In AI We Trust with Marisa Tschopp
KIMBERLY NEVALA: Welcome to Pondering AI. My name is Kimberly Nevala and I'm a strategic advisor at SAS. In this season, I'm so pleased to be joined by a diverse group of thinkers and doers to explore how we can all create meaningful human experiences and make mindful decisions in the age of AI.
In this episode, we welcome Marisa Tschopp. Marisa is a human AI interaction researcher at SCIP AG. She's the chief research officer at Women in AI NP and the co-chair of the IEEE Agency and Trust in AI Systems Committee (LAUGHING - that's a bit of a mouthful).
Marisa looks at AI through a psychological lens and joins us today to discuss our evolving relationship with AI systems. Thank you for being here, Marisa.
MARISA TSCHOPP: Thank you for the invitation. I'm really looking forward to our discussions.
KIMBERLY NEVALA: This is going to be fascinating. So tell us, how does one become a human AI interaction researcher?
MARISA TSCHOPP: That's such a good question. And if you would have asked me this 10 or 15 years ago, I would have said, what are you talking about? I am here. I'm a cosmetician. I am painting people's fingernails. So I do have a bit of a weird, well, CV, so to speak.
But I must say that I have always been extremely interested in people and understanding people. And, well, to be more optimistic, how to help people. And whether this is by painting nails or in the age of technology, I think I totally found my field here. So over the course of my studies, at one point, I started to be absolutely fascinated by technology. Not so much by technology per se, but how people interact with technology and how technology changes our minds, our behavior, our interactions with other people. It fascinates me. It also sometimes scares me. And often, it also excites me. So I think it's, for me, just a very great place and a very great setup at the moment.
KIMBERLY NEVALA: And you are a psychologist. Is that correct?
MARISA TSCHOPP: That's correct, yeah. So I've studied basically-- I was focusing on consumer behavior and organizational behavior. So how people behave in organizational contexts or when they look at products and stuff like that. And now at the moment with the institution in Germany, I am looking more into relationship patterns. So also focusing on the human and how they interact with technology.
KIMBERLY NEVALA: And for those of you who are not already following Marisa on Twitter or LinkedIn, you really, really should. I will tell you it's one of the most fascinating, curated feeds I look at and has become one of my favorites hands down: lots of challenging and interesting topics.
MARISA TSCHOPP: They're all very personal topics I post. So it is carefully curated. But it's really just things that are very important to me.
KIMBERLY NEVALA: Excellent. You talk a lot and, I know, research and speak about things like trust and trust in AI. And certainly today, in a lot of tech companies, a lot of media, we're seeing a lot of articles that tout the importance of developing AI systems that people can trust.
And this concept of trust is a curious thing. If you could start us off by helping us understand what does it mean to trust someone, another person? And then maybe we can extend that to see if that same definition really applies to an inanimate system such as AI?
MARISA TSCHOPP: Yeah. Thanks. That's a brilliant question. Yeah, as you already mentioned, my major passion is trust and investigating this weird feeling everybody knows.
Every human being can say I trust this, that, or him, her, whatsoever. But we don't really know how to define it and what it really incorporates. (Trust is) something we experience-- but it's one of the hardest things to explain. So, of course, there are a myriad of theories on interpersonal trust. So how do humans trust each other? And what does that mean?
But to keep it simple, I think -- and also to relate it to human machine trust later on -- I want to go to one theory where you basically, where to trust someone incorporates that someone is willing to be vulnerable towards another person.
So if I trust you, it's a gift. It's a gift. Because I open myself to you. I show you my vulnerabilities. Because if I trust you with something, you have the opportunity to hurt me. But you also have the opportunity to take this gift and grant it and keep it. And basically, the study of trusting someone is rooted in studying trustworthiness.
And if we look at humans, there are these three pillars that have been investigated a lot, which is integrity, benevolence, and abilities. So these three pillars are what we evaluate, basically, sometimes more intuitively, sometimes more really thinking about it. When we say we trust somebody we think about what can this person actually do? Is this person benevolent? And if that person (has) integrity?
So these are the things we kind of ask ourselves when we evaluate and think about or feel if we trust somebody.
KIMBERLY NEVALA: So when we think about "trusting" or putting our trust in something like an AI-enabled system, are these characteristics that we are trying to design into the system? Or are we really talking about trusting the system itself? In other words, should we be talking about trusting AI systems or trusting the people who design and deploy them?
MARISA TSCHOPP: Well, that's the question we all try to answer at the moment. So obviously, I don't have answers. But I do have some hints and some ideas where we are at. So maybe to get back to what I've just mentioned - how is trust in a technological system different than humans?
So actually, a lot of studies have come to a place where actually it's pretty similar. So when we talk about trustworthiness of a machine, we also talk about these-- meta-analysis have found that three dimensions are relevant. Which are performance - what can the system do, process - how does the system do it, and purpose - why does it do it?
And these are pretty similar to the three dimensions I mentioned when we talked about interpersonal trust. So it is similar. But it is also different. So you can see the similarities. But of course, you cannot take them one to one.
And also, specifically in the human-machine context, there are the similarities. For example, there's a situation that is characterized by uncertainty. You don't really know how these machines are making the decisions. And you don't really know what's happening. You cannot really explain why these things happen. And on the other hand, you don't really know what the companies are after. But I think the most important thing I want to mention here is that if we talk about trust in machines or trust in AI, we always need to talk about the end goal, the target.
So for example, if I say I trust Amazon to deliver this prospect within a week, I do. But do I trust Amazon to handle my data ethically? Maybe not so much. So it's the same entity. But there are two different outcomes. So we want to always look at what kind of goal are we talking about?
We're not just talking about AI. So that's a very general question that is actually-- in one article I wrote-- it's the wrong question. The question, do you trust AI, is actually a wrong question. Because you want to ask, do you trust the AI to do what? To achieve what? You always want to put it in context. So that's important.
And the other thing you mentioned is who's the trustee? I am the trustor. I'm the one giving my trust away. And we don't really understand the whole framework of trust. Who are all the actors? And it's very, very complicated. And the more we know about it, the more complicated it gets unfortunately.
So in one of our studies, for example, we did find out, though, that people really think about whether is it the AI as an artifact we put the trust in? Or is it the company behind it? We found significant differences in trust they place in the artifact and the trust they place in the provider. So this shows that users are making distinctions. So we have to go further than that. But this gives us a direction. Actually, users are smarter than we think. So let's start here.
And in one of our qualitative studies, we also found a lot of people telling us that they stated things like it's not the AI we don't trust. It's really the people behind it. So it seems like there is still this interpersonal trust concept flying around somewhere that they place their trust in the people and maybe rely on the machines that they trust or that they put their trust in. So it makes sense that you could distinguish trust and reliance if you want to better understand all these kinds of frameworks.
KIMBERLY NEVALA: Interesting. So is there also a difference or a bridge we need to make between the concept of trust and trustworthiness? Or is that just splitting hairs?
MARISA TSCHOPP: That's a huge difference. And I think I point this out in, basically, every talk I give.
So when we talk about trust, we're talking about a human attitude. We're talking about something that's happening through emotional and cognitive observations and stuff like that. So we're evaluating things. And this is how our attitudes is formed.
When we talk about trustworthiness, we are talking about the properties of machines. So trustworthiness is, especially in this human machine context, is a very technical term. And it's actually something where I say if you as a company stop talking about trust, just talk about trustworthiness. Because this is what you can control best. This is what your best at. You can think about what do I have to do to be trustworthy?
If companies focus on trust, then we're pretty close to manipulating people. Because trust is an attitude. And we want to make sure that we're not doing this because we want to manipulate people. We don't manipulate their levels of trust.
But what we can do, what we can control is how can we, as a company, be trustworthy. And there are a lot of studies, a lot of indicators that explain how can we at a company can be trustworthy.
KIMBERLY NEVALA: Are there some key characteristics of what makes a company and their systems trustworthy?
MARISA TSCHOPP: Well, as I already mentioned, I think as a start, it's always good to start with the model by Lee and See. Because it's easy to grasp.
At the beginning you focus on the performance. What is the system capable of? And if you are able to communicate this without any hype and without overblowing expectations, then this is what you should do to be trustworthy.
Then the second pillar was process. And this incorporates how, does the system make a decision? And this incorporates a lot of complicated terms such as explainability, transparency. How do we handle consumer data, and stuff like that? And this is that pillar.
And the third part is why-- are we really doing what we want to do-- like selling XYZ? Or are we also gathering data to, I don't know, teach other algorithms something else and make profiling and all these kinds of things? So what are we actually really doing with this product?
And within these three pillars, so to speak, you can also just grab a guideline, for instance, the EU Guidelines for Trustworthy AI. And then you can see how they fit into this framework as well when we talk about transparency, compliance, security, data governance, and all these kinds of things. So you can take one of these guidelines, they would fit perfectly. They're voluntary. You can just use them. And on a final note, you can also look and find companies or more and more companies who do labeling, who do certifications, who do audits to standards, improvements, and stuff like that, that help you to at least adhere to the most important governance guidelines.
And last but not least, at one point, this will lead into laws. You must be lawful at one point. And now you're doing all this the preparation for that if you're already adhering to the guidelines of trustworthiness to-- when the law comes, the AI Act, specifically in Europe, then if you're already there-- if you're already adhering to these standards, then you're best-equipped to not having an illegal product or any illegal practices on board.
KIMBERLY NEVALA: How important is it also that we become more mindful about the language we're using, particularly when we are communicating, whether it's marketing or just discussing and educating and writing about AI capabilities? There is a lot of, I would say - maybe it's not inflammatory, and maybe not even intentional - but I think misleading language, things that we'll say. We have an AI system that thinks you think this. Or we have an AI system that knows when you are depressed. There was a recent article from LinkedIn that you had tagged that said: “algorithmic integrity cannot be evaluated without understanding the algorithm's thinking”.
So how does our tendency to want to, for lack of a better word, anthropomorphize some of how these systems work so that people understand it helping us and hurting us overall?
MARISA TSCHOPP: Yeah. That's a crucial and very, very complex topic. Because it's so nuanced that it's really-- it's so hard to define benchmarks. So maybe to start off with and most obvious, I call it the Muskian marketing strategies. So sorry. He won't like that. But it's OK.
So anytime you just absolutely overblow the capabilities of a system and give expectations that are just unrealistic. And this is just really, really hurting the whole field but also, of course, the end user. Talking about, for instance, naming you’re driving assistant an autopilot whilst you still have to put your hands on the wheel. So there's nothing such thing as an autopilot. But this flows into all other industries as well. But you must be aware of any kind of Muskian market strategies and flag them. You can even think about how to make them illegal.
But to go a little bit more into the nuances of anthropomorphizing language, this is one of my favorite topics. Because on the one hand, anthropomorphism is good. Because it helps us laypeople to understand things better. And it started with weather predictions where we gave storms names and talking about, listen, Storm Katrina is coming. And it's dangerous. And it will destroy us.
So it started with these kinds of things. But it helps us learning about the accuracy and the phenomenon very instinctively fast. And you grasp it right away. When you use anthropomorphized language, you understand things way faster, especially if you don't know what we're talking about.
For instance, talking about cyberattacks, the virus. Everybody knows how a virus works. But I can guarantee you that nobody knows how a computer virus works. You get how it works just because how a virus works. You know there's something bad. You get infected. It kind of creeps around. Maybe you don't even know you have it and all these kinds of things. So it's extremely helpful to explain things.
On the other hand, there are also very negative effects that come with anthropomorphized language, especially if we say the AI… Let's start with the AI. There is no such thing as the AI. It's not an entity. It's not like the thing moving anything. It's an algorithm, or it's a system. Or it's something. It's an artifact programmed by humans. So there's no such thing as the AI or an AI.
On the other hand, the AI does not think. It does not even learn. It doesn't do these things that humans do. It doesn't learn the way humans do. Like machine learning: it recognizes patterns and it makes statistical associations and then identifies outputs based on these patterns. But it doesn't learn the way we do. It doesn't understand context, et cetera, et cetera.
And the danger in this is that people have absolutely overblown expectations of the performance which leads to misuses of AI, also wrong business models, even to AI winters that destroy the whole field. Yeah.
I don't have an answer to that, to be honest. But we have to be extremely, extremely cautious how and where we anthropomorphize. And we really have to nail this down where and when and how this is best. I don't have an answer to this unfortunately. It's just that there are good ways and bad ways.
KIMBERLY NEVALA: And would it be fair to say, also, that whether we're promoting products or I'm writing articles that we should also be thinking carefully about what our intention is? Because, again, as you said, there's nothing wrong with helping people contextualize and understand things in a readily common context.
On the other hand, if we're using that as a tactic, I suppose, to lower resistance or lower skepticism. I worry more about this not so much for folks in the field, but for laypeople. You used the Amazon example earlier, which says, yeah, I trust them to deliver a package in a week. However, I don't trust them to use my data ethically. I think the public is more and more educated these days. But not everyone even thinks about that or understands that there is a trade-off to trust them to get your package there. There may be trade-offs you're making that you don't even know about in terms of your data security and privacy. So how do we more broadly address this issue and try to hedge against general overconfidence in systems that occur from all of these different influences?
MARISA TSCHOPP: Again, you're asking a tough question here.
We literally just three weeks ago at the university in Switzerland, we had a track on over-trust and how to mitigate over-trust in AI and automated technologies. So as we're really simply just on it trying to better understand the field and trying to co-develop in a participative approach in integrating stakeholders, we are really in a development stage.
So at the moment, there are strategies you have to apply from the technological side. How to design for calibrated trust. So to use a technical term here, calibrated trust is when we talk about trust, that corresponds with the level of performance of system. And you can see this from two sides.
The one side is, how do you develop a product to foster collaborative trust or to calibrate trust? So how do you communicate the performance? What kind of algorithms do you use? What kind of transparency level do you use? How do you implement explainability and through what ways? We don't know. Does a computer explain itself? And how does that affect the user and so on? We don't have all the evidence here, the scientific evidence.
So that's the one thing. And on the other side, from the peoples, from the humanity side you want to think about, what do we have to do to calibrate our trust ourselves? How can we exercise meaningful agency?
And, of course, there are a lot of approaches in education and knowledge and also from the legal side. If I know that the European Union is working hard to build laws governing AI, there must be something going on.
So there are all these cues that we, as users, are now soaking in, which will eventually help to calibrate this level of trust. And on the other hand, however, there are for these very, very strong forces that prevent us from calibrating our trust-- For example, convenience group pressure, having no other option, having not the resources to monitor.
My friend uses WhatsApp. So I use it as well. Because I don't want to be left out. There's no other option. Then people also resign: they're like, I don't even know what this all means. I'm just going to click OK - take my data. As long as I can share my pictures, I'm fine.
And this whole fear of missing out and all these nudging people from design perspectives and so on. These are things that even if we're trying the best we can to be as thoughtful about our decisions, there are mechanisms that influence our behavior in ways that we almost can't control. And this is extremely dangerous.
KIMBERLY NEVALA: Yeah. Nudging is an interesting phenomenon. And, again, another article I keyed into through some of the feeds that you put in was talking about the ability of an AI to draw your face from hearing your voice. And it was fascinating. Because I instinctively said, that's BS before I even really looked at it. I started to read it before I looked at the explicit pictures. And when I looked at the pictures, I thought those don't look at all alike. The woman's female. She's got dark hair. And looks maybe a little bit LatinX. I didn't think there was even really a passing resemblance. But the title of the article was ‘draws your face with surprising accuracy’. I started to think about, again, the psychology. Which is, as you said, prompting someone to see what you want them to see. If I just read the article (title) and maybe didn't have the background… when I looked at those comparative pictures, I wonder if I might have seen more of a likeness than I did. And then I worried about, do other people see more of a likeness because they're influenced to do so or not?
MARISA TSCHOPP: Yeah. Yeah. And there will be a myriad of people who think, wow, that's amazing. I want to work in this field. That's great. The big tech people, they get so excited about these things.
They don't even mean it. They're like, wow, I can build something really cool. And it kind of works. It gives me some crazy outputs.
And they're super excited about these things. So I totally get all the sides from working in academia as well as in a cybersecurity company. I get all kinds of weird inputs and fun things. And I get to know all these interesting different characters and stereotypes myself that I have to think about. Definitely.
And I think to get back to this article, and, of course, I also have an agenda when I post this kind of article. I know people only read this headline. And then I'm thinking about, OK, what do I say about this article that fits my agenda? Because I'm also out there to make business. I'm also a businesswoman.
And I just wanted to say everybody has an agenda. And the other thing that's kind of maybe out of context, but to just close this topic also is my friend who's a great statistician. She gave a lot of great inputs as well thinking about, listen, you have no idea how many tests with what kind of people they've done. And maybe they're just showing those 10 people with the great output. And you don't even know what the population of the sample even looked like. So she also has her agenda. Because she's doing consulting on statistics. So I'm just saying we all have our hypotheses, our agendas in our head. And this is how we conceive the world. This is how we communicate to the world.
And I think this is something we all have acknowledge and also try not to put too much value on and try to have a meta perspective. Why is this person hosting this? And how could we see this differently? And how can we add another perspective to this?
KIMBERLY NEVALA: That's such a great point and circles back to the conversations we've certainly been having about diversity and non-exclusion and getting all of those different viewpoints.
Now, I'd like to take a slight turn. I know another area of research that's fairly early and emerging is looking specifically at this idea of human and AI relationships. Or how people develop relationships to their devices. And there's two areas I'm interested in your thoughts, in what you're seeing.
One is, I will tell you-- and again, putting my own personal biases out there-- I am highly turned off, I think, when I see a lot of companies -- whether it's a humanoid looking model or a digital assistant that purports to be the perfect wife or to replace the need for elder care. And I worry about us trying to use these systems to not enable human connection but to, in fact, replace them or augment it (in places) where today maybe we don't have the social connectivity and nets that we need. So I'm interested in that.
And this idea that people really form an attachment to their device or to their AI assistant is intriguing to me. Can you talk a little bit about the research that you're doing and some of these phenomena and what we may be looking into as we move forward here?
MARISA TSCHOPP: Yeah, yeah. It was a great listening to you. Because I think I'm the opposite. I'm totally fascinated by talking to machines. I love watching robots. And I get very enthusiastic when I see robots and when I talk to my Google Assistant and so on. It's so great to see all these different receptions.
When I began working in the field, I really was super focused on trust and performance. Looking at how these AI assistants - I'm focusing on conversational AI in my research - how they are perceived, how they're trusted, and how smart are they? And how does that affect trust?
Because before I started my research, I was doing something differently. I was working in a university for digitalization of blah, blah, blah. So it was also for me new working with digital assistants. At one point of my research - I think it was like two or three years into my trust research - I turned off my Alexa. But before that, I said goodbye.
And that was the point I was like, well-- what the--
KIMBERLY NEVALA: Wait a minute.
MARISA TSCHOPP: What's happening there? And this is the point where I said, OK, wait. Something's going on with me. And this is often or sometimes, or at least for me, is how I think, OK. I want to understand this. I want to delve deeper. And I want to explain this. And I want to see how other people perceive this.
And this is where our or where we started doing research on relational patterns with AI, conversational AI. And we wanted to find out do people develop relationships specifically with conversational AI like Alexa and Siri or like they do with humans? And it's basically the same approach as with trust.
We have the interpersonal trust, the human-to-human trust theories. And we have translated them or repurposed them to a human machine trust. And we're basically taking the same approach. So we're looking at interpersonal relationship series and repurposing them for human machine relationships-- so the same things in a different context. And before maybe I delve deeper into the research, I think what triggers me most is, of course, finding out what can we find out from a scientific point of view?
But what triggers me and what keeps me awake at night are the articles I read about people forming relationships not so much with Alexa, but with other AI companions or with the Microsoft chat bot Xiaoice in Asia, where they get so attached to this chat bot that they feel bad or even depressed when they had to abandon this kind of relationship.
And there's also emerging research that shows that people report sadness, that they report addiction towards interacting with this 24/7 available companion, fake person, however you want to name it. Because they built this attachment. And they're somehow unable to release that.
And I'm pretty sure, although I don't have evidence for that, and this will spill over to human interactions. I'm so sure about that, that this will interfere with and influence how we also interact with each other. And I think the effect will be rather negative than positive.
KIMBERLY NEVALA: Interesting. So is this a primary area of focus for you and your team moving forward then?
MARISA TSCHOPP: No, that's my hobby.
KIMBERLY NEVALA: It's my hobby? You have the most interesting primary job and the hobby. I love it.
MARISA TSCHOPP: And my actual area is really very theoretical. So we're really looking at these interpersonal models. And we want to understand how do people perceive the relationships with conversational AI. And in our first study, we had some really interesting results actually where people…maybe, let me ask you a question.
So you know Alexa or you know Siri or Google Assistant? Let's imagine you have three different types you could have a relationship or can perceive relationship with. It's more like the servant relationship-- like you have a digital assistant. I have an order. You are the servant. This is one kind of type.
The other type is more like an exchange partner on rational basis, like an employer or I give you something. You give me something in return. But we're very equivalent on human level. And we just negotiate on the things we want. I give that to you. You give me money back and so on. That's the second type.
The third type of relationship would be friend like, peer like. You're my friend. I do everything for you. We're the same kind of person. We share visions, morals, goals, and so on.
What would you guess, what would be your best guess how most people perceive the relationship with Alexa, for instance?
KIMBERLY NEVALA: Gosh. Maybe I'm a cynic. But I'd be concerned that most people would say number one. And I would be horrified if the majority had actually said three, which was that friend relationship. So I'm going to say that maybe it was three. Was it three? Did they think of it as a friend?
MARISA TSCHOPP: No, it was not. So actually the majority of people identified or characterized the relationship as a servant: master/servant relationship. A small type, a very small majority chose or perceived their relationship as with a peer-like companion type of a thing.
KIMBERLY NEVALA: Whew. Interesting.
MARISA TSCHOPP: Which is also kind of weird. Because a lot of research-- and they're also working-- Google Assistant, they're working hard towards developing their assistant as a friend, as a peer, as somebody who's always there. They're putting in emojis and all these things.
But the most exciting thing we found is that actually, greatest parts actually was the type two part. It was the rational interaction. But there was some equivalence attributed. There was some we're not the master/servant type. It was really this kind of exchange part.
And this was also the relationship pattern that was the one with the most significant predictions and how people perceive the other variables such as trust, such as warmth, competence, performance.
KIMBERLY NEVALA: Interesting.
MARISA TSCHOPP: So we believe in our first study that this kind of servant/master relationship is the default relationship. And the other things they kind of develop through the way. We don't know yet how.
So there are people where we have all these kinds of relationships we perceive within our interactions.
And this is exciting. Because we have a tendency to categorize they're either the servant or the friend. But I think it's very exciting. Because we could show or find that there are multiple ways of conceiving or perceiving these digital assistants and to interact with them in various contexts maybe.
Maybe this will also change over time. And this will be our next study. So we want to find out will this change over time? Will we start with more of - like I did - more with the rational servant/master type. And at one point, I'm saying goodbye. Like, wow. You know. Does that change over time maybe? Does that have something to do with it? We don't know. But that will be the foci of our next study.
KIMBERLY NEVALA: Oh. This is absolutely-- it's a fascinating conversation. And I think it will be fascinating to see what those results are. I will say that it leaves me with increased optimism for the future that although - I don't think this is positive- today the foci is the slave/master relationship. Years ago, I saw a kid bullying Alexa. And I thought, oh, this is not going well and really testing out what was the worst insults and questions they could ask. And I thought hmm. But there's a lot of optimism based on what you've said about our ability to perhaps evolve beyond some of that. So we'll definitely have to keep an eye out and hopefully have you come back and talk about it.
I think this area is incredibly interesting. And for me, I find it just personally challenging as well. So I'd love to just keep in touch.
MARISA TSCHOPP: Definitely. I couldn't agree more. It's challenging and exciting and sometimes also scary. But all in all, I'm also-- I would say I'm a skeptical optimist.
Overall, I believe we are definitely going into the right direction. We just have to get this, to fine-tune and work on all these details hard. All in all, I'm very positive that we're going to get through this. And we're going to do a good job hopefully.
KIMBERLY NEVALA: That is amazing. Thank you so much. And as I said to everyone listening, if you're not already following Marisa, you absolutely should be. It's a very varied and stimulating set of content that you do curate. So thank you so much for joining us today.
MARISA TSCHOPP: Thank you so much, Kimberly. It was a real pleasure.
KIMBERLY NEVALA: All right. So next up we're going to have Dr. Dorothea Baur. And she's going to join us to discuss how do we strike a balance between a risk and a rights-based approach to AI ethics. Subscribe now to Pondering AI so you don't miss it.
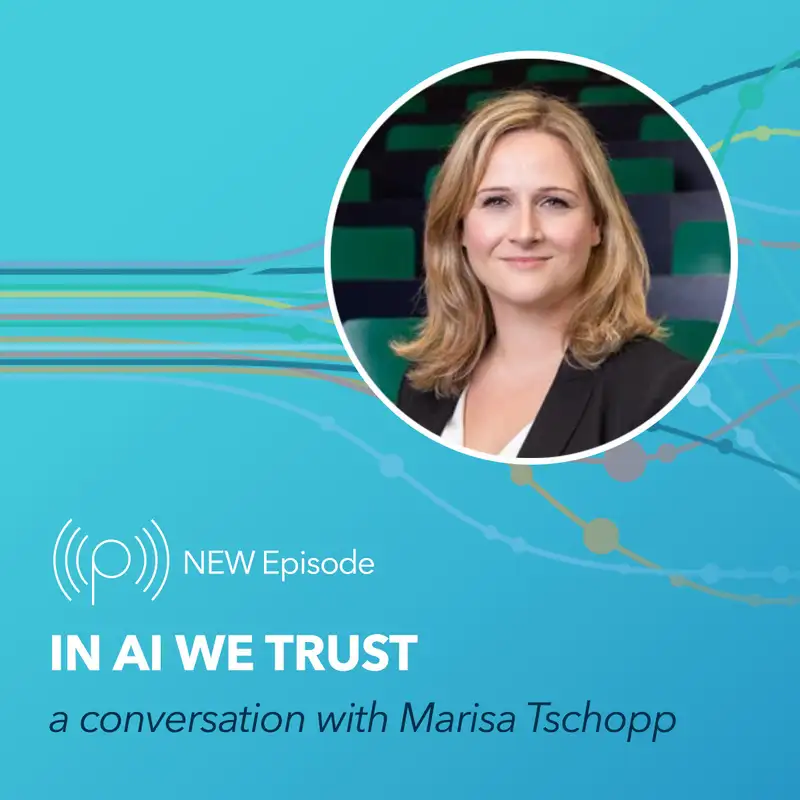