who the stakeholders are who are going to be impacted? Do I really understand who they are and what their particular sensitivities are? You can deploy a certain piece of algorithm data-driven procedure for making good predictions about vulnerabilities in families in certain types of communities that are amenable to this kind of data access.
You cannot deploy that in others that have historical precedents of misuse of data about them. They're not going to want to have this. Understand the stakeholders that you're engaging with. The other part is, think about what this system is supposed to be doing. AI machine learning, it's basically a great tool for finding statistical patterns. Not all statistical patterns makes sense.
If I just use statistical patterns, I can probably find a correlation between the color of the leaves and maybe people's tendency to get sick. That doesn't mean that that's the kind of thing that I should be using to drive my predictions. Think about whether it makes sense, what the system is doing, and also make sure that it is actually doing what you intended to do because these AI systems, they're just finding a way to maximize the reward in your optimization function.
So they're getting the highest high score on effectively the game that it is playing relative to the data that you're giving it, but it can sometimes find a pathway to getting a high score on that game that isn't at all what you intended it to do. It found sort of a loophole in the way you described the problem, but if you then expose that system to an outside actual use case, it will have significant vulnerabilities where will break in a way. So understand your stakeholders.
Understand what your system is actually doing, and think about whether or not this is something that you would be comfortable having done to you. It's the very basic kind of idea of, if you wouldn't want it to be done to you, probably you shouldn't be doing it to somebody else either. And it's really around that.
It's the understanding what's going on. Don't blindly use a piece of technology just because it managed to do the thing according to your test case. It doesn't mean it's going to work well if you can't say, this makes sense. If you're going to get a high score on your emotion recognition technology, be that taken from faces, or from the newest one I read is head vibrations, if you're using any of those, there is no reason why this would make sense. So don't do it.
KIMBERLY NEVALA: Yeah, I think you commented on LinkedIn and just basically said, this is one of the recent spate of applications that do things for which there's no solid scientific evidence, right? Ethics sort of be damned. They might maybe edit that out. So when we think about that, that might be an area. What are the core tenets and objectives, then, of the current regulatory efforts? You've been involved in IEEE, developing standards there, and certainly there's the EU regulation. What do they aim to achieve, and what's their objective, and what do and don't they guard against?
ANSGAR KOENE: So what the standards activities, be it IEEE, ISO, or other standards activities aimed to do, is to give clarity as to what are good procedures to follow. If I do this, I will feel that, yeah, I have actually checked those questions, you know. I've made sure that I understood the use case, the right way. I made sure that I tested the data set against certain kinds of underlying problems with its distributions, et cetera, those kinds of things. The standards are really all about providing you with best practices around how to approach it so that you will know that you aren't tripping up against sort of obvious failures.
What the regulation are trying to do is safeguarding society and people, and so that's why we are seeing in the commission's regulation that it is focused on risk. And actually we are seeing the discussions in a lot of the other spaces be it the OECD, or be it in the US or in other places, are all sort of circling around this kind of question of, where are the risks that we need to identify and that we need to be dealing with? Because nobody wants to be suppressing innovation for the sake of suppressing it. It's all about making sure that we get services that are beneficial and aren't going to cause significant risks to people.
So a key question is, how do we identify what the risks are? And that is a challenge because this is a new piece of technology we don't have a long history of exploring, of using this, and I think in most countries we have this experience that usually a traffic sign or a traffic light gets put up after there's been an accident at an intersection because that accident has provided us with the evidence that this is a risky intersection. But what we're trying to do is sort of get ahead of that because we are seeing that this technology, it has the potential for being used at scale, and that's really where a lot of its value comes from, the scalability.
But that means that if there is a problem here that is going to have a negative impact, it's potentially going to have negative impact at scale. And so we don't want that to happen. We don't want to say, well, apparently we need to intervene here because tens of thousands of people have just had a negative impact on them. Instead we want to be able to catch that, but it's the, how can we do foresight? How can we identify the potential problems?
Like we talked about earlier, if we're talking about intended use, we also need to try to think about what are the potential misuses of this, potential unintended uses, and there's always going to be some that get away from us. But of course, we're just trying to minimize that space. Nobody expects the world to be completely safe. We just want to avoid dangers as much as we can, and that's at the core of this risk identification.
And then if we've identified this is a potentially risky one, it's not a, you can't do it. It's a, well, in that case, you need to make those extra steps to make sure that you are guarding against the and consequences. There are still a lot of open questions on the details of how to do this. We were having a conversation earlier today as part of the CEN-CENELEC standards activities.
The commission has indicated that they will be requesting some harmonized standards in this space to be ready before the regulation comes into effect. So that industry has these best practice guidances that they can follow to say, we're not sort of doing our best, and then end up not being able to be compliant, but rather we actually have clarity. If I follow these steps, I'm probably going to be compliant as long as I do it properly, do it to the best of our abilities.
KIMBERLY NEVALA: Yeah, there's an interesting tension, I think, there between, as you said, that reactive, we're going to put something in place because harm has already occurred, and then trying to look proactively. So policymakers are racing to regulate AI at the same time as we are racing to develop core capabilities, and then organizations are racing to adopt them. What do you think is going to most trip all of those actors up over the next couple of years?
ANSGAR KOENE: Well, one of the things that we are seeing is, first of all, that in the regulatory space we are seeing two races happening at the same time. We are seeing the race to build comprehensive and well-founded pieces of legislation that will deal with this technology at a kind of fundamental scale, but that is moving slowly because it needs all of the input from the expert committees. It needs to go through lots of rounds of evaluations, impact assessments. Is this going to have too much of a surprising impact on the ability to innovate, versus the benefits that you're getting, et cetera?
So therefore we are seeing in parallel another path happening, which is that some technology got deployed, people were angry about it, we need to do something to stop it, and that's, for instance, what we're seeing with a lot of the face recognition legislation that's happening, to block the use of that. It's because it is being deployed rapidly without a lot of thinking about these, what are the sensitivities of the stakeholders? And then those communities have stood up and en masse said, we are not happy with the way this is being used, and therefore there needs to be a response from the legislature.
So we're seeing two things happening there at the same time. What is the big event going to be that's going to trigger something? Obviously that's difficult to predict. I suspect it might actually come from a corner that seems innocuous to begin with.
It seems similar to what we've seen with the internet and social media, social media is just a place for people to talk about their daily lives and share things that they were interested in. How could that ever have caused big issues? And now it is the central point that we are talking about when it comes to, does the internet need more regulation?
Because it has accumulated that other use that, instead of it being us communicating about, hey, did you see that nice picture of my cat? It's, did you see this story in the news? Or I heard somebody say this. It's become that news flash rumor mill where facts and fiction get confused. So it might be something like that, maybe.
It's not going to be the autonomous vehicle which apparently is still going to take quite a couple of years until they're going to be deployed. It's going to be the Alexa, the home device that we use for accessing certain pieces of information and playing music because maybe a certain functionality got added to it, and then it is starting to have different kinds of impacts. We're seeing, for instance, a lot of children engaging with these voice technologies, and parents already starting to have certain concerns as they say, I only want them to use it a couple of hours a day, or only when I'm in the room, because I don't know where they're going with this, and concerns about how the way the devices are interacting with children, how that might change the kinds of expectations that children have, also of how other service delivery people are supposed to be interacting with them. Those kinds of things might potentially be something that we don't expect to be the risk application, but we will certainly see something happening that will potentially change the way in which we want to approach this.
KIMBERLY NEVALA: Yeah, it's interesting to think about how some of those behaviors or expectations we can cultivate in the digital world will boomerang back into how we engage with each other, and not just systems and organizations, but human to human in the real world, in ways that may be very good or maybe less good. So you spoke earlier about some of the challenges for us to really engage citizens and individuals in this conversation, but what advice would you give the average citizen or tell somebody as an individual that one important thing we really need to pay attention to as this moves forward?
ANSGAR KOENE: I think what you want to ask is that same question, does it really make sense? If I'm going to use this piece of technology, is it reasonable to expect this technology to really perform that function that it's being advertised as doing, or is it snake oil? That is basically the kind of question to ask, but it's a difficult question if you are not somebody who is in this technology space who knows what it is, that it is reasonable to expect for it to be able to do. So I guess the first thing to instill in people is this is not magic. If it were impossible for a human to do, if that human were to have access to tons of data, et cetera, if it is fundamentally impossible to conceive of a human being able to do this then probably you should be critical about this claim that this technology is doing.
KIMBERLY NEVALA: Now, that's an interesting perspective, and one I'm not sure I've heard before. So thank you, Ansgar. This was an insightful look into, I think, both the importance and intrinsic complexities of regulating what is a very fast evolving space and the challenge that's facing policymakers, organizations who are both creating and adopting this technology, and us as citizens. So a lot to think about, thanks again for joining us.
ANSGAR KOENE: My pleasure.
KIMBERLY NEVALA: All right, so that's a wrap for season one of Pondering AI. It's been an amazing journey of discovery on many fronts, and we are looking forward to season two kicking off soon. Now, in the meantime, if you'd like to expand your understanding of what AI is and isn't, learn more about how good AI systems can go wrong and why they don't have to, or explore why inclusion pays dividends and how the vision of AI for all is being achieved, we have an episode for you. So if you've missed any of our stellar guests, or would like to revisit an old favorite, like Ansgar, now's the time. Cheers.
[MUSIC PLAYING]
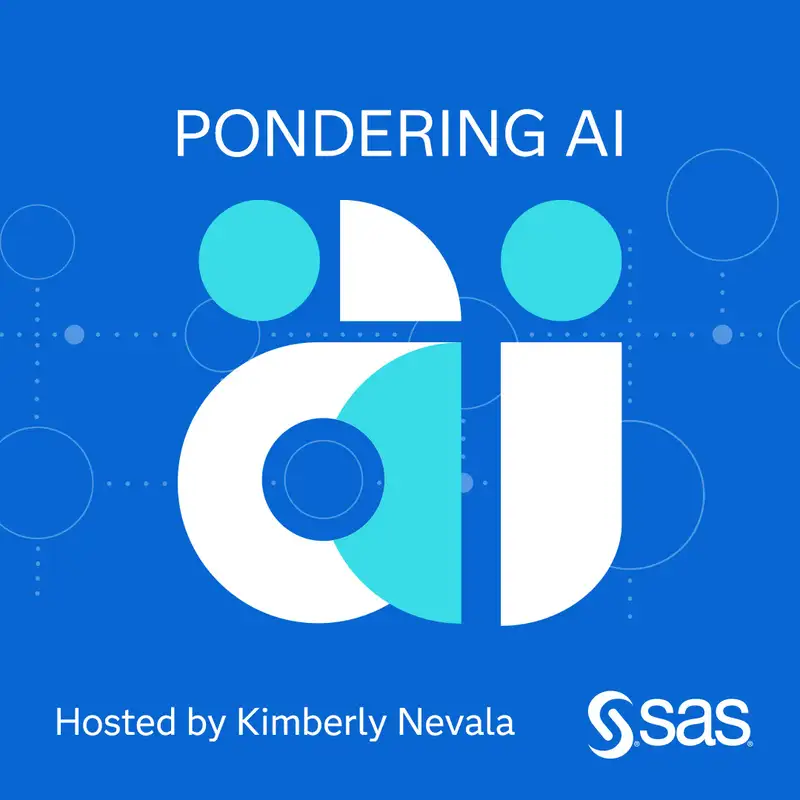