Risk vs. Rights in AI with Dorothea Baur
KIMBERLY NEVALA: Welcome to Pondering AI. My name is Kimberly Nevala and I'm a strategic advisor at SAS. In this season, we're joined by a diverse group of thinkers and doers to explore how we can create meaningful human experiences and make mindful decisions in the age of AI.
In this episode, we welcome Dorothea Baur. Dorothea is an independent consultant and thought leader on ethics, responsibility, and sustainability in tech and finance. Amongst other things, Dorothea will help explain why pursuing an ethical practice isn't just an esoteric endeavor and if a risk-based approach to ethics is enough. Welcome, Dorothea.
DOROTHEA BAUR: Welcome, Kimberly.
KIMBERLY NEVALA: So let's start by getting a sketch of your career trajectory to date. And correct me if I'm wrong, but I believe you may have started out in academia.
DOROTHEA BAUR: I did. I did. And I stayed there for quite a long time, even beyond my PhD.
So I did a PhD in business ethics, which is pretty interdisciplinary because it is the application of ethics to the business context. And I stayed in academia for a while longer as a postdoctoral researcher and lecturer at various universities. But then I found I wanted to have more impact in the real world. I felt a bit caught in the ivory tower, which I'm not blaming anyone but myself for that.
So I left and I founded my own consulting company and have gradually moved from business ethics, or expanded the horizon from business ethics, to also include tech ethics. Which is again, in line with my very interdisciplinary, or you could even call it transdisciplinary, perspective on things over the past five or six years I would say.
KIMBERLY NEVALA: So this is great. Because certainly, everybody in tech and in AI in particular - but also in the context of crypto, and blockchain, et cetera, et cetera - is talking about ethics. But there are relatively few trained ethicists like yourself in practice.
And often, when this topic comes up, it does seem, as I said previously, esoteric. Or people view it as a tool for judgment and primarily for finding fault. What do you think is the most common misunderstanding or poor framing of ethics as a topic or a discipline today?
DOROTHEA BAUR: Well, it's often a misconception that ethicists are here to name and shame, as you said, with a raised moral index finger and to make people feel bad. And that's the last thing I want to do. My job is to provide orientation in your thoughts. And so one misconception is ethics will always make you feel bad.
The other misconception that's also quite common is like, wow. You can't really talk about ethics. It's so individual. It's whatever pleases you. And that's called relativism. And I argue against that. There are some established universal values, especially as laid down in human rights conventions, et cetera. Even though we do differ across cultures and also between individuals it's not totally random, haphazard. Whatever your preference is can be compared, in ethics, could be compared to your preference for a red or a blue sweater. That's not what ethics is about. So that's the second misconception.
And then the third misconception, which however obviously does not apply to those who are willing to talk about ethics in the context of tech, or business, or blockchain. But the third misconception is you cannot apply ethics to things like technology. These are just things and they do not have values. But here I would say, everything that has been created by humans is the responsibility of humans. What we set in motion is part of our ethical responsibility. So if you are even willing to talk to me about tech ethics, that means you're not seeing technology as something that follows natural laws like earthquakes or gravity where we do not have any impact or influence. And neither do we have responsibility for earthquakes for example.
KIMBERLY NEVALA: You mentioned your background in business ethics. And I'm interested: when we start to expand or project that into tech ethics if those two things are really the same. So where do those practices of business ethics and tech ethics, if you will, converge? And where do they diverge? You alluded to this a little bit in your opening statement.
DOROTHEA BAUR: Well, in many ways, they do overlap if you think about tech as an industry that's following the logic of profit. Not necessarily maximization, but profit, making profit generation. So you do have business issues that are just applied to different industries. And the tech industry is one among them.
So if you look at technology as a product or a service that's being - there's a supply, and demand, and that's being created and sold by companies, and used by consumers, or users as we say - you have a lot of business ethical questions in tech that are not necessarily new. But you do also have an entirely other branch of tech ethics where you say, what are the specific ethical challenges that tech, like for example artificial intelligence, entails, and that have never been presented to us before?
And that's where the whole AI ethics debate has to deal with issues of bias, for example. And where there is a really interesting and challenging convergence of, for example, statistical issues and ethical issues. And where it's really hard to kind of - even for me as an ethicist - I do have my limits. I don't know everything about statistics.
So these are entirely new inter-disciplines in the field of tech ethics. And these are distinctively new challenges. So that's where the two fields were. Business ethics is not sufficient anymore to answer tech ethical questions.
KIMBERLY NEVALA: And is there a good example of that mashup, if you will, between statistical perspectives and ethical perspectives or considerations?
DOROTHEA BAUR: Yeah. So for example, if you talk about fairness. Fairness, of course, is a value that we have when we watch a soccer game. We say, this is fair. This is not fair from the referee, and this yellow card, or red card, et cetera. But when it comes to AI ethics and you talk about algorithmic fairness, it is a statistical concept.
And then it's the question of where, for example, do you set the threshold for - I'm not a statistician, so please correct me if I'm using wrong terms - but where for example you set the threshold for the amount of false positives that you want to allow in the application of AI. So what is your limit of tolerance? And how do you measure fairness? Is it the amount of people who benefit from certain service? Or what is the accuracy rate?
And that's where you have on one hand mathematical measures, numbers. But before you get to these numbers, you have to make an ethical judgment. Which method of calculation do we want to apply? And that's really fascinating. These are entirely new issues, at least for me.
And I know philosophers, some philosophers or also some really smart theoretical computer scientists, and maybe many other people, but not me, they do have answers. Or they do have suggestions on how to resolve those. But these issues are becoming much more prominent now.
KIMBERLY NEVALA: And I wonder if some of the new - as you said - or emerging issues with AI as a technology and tech ethics, have to do with the scope and scale at which these systems are in fact operating. I think in the past, it might have been very simple to see - and we always talked about things like user experience, or customer experience, or stakeholder engagement.
And certainly, stakeholder engagement is a discussion we've had with many, many folks. You say this is so important, to have that multidisciplinary, and all these different folks in the room. In your work, why do you think that's so important and so difficult when we're dealing with AI?
DOROTHEA BAUR: Well, the first thing is that AI is not a tangible product. If you have stakeholder engagement on textile industry issues, we need the workers, we need the farmers, we need the customers, we need the salespeople, et cetera.
So when you talk about AI or tech in general, there is a new category of stakeholder that arises and that is hard to grasp. And that doesn't follow conventional stakeholder identification logic, namely the person upon whom AI is applied, which is not necessarily the user.
Let's say I go to social services and they use AI to predict my likelihood of, I don't know, fraud. Benefit fraud. So I mean, then in that moment, I'm a stakeholder. But if you follow the ideal of doing stakeholder engagement as you develop the product and ex-ante, not just ex-post, how do you feel treated by this AI application by social services? But ex-ante, how do you define the criteria by which you identify the stakeholders?
You will need all those upon whom AI could be used. But then what's important is to select these people according to, for example, sociodemographic characteristics. Because we know that especially in ethically sensitive contexts like social services where there are a lot of issues of potential discrimination, et cetera, the idea or the logic according to which you identify as stakeholders must look at categories of people who might be at higher risk of being discriminated because they represent minorities in a society.
So the whole concept of stakeholder engagement needs to evolve. And you need to look at how you identify your stakeholders from a different angle than just a simple, oh he's a worker, she's a salesperson, they are consumers perspective.
KIMBERLY NEVALA: So it likely follows then that you also can't necessarily assume that even if you design a system to scale, or you want to deploy it globally, that in fact you're going to be able to sort of design one system, if you will, or one algorithm to serve them all. Right? Because there are different cultural contexts, there's different sensitivities. We talk a lot about the need to address things like systemic bias here in the US that may or may not… and that's actually not the only ethical issue we need to be concerned about with AI systems. That may or may not be as big a concern in other areas.
So I'm interested in your thoughts on whether there is in fact a… If we are trying to design systems that scale, and scale being across regions, or globally. Or maybe it's just state to state here in the States: is there a global or a universal context we can use to ground these discussions of ethics or even issues, more targeted issues such as discrimination? Or does it just really depend?
What is universal, I suppose? Is there anything that's truly universal?
DOROTHEA BAUR: Well, and this of course, I mean, is a century old debate among philosophers. And I'm sure they're not presenting a new answer. I do have my preferences for certain philosophical strands.
And I would say human dignity is universal and they're inviolable human rights, et cetera. And there's a basic body of values mostly related to human rights that should be inviolable.
But what you meant, I think also-- what is interesting when you say tech is all about scope, and scale, and speed. And there is an unholy collision of the ideology behind the original actor, the hotspot of tech industry, Silicon Valley mentality with move fast and break things which asks for scalability and speeding up everything, and the-- and transparency to a certain extent uncontrollability of the impact of AI. Again which has to do with the fact that it is less tangible than other goods that are being produced.
And so when you scale something from Silicon Valley and you think, oh, we found that one AI application that can be applied universally to solve, let's say, an issue in health care, you need to be very careful that you don't make what is called the generalization error. That when you use AI that has been trained on data from a certain context and you apply it in a context where data is totally different, or the reality is totally different, you will have a really high risk of making mistakes, at it being inaccurate, and also at committing a lot of other kinds of discrimination.
So that means on the one hand tech is all about scaling, about democratization, as they say it oftentimes, making health care universally accessible by using AI as a diagnostic tool. So you could in theory dream about people who cannot afford seeing a doctor who are too far away from medical services, they could just rely on whatever AI application.
But on the other hand, it is really sensitive in terms of ethics. It is very sensitive because the context in which you apply often differ from the context on which the data was trained. And so that's one thing. But I don't know whether the universalization of values-- I think I would say the values are similar, but the challenges that they pose are different in different contexts.
As you say in the US, you have systemic bias, you have highly racialized justice, and other societies have other problems, other structural problems of justice. Maybe there, it's not racialized. Maybe it's more along the lines of gender, or a north-south divide in a country, or religious divide, or whatever. And so that's what we need to be careful when we, especially in Europe, talk from a European perspective, try to just apply whatever comes from the US in our societies. We don't have the same structural foundation on which our societies-- we don't have the same problems. We have other challenges.
KIMBERLY NEVALA: Yeah, and different sensitivities, different perceptions--
DOROTHEA BAUR: Exactly. Exactly.
KIMBERLY NEVALA: Different experiences.
DOROTHEA BAUR: Yes.
KIMBERLY NEVALA: So that really then underscores the point that there's no AI-enabled system that is perfectly ethical or that will ever be perfectly ethical because there's context.
DOROTHEA BAUR: Especially not if you apply to people, machine to machine AI implications. I think sometimes, I feel forced. I have to emphasize that there is AI that really does not raise a lot of ethical questions. But of course, my job is to talk about AI that raises ethical issues. But I don't want to say AI is always a terrible ethical challenge, whatever. We're talking about sensitive contexts about applying AI in an inter-human context, et cetera.
KIMBERLY NEVALA: So I read something that I believe you said a while back. And you said, back in the day, this conversation about what is the business case for AI ethics, or tech ethics? And I believe your statement was ‘I would have refused that discussion back when I was in academia. I just would not have engaged’. And you may have changed your thinking on that.
So interested in the basis, if that's true, if I've quoted you accurately. And what do you say to business leaders and organizations? Why is ethics not just interesting, not just something we should give a nod to, but really material for businesses today?
DOROTHEA BAUR: Yeah, I know I said that. I said that I can't believe that I am kind of backing up Milton Friedman, who says something like, the business of business is business. And I'm usually not in sync with him. But I mean, you cannot deny that business leaders want to see business logic. And you cannot force them or expect them to become social justice warriors. And you probably do not want them to become social justice warriors.
So you need to talk their language. And you need to tell them what the non-financial dimensions of their responsibility (are), what they mean for their business. And just as we have a discussion about the business case for sustainability, which is oftentimes straightforward of saving costs when you use less energy, well that's a simple example. Or social dimension, treating your employees well, will lower turnover will lower the changes among your staff.
KIMBERLY NEVALA: So just as AI and data strategies don’t start with AI or data, discussions of ethics should also start with meaningful business objectives – fiscal and otherwise.
DOROTHEA BAUR: Exactly. Exactly. So these are the low hanging fruit or the no brainers. You need to find such examples also for AI ethics. And so I think it's also stakeholder related.
On one hand, the most powerful stakeholders in the tech industry that will make businesses very much aware of the financial dimension of AI ethics are the employees. You can see the employees-- just today, I read something about Google firing someone who had criticized the plausibility of a claim they made about some technical innovation or AI related innovation.
And so if employees don't feel that their employers or their company is acting with integrity, and if they don't feel that they are employed by someone or by people who serve meaningful purpose, and if they refuse, for example, if they are not OK with working for a company that, for example, works with a Department of Defense or some states with a dubious Democratic track record, et cetera, they walk away.
And there is nothing more valuable in the tech industry than the employees. So it is in your very interest as a tech industry boss to ensure that you have a comprehensive notion and concept of AI ethics, and also make it part of your corporate culture. Because it will cost you dearly if you lose your really valuable and good people because you have a blind spot on these issues.
So I think employees, and then the other force for potential good, I'm setting that in quotes, are shareholders. We often thought about shareholders back in the Milton Friedman days. Oh, they only want to have profit maximization. And of course, there are those shareholders. But I would say the whole ESG discussion, like about environmental, social, and governance issues, of corporate strategy, is also increasingly including AI ethics questions.
We've already seen that with some shareholder, criticism towards Amazon for their facial recognition technology. And I think that's the ESG movement will embrace AI ethics issues very soon. And if-- tech business bosses could say they don't care about their employees, but they will certainly always care about their shareholders. And I think that's what shocks me so much about myself that I'm here fully talking along the lines of shareholder capitalism and Friedman. But maybe I have just become a bit more pragmatic.
KIMBERLY NEVALA: Well, you always have to find what motivates people and go there. Right?
So you've been mentioning a lot of these issues in the context of tech companies. By which some listeners may assume you mean folks developing AI systems for their own use and potentially for use by others. I think we could make an argument that says all businesses today are tech companies. And probably all businesses, even those that don't develop AI algorithms, will very likely deploy and utilize AI algorithms.
So what kind of additional pressure or due diligence does that require from businesses in general, not just necessarily the tech titans?
DOROTHEA BAUR: Yeah, I think that's really important, although legal discussion. I'm not a lawyer. So I cannot really go into the details there. But it's essentially a question about product safety. Because if you as a company buy some other kind of technology, some hardware machine, the producer or the vendor is liable for the safety if you use it correctly.
So I think that's very much at the core of the upcoming AI Act in the EU that they are trying to kind of transfer this notion of product safety to the AI context. And they're trying to figure out who should be responsible for what happens when you use AI, or the ethical issues related to AI. And I just read it up again to make sure I'm right here. But apparently, the AI Act puts the onus of duties and rights on the initial provider.
So that means if you buy it, you should be able to trust that everything is OK. But of course, this is also being criticized because it kind of means that you can lean back as a user, say, well, I just bought that. I'm just using it. And it has been sold to me. So where do you draw the line between holding those who use it accountable without putting too much of a burden on them, especially if we are talking about SMEs, who just they use software? They will use an AI based software. What is a reasonable expectation in terms of how much they should know about how much they should look inside the black box that AI effectively is?
KIMBERLY NEVALA: So we may be having a lot more conversations moving forward about supply chain in the context of AI enabled products, specifically. Yeah?
DOROTHEA BAUR: Exactly. Very much so. Yes, I think that's really-- that's coming up now. Yeah.
KIMBERLY NEVALA: Now another thing that I find really interesting is some of the terminology that we use. Ethics, or ethical AI, fields as you said early on can seem overwhelming and a little threatening. We talk a lot then about responsible AI, more trustworthy AI. I'm wondering in your thoughts about how do those concepts relate? Is responsible AI and the way that it's being practiced the same as developing an ethical AI practice?
DOROTHEA BAUR: Yeah, I know. There are a lot of terminological discussions. And one of the good things about not being an academic anymore means I don't have to be so strict about terminology. So it feels quite liberating.
At the same time, I still remember why terminology is important. Because it can confuse people. And I really, I mean, as long as people explain to me what they mean by a certain term, I don't have a preference that I say we should always talk about responsible AI or ethical AI. I think I just want to know for people when they use such terms, they should make it clear what they mean. Because sometimes, they have very strong ideas and convictions behind using certain terms. I don't. But for me, many of these terms, trustworthy, responsible, ethical can be used interchangeably if you make a good case for why they are interchangeable.
The one important question that always pops up and that is in fact legitimate, even though I'm also guilty of using it the wrong way, is when you say ethically, you could imply that AI itself has agency and it can be ethical, which of course, I strongly deny.
But still, it's just it's easier to talk about ethical AI instead of ethically deployed, developed, used, sold, and monitored AI. So I think that's something that we should make sure that we don't forget this when we talk about ethically AI that AI does not have agency. And an algorithm will never be ethical. It's just, I think it's laziness when we use it that way.
KIMBERLY NEVALA: Yeah, such a good point. Marisa, who I know you know well as well, was talking about the pros and cons of anthropomorphic language in explaining how these system work. And there's good and bad in our ability to use that. So such a great point. But I wholeheartedly agree that AI systems cannot be ethical. It's our use and deployment of them that confers that status.
Another trend I've found interesting, and I don't know that I have an opinion on this yet, is that in a lot of cases, organizations are really going back to and leveraging a lot of their risk management practices to try to support and develop their responsible AI processes and procedures. So this idea of being able to use risk management to identify risks that need to be mitigated or avoided. It somewhat aligns to a profusion of AI ethical audit type offerings as well.
And certainly, I think risk management, as it's been traditionally practiced, has a place in this puzzle. But I am wondering if does that focus on risk actually risk missing out on a broader consideration of rights, or maybe better stated, values?
DOROTHEA BAUR: Exactly. You're actually anticipating what I wanted to say. There is a certain conflict between risk perspective and the rights perspective.
Because if you look at something from a risk perspective, you're only thinking about the consequences of developing or using something. And you will only act if you know that you will be negatively affected, or someone who you care about for whatever reason will be negatively affected.
But a rights perspective would be ex-ante, as we say, to really say certain rights always have to be taken into consideration no matter what risks, what the risk score is. And I think that's also what people criticize about the EU AI act, which I just mentioned. Because it's a risk-based classification. They say the higher the risk of a context in which AI is applied, the stricter the regulation that we need.
So they say in high-risk contexts like, we need stricter regulation, which makes sense. But still, it's exclusively risk-based focus. And it's also not looking at people as subjects of rights. It's only using at people as being affected. And so that's maybe why a risk-based approach, again, is very attractive when you talk about AI ethics to corporate executives. Very much so where there is, I mean, I hate using the term, a win-win situation between ethics and business because they understand the language of risk.
But it will never, as you said, cover the full spectrum of ethical issues. And there's always a danger of missing out on aspects if you only focus on risk.
KIMBERLY NEVALA: Now you have a business consulting practice. And you work with organizations to evaluate and implement ethical practices and processes. What dimensions do you assess when you're evaluating ethical maturity or ethical practice?
DOROTHEA BAUR: I don't have a checkbox approach. I always try to grasp through qualitative approaches, qualitative interviews, what kind of awareness or level of reflection company employees, or whoever I'm talking to, represent. And this is really the first task is basically what we started today with, what does ethics mean? Do we have any awareness? Is there any shared language for ethical issues in a company, et cetera? And have they internalized it? Or even if there is some ethical awareness that they know that a company does have a corporate code of ethics, whatever.
The way they talk about it gives me an indication of the level of maturity. If someone says, I know they say there is something like this code of ethics and that we should do that, you can really feel that, no. This hasn't been internalized. So from the way people talk about ethics, you can really tell something about the ethical maturity of a company. And it's got a lot to do with corporate culture, which of course then translates into behavior of individuals. So this for me is, it's very much in talking to people that I really assess how mature the ethical culture or ethics the company is.
KIMBERLY NEVALA: Yeah, and I love that direct line between culture to behavior because very often, I think it's really easy to talk about these things. But if you really want to understand what someone's culture or beliefs are, you need to look at how they operate in the real world. So very interesting.
Now before we finish, and I could go on for a very long time with you, I find this area fascinating…I saw your TED Talk from about a year ago, if that. But there was this really fascinating - and I'll paraphrase because this was what I took away from it and may or may not have been what you were getting at observation that in the end, our freedom as individuals, as humans and societies comes from having the power to decide. Or from the power of self-determination.
And you pose this question of, how can we truly be free when we delegate - if we delegate - decision making to something else? In this case, an AI system. So I was interested in your current thoughts and thinking about how we ensure we don't inadvertently subjugate individual freedoms and decisions when we're delegating these types of decisions to AI systems?
DOROTHEA BAUR: Well, there is a technical answer to that, which is through measures of accountability, et cetera, and that's what you're trying to do. We defined, as we said, now, who should be held accountable if something goes wrong, and auditing systems, et cetera. That's one way.
But on a philosophical level, I think we need to remember that humans are, as far as we know, the only species who has the ability of being responsible thanks to our force of reason and our ability to discursively legitimize our actions, et cetera. And because we have this ability to be responsible, we have the responsibility to keep this ability alive. So we must not -- we are actually morally obliged to keep responsibility alive in this world.
And that's a philosophical answer. But I like that very much. And it comes from Hans Jonas, who was a German immigrant, Jewish-German immigrant to the US, philosopher, and who said that the mere fact that humans are the only species that is capable of being responsible means that we are responsible of keeping responsibility alive.
And that's for me always, we must not do away, we must not abolish responsibility. And if we follow the way of using AI blindly, or applying it without understanding it, we are at risk of erasing responsibility in this world.
KIMBERLY NEVALA: So I guess as final thoughts, what would you like to see happen, whether that's organizationally, as individuals, as broader society, to ensure that we do not, in fact, give away or not rise up to that responsibility?
DOROTHEA BAUR: Well, I think we are on an OK, journey.
I don't see AI as getting out of control yet. I think especially, certain companies, but also certain geographical regions, I don't want to be too Eurocentric here, but have made efforts, and have addressed the issue, and are trying to really set reasonable regulation and guidelines for how we develop and use AI. And I think that the legal side is one thing, as I said. The corporate ethics plays another role. Individual ethics is a third level. So across all these levels, state, or transnational regulatory level, corporate level, and individual level, we need to work on raising awareness and stand up to our responsibility again.
KIMBERLY NEVALA: Excellent. Anything else you'd like to leave with the audience that we may not have touched on?
DOROTHEA BAUR: Not really. Thanks a lot for making my brain boil in my head if I can say so. It was a really good and inspiring conversation. I mean, your questions made me think a lot. Thanks for that.
KIMBERLY NEVALA: You're welcome [LAUGHING].
Thank you, Dorothea. I think you have such a definite knack for making ethics both relevant and accessible and understandable. And as everybody - and I do mean everyone - will either develop views or be affected by AI-enabled systems, we definitely need more of your like in this space. So thank you so much for sharing your thoughts.
DOROTHEA BAUR: Thanks again for having me. Thanks.
KIMBERLY NEVALA: Awesome. Now next up, we're going to be joined by Roger Spitz. Roger is the CEO of Techistential. And he's going to discuss his triple A model for human decision making in the age of AI. So subscribe now so you don't miss it.
Creators and Guests
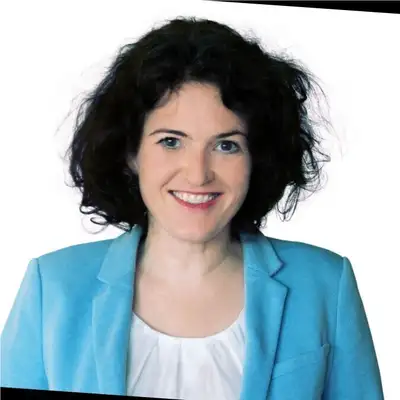
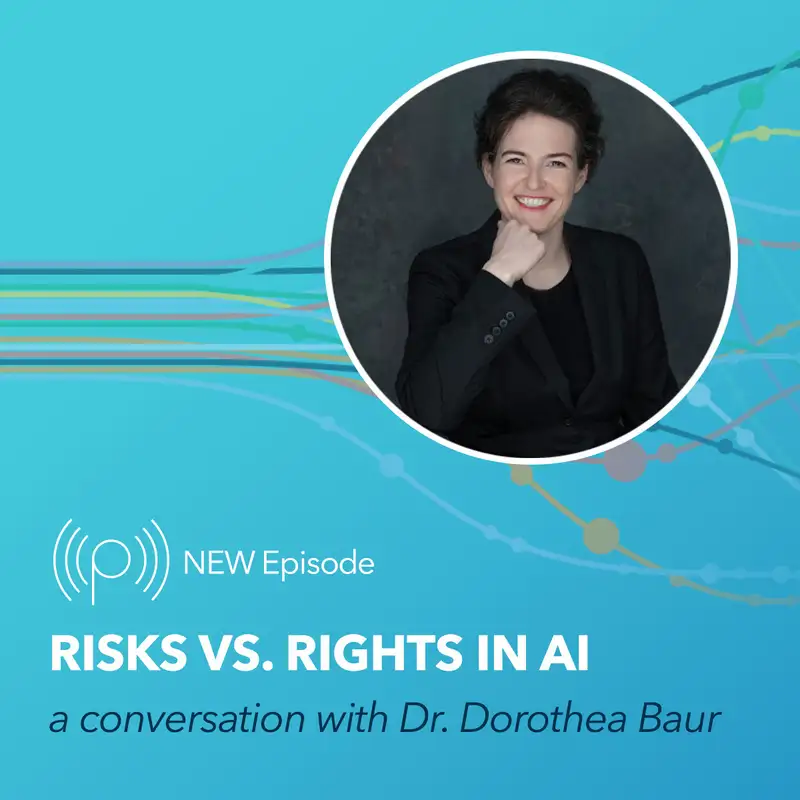