An Outlook on AI Ethics with Beena Ammanath
KIMBERLY NEVALA: Welcome back, or to, Pondering AI. My name is Kimberly Nevala, I'm a strategic advisor at SAS, and your host this season as we contemplate the imperative for responsible AI. Each episode we'll be joined by an expert to explore a different aspect of the ongoing quest to ensure artificial intelligence is deployed safely, fairly, and justly, now and in the future.
Today, we welcome Beena Ammanath. Beena is the Executive Director of the Global Deloitte AI Institute, and leads Deloitte's Trustworthy AI practice. She is a seasoned executive, she's got global experience leading AI and digital transformation programs, and has also served as a board member and advisor for numerous tech startups. Last, but certainly not least, Beena, is also the founder of the nonprofit Humans For AI. Thank you for joining us today, Beena.
BEENA AMMANATH: Kim, thank you so much for having me on your show.
KIMBERLY NEVALA: Yes, absolutely. You have had a really expansive career. What have been the most important or influential inflection points for you en route to your current position?
BEENA AMMANATH: Yeah, no that's a great question. And I get asked this question a lot of, how did you plan for your career? How do you get to where you are? And I don't think I ever planned for my career per se, right? The way it evolved, I've always been anchored in data. Right out of college, I was a SQL Developer for SQL Server, and it was a bad time, and it was more transaction databases.
And then came the era of business intelligence and data warehousing, and there was a big shift, at that point, from a data perspective. And I got very actively involved in that. I set up a BI team, the first BI team, at E*TRADE. And I saw some of the challenges, both from a cultural perspective, and also from a technology use perspective.
I bring that up, because now that same anchored on data, but we've moved into the era of machine learning and AI, when big data became real. Right? And we see the same questions coming out about, how will this technology be used? Will it displace work? Will it replace transactional systems? So having grown through this data journey, I've seen those different inflection points, and I think I was very fortunate that I chose data as an anchor to base my career on.
The other thing that I'll add in is also the fact that, even though I have anchored in data throughout my career, I have explored different domains and different industries. And it was more of the need to understand, the curiosity to understand, what's happening in the separate industries. So if you have seen my career path, you'll see I've worked in the financial services at banks and trading, and industrial and manufacturing, and field services, hardware technology. What was the curiosity to learn more about another industry, but anchored in data is what led me on this career journey, which has been a mosaic of experiences. Right?
KIMBERLY NEVALA: So did your thirst for diverse experiences and knowledge also play a role in the founding of Humans For AI?
BEENA AMMANATH: You know, I've always been an advocate for getting more women into tech, women into STEM. As I grew in my career, I started noticing there were lesser and lesser women at the table. And once AI started becoming real, data science and machine learning teams were being set up-- I have personally set up a number of data science teams-- and I realized that there was a very homogeneous group. And it was also during the phase of, everybody was trying to hire PhDs in machine learning and AI.
And what I realized is that, as you're building AI products or solutions, it's not just a data scientist skillset that you need. You need software engineers who understand AI. You still need product managers, you still need QA, you still need designers, right? You need all these skillsets. So it came from this frustration of not seeing enough diversity in AI, and my also my worry that unlike other technologies, I feel AI is one where you truly need diversity of thought as part of the AI process.
And since there was a lack of diversity, the intent of Humans For AI is to really drive basic AI fluency, with a view to getting more product managers, more QA, more designers. Surround the homogeneous group of data scientists with diversity of thought. And diversity of thought, for me, includes gender, race, geographical background, cultural background, educational background. The more diversity we bring into building AI, the better and the more robust those AI products are going to be. So that's the intent for Humans For AI, to drive more AI literacy, to enable more diversity and inclusion in AI.
KIMBERLY NEVALA: Yeah, I think that's so important. And we had the opportunity to speak with Tess Posner who is the CEO of AI For All, and she talked about her work in that same area. And we talk a lot about the technology ecosystem for AI. But you're also underscoring the importance of attention to and deliberate cultivation of the human ecosystem around AI, and that network of voices and people. And the importance of creativity and imagination.
Has that been an aspect of AI that's really changed? Or that conversation's changed over the last, maybe, three to five years? And are there other areas that are also really coming to the fore?
BEENA AMMANATH: Yeah, it definitely has changed. Ethics is a broad terminology, and I definitely think, even compared to five years ago, right, ethics was more of an afterthought. And we were beginning to hear some rumbles around, what are the ethical implications of this technology? But it was more focused on clickbait headlines, right? How do you make this into a big deal so that you can drive that fear, which drives to more clicks?
So it was more of that stage. But what I'm seeing that has evolved is ethics and the different aspects of ethics is coming early in the conversation, as companies are beginning to look at using AI broadly across their organization, which makes me very, very happy. And I'm very optimistic about it.
It also drives the need for operationalising AI ethics. It's one of those complicated and nuanced subjects, because ethics is not going to mean the same thing for different companies, or even within a company for different use cases. So being able to think about the nuances is super important. And I do think that those conversations that are happening, which is driving more awareness, which will then drive to best practices and policies and regulations, which will really help us build those guardrails to help innovate faster with AI.
So maybe I can give you an example, or a view of how I think about ethics. Just to level set.
KIMBERLY NEVALA: Yeah, I think that would be helpful.
BEENA AMMANATH: So having-- I've totally dated myself-- so having lived through this time, right? When I studied AI, it was very much in theory. This was in the late '80s, early '90s. And when I was studying, there was no easy access to massive compute. Right? It was not generally available. And there was not massive amounts of data available. So what we were doing is more of theory.
And for me to see it becoming real now, I can clearly see three streams. The first stream is really the core technology. Whether it's the next wave of quantum computer, the next wave of neural network, neuromorphic computing, all this core research on developing the technology is happening. And that's the first stream, which is primarily happening in research groups in academia.
Then there is a second stream, which is really about the applications of these technology. While the technology is still being developed, we're applying it in the real world for solving real world problems, because that technology is so powerful. We have not fully thought through it. So this one is accelerating as well, the second stream.
And then there's a third one, which is the impacts of that technology beyond value creation. In the second stream, we primarily focus on what value can AI bring to us? How can my data drive more insights to drive more business value, right? The third stream is everything beyond the business value. What are the health implications of using this technology, and what are the risks associated with using this technology from a brand, from a legal perspective? What policies exist in the space? So everything that is beyond value creation, that is a third stream, which is also still evolving. Because, as you can see, everything is still evolving. So think about trying to put in speeding limits while the car engine is still being developed, and we are also driving the car forward slowly.
Right? So, for me, I think we live at this great point where we're seeing all of these big forces happening, and it's an opportunity for us to shape and influence it, and tie it back to the diversity challenge, right? We've always had a problem around not having enough women in tech. But here, we have an opportunity to get more diversity into technology, because these new roles are getting created. So can we proactively train more diverse humans to take on these new jobs that AI is creating?
KIMBERLY NEVALA: Yeah, I think that's so important. And there's just this ongoing thread in the conversations we've had to date, and I expect to have in the future, about diversity and inclusion being really fundamental to how we do this. So we obviously come from, maybe it's not obvious, from technology backgrounds. And certainly, the tech industry in general has been-- and this seems, again in the clickbait headlines-- accused of techno-solutionism. Even in the context of, AI is going to solve all of our problems and we should basically apply AI solutions to everything we can.
I wonder, are we in danger of being overreliant on technology to inform us, identify or correct for some of these issues? What's the right balance there? What's your perspective and experience?
BEENA AMMANATH: Yeah, so my experience has been that, especially as a technology company, you are kind of providing the foundation which will be leveraged across different industries. And you pretty much don't have that control beyond it. So how do you put that guardrails within the technology, or a disclaimer, so to speak, so that you are providing the education on all the different aspects, maybe, you tested this technology on. So that if there is a new use case that's going to use the same technology, they know it hasn't been fully vetted out for this.
I'll give you an example, Kim, to make it a little bit more real, right? So this one was working at a jet engine manufacturer, and we were looking at jet engine data, so that we could proactively predict when an engine might fail. And then proactively send a service engineer to fix it so that there is no unplanned downtime and flight delays. Very simple value prop.
But as we started doing data discovery, we started noticing that you could actually see how the pilot was flying the plane. Was he or she hitting something too hard which was causing engine corrosion, leading to more frequent engine failure? So we could actually see pilot behavior, which was not the original intent. And the company wanted to use that to actually impact that pilot's performance evaluation, because he or she was not following the training, right?
So when you go through this data discovery phase, you discover things which may not be what you were actually looking for. And to wrap this one up, we went to FAA, the Flight and Aviation Authority to see whether there were any guidelines, and obviously, there was none. So what ended up was, we didn't share that data, because looking at the data, that was not the original intent. But what the airline ended up doing was providing better training. They actually made it more gamified, so that they improved their training to make this better as opposed to.
So you respect the privacy. You respect not sharing that level of data. But at the same time, there was real world impact, which hopefully drove better behavior. So thinking through it as the team goes through data discovery, as they find these nuances. You know, at this point, as technologists, I think it's on all of us to think through the ways this could go wrong, and proactively ask those questions to make sure that you are putting in those guardrails.
KIMBERLY NEVALA: Yeah. I'm laughing to myself here, because as you were talking, and what was going through my head-- and I both hope and don't hope anyone from my insurance company is listening-- and I was thinking, this is why I don't do that sort of good driver widget in my car. Because I'm an excellent driver, but they may not always agree. So this idea that, yes, you're going to save me some money, but you may also make some decisions or look at things in a way that I think just might be a little bit of an overreach. Or very, very invasive.
So variation on that theme that I think is interesting, and going back to something you said before, which is, we have a lot of focus, when we're having those discussions, on bias and fairness, which are absolutely critical. And should be front and center, but they don't always apply. But there are other ways in which these solutions could, it's not even necessarily go evilly awry, but result in outcomes you didn't intend, or undermine human agency.
And there's a very simple example out there where we're using machine learning to try to optimize for inventory on a shelf. So it might be at a grocery store where things that haven't been selling, how long do you keep them? Do you discount them? Do you throw it out? And how do we sort of optimize that whole process?
And seems like a great system, except if the store managers incentives are aligned with just selling based on actual revenue, regardless of how heavily discounted. And you're giving them a suggestion that says, you need to throw stuff out, which, by the way, is going to contradict what we're trying to incent you on, or your human objective. We've now got an interesting problem.
So this need to think about alignment between the system objectives and human incentives. Or to align how things work in the real world with how we are modeling that in the digital world. Are there examples of things like that, or other considerations that we need to be focusing on in addition to these probably more highly-publicized aspects?
BEENA AMMANATH: Yes, absolutely. I think that that's a great example, right? And it's forcing all of us to think through how our technology has a broader impact. So going back to your insurance example, Kim, what struck me was that you are empowered, you are educated enough to realize that data might be misused. But there are millions of people in the world who use the same technology, but they just don't have that basic technology literacy to even ask that question, right?
And it's always fascinating for me to see how much of a divide technology causes sometimes. And it's just basic lack of literacy. Like my mom, she would never ask that question. It won't even cross her mind, right? Or, for that matter, my teenagers. They would happily share all the data that they're asked for, because then their music system is recommending the right songs for them, right? So irrespective of whether it's a music company or it is an insurance company, they have to think through all of, how do you actually show the value and proactively put in the guardrails to say, hey, we're not going to share your data beyond this. This is the only purpose that we will be using this data for.
So one of the principles that I have in there is privacy. And the question comes up, why do you have privacy AI ethics principle? The privacy just for data and its primary usage in the past, that has evolved. Now we use that same data to train models, and as you go through the data discovery, to my prior example, you're going to find correlations that you're not anticipating. So are you going to share that with your customer? That we are going to use, not only for this ranking, but we're going to use this data to build out your digital twin and use it for marketing.
So here, I think one of the key aspects we need to think about from every dimension that we might have used in the past, from a data perspective, is its privacy or security or robustness. You have to apply the AI lens. Or any emerging technology that's coming up, right? Apply that AI lens to see, how is AI now changing the dimensions of privacy? And how do we re-evaluate our processes around it? How do we re-evaluate our communication around it? And making sure that you bring all your users up to speed on the implication, so that they can actually have a valid voice, rather than being caught up in headlines.
KIMBERLY NEVALA: Yeah, and there's been this ongoing conversation about, as you really illustrated so well here, that in a lot of cases, we haven't thought about how we might put the data and information to use at the time we're asking people to gather that information. And so there's this very interesting loop or process we have to think about where, justifiably, just asking for these broad, we're going to use your data for anything we can think of, sort of statements, I think are going to go the way of the dinosaur, I hope. But there is this interesting conundrum.
And the other thing that I've personally been pondering is in the past, the data that we're talking about securing or keeping private is very discrete. It might be my shopping history and things like that. But with AI, the data that we have to think about keeping private is the data and the information that's derived from that. And that information is equally, if not more, interesting and telling, I think, from a personal perspective or even from a societal perspective. Today, I'm not sure that we think about not just the explicit data pieces, but the insights that we're deriving as also requiring privacy and security.
BEENA AMMANATH: Yeah, you're so right, Kim. And I'm smiling, because I look back in the early 2000s we were using data-- business intelligence and data warehouse-- we were using data, discrete data, to do personalized marketing. We used data not only that potential needs or prospects gave on our own website, but we were able to buy external data sets, merge it together, and then build a better customer profile to do more personalized marketing. This was happening 20, 21 years ago. Right?
But what that has done now, that same process has now scaled to an extent that you can get all kinds of variety of data. That was more of the era of where you got very structured data sets, right, that you could merge. Now you can get all kinds of data about an individual, and how do you make sure that your clients, your customers, are fully aware? They might be just giving your name and address, but you're merging it with all these external data sets that is actually helping build a profile.
So how do you actually educate your customers that this is all the ways that could actually drive the data usage? I think the companies of the future, the companies that will succeed and continue to earn trust of their customers will be the ones who are more transparent. Who educate their customers and who bring the customers along their journey, and not do things in the background without fully informing the customer, right? Do you agree that that's where we're headed?
KIMBERLY NEVALA: I do. I think people are really, oddly, willing to share their information and have it used, if they're told that it's being used, and that you're operating in a transparent way. And I think that's even true if they said, we're not exactly sure exactly how we're going to use this, but we think it could be done in these ways and for this purpose. And so establishing that quid pro quo in a way that is good for your consumer or your user or those you serve in addition to you.
And I think that younger generations, while they are eye openingly-- or eye wateringly-- willing to have all their information out there, right. So sometimes I do wonder, to date myself, I learned to program in Fortran. So if this idea that all the information about us is used to sometimes wean people out of things.
So we might look at, hey, you know your social life and the things that you said when you were young and maybe just less aware and/or more uninhibited will come to haunt you in the future when you look for a job. Maybe, in the future, we're going to look for people who don't have those things and wonder if they're actually human. You know? So there's the perspective of the way that we grew up and think about some of this information might be different.
There's that old adage that change is the only constant. And I think AI systems really model that adage, no pun intended, or maybe pun intended. So how can organizations manage that, and confront that sort of inherent uncertainty?
And I think in 2018, you wrote this article, and I loved the premise of it. And it was titled, Educating Against Good Intention. How has awareness of the need progressed, and is our ability to execute against that keeping up?
BEENA AMMANATH: Yes. That's a great question, because I think we went from murmurs about ethics, five, six years ago, to a lot of noise in this space. Because the headlines still continue to get created, and then there is a lot of additional voices that are adding to the noise without really focusing on the solution, right? I think the way to sort through this is through the human ecosystem that you're mentioning, bringing in that diversity of thought, because AI ethics is one of those topics where it's not just about technology. It's also about the legal implications. It's also about the human aspect, the health implications, of using these technologies.
So what we are seeing is really companies being proactively thinking about it. I mean, I have seen two different scenarios. One is companies that are very early in their journey, they are thinking about, what are the ethical implications that I should be thinking about? And what guardrails do I put in place today so that my team can run faster and innovate faster? Now they have the luxury, because they are early in the journey.
The companies that are later in their journey and have been dabbling with AI, or have AI solutions in their production systems, are now really taking a step back and saying, how do we proactively think about ethical implications of the technology that we are using? Both technology that might have been built in-house, but technology that might have been brought. Right?
So we will see a lot of movement in terms of defining, what are the ethical principles for my organization? What are the ethical principles for this project that we are working on? And how do we track it? How do we train our people?
So Kim, one aspect that I have seen evolving is really those nuanced discussions on, how do you make it real? And from my experience, what has worked is it's beyond technology and just putting guardrails. You have to train your entire workforce on what this technology ethics means, so that the accountant in your finance department, if they're using and AI software, can still raise a flag if something is appearing to be a risk, or is raising an ethical concern.
So training and driving of cultural change. Because as a technologist, I know we get really focused on the value creation that technology can drive. But early on, the conversation is now around, what are all the ways this could go wrong, and how do I prevent it with the work that I'm doing? So I kind of push our engineering teams to really think through, OK, great. These are all the positive things. This is how it's going to create business value. Now, let's take the time and think about, what are the ways this could go wrong?
The project team is the best team to define that, right? Because they know what they're building. So how do they actually proactively think, what are the ways this could go wrong, and how do we prevent, how do we mitigate, and how do we make a decision around it, right? How do we make sure that the systems we build are trustworthy, and that we put in all the guardrails ahead of time? So definitely a lot of movement.
I still think it's still early days, because there is no one defined playbook. The regulators and policymakers are still catching up on it. So and then there is the academia and research group that's continuously putting out new research in this space. So how do you connect it across all these dimensions and make it real in the enterprise? Those conversations are happening. And I think we will get through this. I'm very hopeful we will get through this before we talk next, Kim.
KIMBERLY NEVALA: Well, that's good. I'm excited that we have the opportunity to talk again. So you mentioned organizations putting AI principles in place. And certainly, there are a lot of frameworks out there in academia and public partnerships, and profit and nonprofit organizations. Is that the right first step for organizations?
And then, in terms of those guardrails you were talking about, are there actual practices or processes that people can start to put in place today to really translate that from principle to practice? Because I think it's fairly easy, to some extent, to agree to the principles. But how do we actually create the space and the safety, but also the rigor, to stop and ask those questions, or to do those checks along the way?
BEENA AMMANATH: Yeah, no, that's a great question. And speaking of frameworks, I have put out a framework as well called Trustworthy AI Framework. And if you think about it, it is very broad and all-encompassing. The challenge that I've seen in the past is really, when we speak about ethics, it tends to go very quickly and very rapidly down the path of fairness and bias. Which makes a lot of sense, and I think that is a real ethical issue we need to deal with. But it may not be relevant for all use cases, right?
Having worked in a manufacturing company, for example, right? If I'm trying to automate, predict when a machine might fail on the factory floor, fairness and bias don't play as much significance-- in fact, there is no significance there-- as much as the robustness and the reliability, the security of the algorithm. So I think frameworks are a good starting point to think through, what is important? Which part of this is important for how I am using this technology within my company?
So that's what helps to define the principles. You agree upon it. So for a factory floor, it might be that they don't take fairness and bias, it's not important for predicting factory floor machine failure. But it is super important if you are a retailer. So defining those principles, having that conversation with key stakeholders, is that first step.
And once you agree on the principles, the next step is now, how do you operationalize it, as you rightfully asked? And we look at it across the three dimensions of technology, process, and people. And people is really empowering, the stakeholders have agreed on the principle, but how do you communicate it to the entire workforce? Because even if they're not building AI solutions, they're probably using it.
So how do you create that space where you can raise a concern if you think a particular software that you're using is not behaving in an ethical manner? How do you put controls in place so that there is process checks that can be added? And Kim, I speak about it in general, because it has to be at that level, because you have to think about it from an organization, within the organization, what processes are there? That need to be changed where you are proactively bringing up the ethical conversation.
At the beginning of the project, it might be a question, what are all the ways this could go wrong? Right? But as you roll it out and scale it out, you still need to continuously keep evolving and see, have the ethical implications changed? Is the model still behaving?
The other aspect is the regulations, because the regulations are still evolving, right? So you might have a model in production, which you might now have to re-evaluate from the new regulations that have come up. If you are working with a vendor, and this could be happening anywhere in the organization, how do you make sure the software that you're buying is aligned on the same ethical principles? And making sure that you check for those.
So that is the people and process part. Having the controls across all throughout all your processes where AI might be used. And the technology is really where I'm seeing the most traction in the market in terms of solving for it. Because there are a number of startups, but there are also companies who have moved ahead in being able to assess ethical implications. Once you define the principles, how do you assess the existing AI to make sure it aligns from a technology perspective? And then, provide the code that you can modify or inject into your existing solution to make it, to align with the principles?
I see a lot of traction happening on the technology side. I see movement on the people and the learning and development side. I think the process side, because it is so nuanced and specific to an organization, is still very individual company basis. There's also, you're seeing newer roles evolving, right? Whether it is hiring AI ethicists, or getting a Chief AI Ethics Officer, or having AI Ethics Advisory Teams. There are different models on who will own this going forward. I think that's evolving as well.
KIMBERLY NEVALA: Yeah. We often say that you need to measure what matters, and what matters is what you measure. And you've recently been talking about the need to, then, think about incorporating things like sustainability and ethics as core business metrics. As things that how we value companies. Why is that important, and how does that integrate with the evolution of AI in your mind?
BEENA AMMANATH: So, a couple of things. One is, I think for a very long time, we've measured companies based on pure numbers and metrics and balance sheets. But to your point, the younger generation that's coming up is digital native, right? My kids are fully aware of all the ways they can use technology, and they may not be as worried about sharing their data, but they worry about the planet. They worry about climate change. They worry about sustainability.
So our user demographics are changing. And I think for companies to thrive, they have to think about all these dimensions that's important for this changing user base. And we are going to see whether, it's ethics balance sheets or sustainability balance sheets, those metrics are going to evolve, and companies are going to get tracked and measured on that. Otherwise, you're going to see user base drop.
You already started seeing some examples of it, but I think the next generation of users is going to be more savvy. At least that's my hope. And they will be looking for these additional dimensions which are going to enable them to trust a brand. Brand savviness, brand association, for some of these softer dimensions which move beyond just profitability is definitely something that we'll see happening, and we're going to see companies evolve to be able to accommodate that.
KIMBERLY NEVALA: That'll be an interesting evolution to watch. It's an important one, and just such a new thought, in a lot of ways. Although I think we've had things like ESG initiatives. I don't know that they've transcended to balance sheets and all of those things.
So as we wrap up here, there's so many things that I've got going through my mind I could be asking and pursuing with you. What are you most excited about as we look to the immediate future in AI? Either broadly or in relation to the ethical integration with AI?
BEENA AMMANATH: I am most excited, from an AI perspective, to really solve for ethics and operationalize it in the real world, and to get more diversity and inclusion in the process of AI. Right? Because I think for AI to reach its true potential, true full potential, it needs to work for all humans. Not just the tech-savvy, not just the tech-literate, but for all humans to be empowered to ask those questions.
I'm also excited about one other thing. Not directly tied to AI, but with AI and ethics, we are playing catch up. But what does ethics mean for all these new technologies that's coming at this? What does ethics mean for quantum? What does ethics mean for VR and AR? What does that mean for blockchain?
And the reason I bring it up is, as virtual reality or augmented reality become more real in the world, there is an opportunity for us to stop playing catch up and proactively think about it, and say, is fairness and bias going to be relevant for virtual reality? When you are projecting an image, is it going to appear differently based on the color of your skin, for example? Right? So I'm very excited for us to also think about the ethical implications of emerging technologies, and for a change, be ahead of the game.
KIMBERLY NEVALA: We might have just found the topic of our next conversation. So this is a win-win all around. Beena, thank you so much for sharing your diverse perspectives on the state of play in AI and ethics today. I've really enjoyed this chat.
BEENA AMMANATH: Kim, thank you so much for having me. I know we could have a long conversation around this. I'm happy we started this.
KIMBERLY NEVALA: Absolutely. Thanks again. Next episode, we will be learning from Teemu Roos. Teemu is an accomplished academic, researcher, and applied AI practitioner whose team at the University of Helsinki develops new machine learning methods for areas from cancer epidemiology to digital humanities. His free Elements of AI course was rated as the world's best computer science MOOC-- that's Massive Open Online Course-- on Class Central. Make sure you don't miss him by subscribing now to Pondering AI.
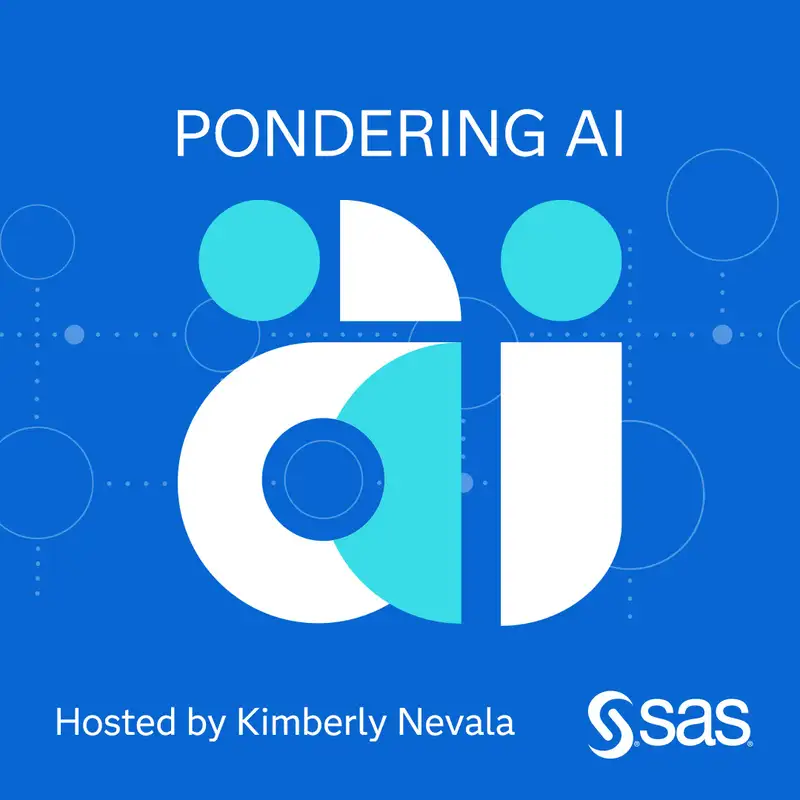