Automation, Agency and the Future of Work with Giselle Mota
[MUSIC PLAYING]
KIMBERLY NEVALA: Welcome to Pondering AI. My name is Kimberly Nevala, and I'm a strategic advisor at SAS. It's been an absolute joy to be hosting our second season in which we talk to a diverse group of thinkers, advocates, and doers ensuring our AI-enabled future puts people and our environment first.
Today, we're joined by Giselle Mota. Giselle is currently a Principal Consultant on the Future of Work at ADP. She thinks critically and advises organizations on topics related to automation, human agency, and learning in the age of AI. Welcome, Giselle.
GISELLE MOTA: Thank you.
KIMBERLY NEVALA: Now, you bring such unique insight and passion into where and how technology can both enforce and break down barriers in the classroom and at work. Can you tell us a little bit about your path to this mission and your current role?
GISELLE MOTA: Yes, absolutely. Thank you, again, for having me.
Yeah, the way that I got into this field hasn't been very traditional. I started out when I got out of college and I finished my graduate degree. I wanted to get into how people learn in academia and et cetera.
The reason why is because I struggled with my learning growing up. I have dyslexia, and it was always a struggle to be able to comprehend reading and to be able just to function normally. Right? And so I found ways, over time, to adapt and learn how to utilize technology even in times to help me figure out how I worked and how I could adapt and technology and the world around me could adapt to me. And so tapping into that brilliance and being able to be my full self, I wanted to share that with other people-- whether it was someone who was trying to get a degree, whether at the college level, whether it was a child, whether it was someone in the corporate learning space that wanted to continue skilling themselves.
KIMBERLY NEVALA: Yeah.
GISELLE MOTA: So that's how I got into it. And eventually, I did some work in academia, which led me into working in the corporate learning space. And I had my own consultancy around, how do you use artificial intelligence and adaptive learning technology to help people learn better at work? And here I am now, years later in the Future of Work. [LAUGHING]
KIMBERLY NEVALA: This is so awesome. And I want to come back and talk about how we actually can use AI for adaptive learning. But you talk about the Future of Work - it's interesting because we often hear about how AI is driving the future of work or the future of learning. And to me, this sounds a little strange. It's like we're anthropomorphizing the technology, as if it has agency in deciding what this sort of future looks like. So perhaps I'm splitting hairs, but should we really be talking about AI driving the future of work or learning or is creating that vision a job for us as humans, which we can then apply AI to enable or achieve?
GISELLE MOTA: Yes. I'm saying yes to both. The reason why I say that is because human agency and automation, they don't have to be mutually exclusive. They can coincide.
And so as long as humans adapt and evolve and as they continue to implement artificial intelligence and automation but they grow it in parallel to human agency, that's when we're hitting the money. That's when we're starting to use AI and automation as a tool to augment the human experience rather than using it as, hey, let's do more with less and the concept of, oh, we're just going to use this because it helps to meet the bottom line. So I believe that AI can actually be used to help preserve human agency.
KIMBERLY NEVALA: Are there examples you can give of (organizations) doing that? Because certainly a lot of the early work or the applications that we hear about are about automation: automating repetitive tasks, sometimes under a thin auspice of augmentation or assisting humans, but not always. So how can we be more deliberate and mindful about where and how we're applying AI to augment human work?
GISELLE MOTA: I'll give you real-life examples of organizations who are doing this. So I think of two cases.
One, there's this global engineering organization. And typically, they would have their inspectors inspect bridges. And they would manually have to go out there, use their human eye, inspect the bridges for safety issues. And sometimes, they would get it wrong.
So what this organization has done is they're using drones to pick up 3D imagery of these buildings and bridges and they're now feeding that. And through an AI algorithm, that can detect automatically where there may be an issue and a defect. So what that's done is actually improve the human agency, because now the inspectors are not just going out there and sometimes getting it wrong and perhaps even causing huge implications to human safety and infrastructure.
Rather, they're now able to rely on more of their intellect, rely on more of the decision and the analysis from the human being of, OK, this is what the AI is telling me. What is my prescriptive approach to this? What is my expertise applied to this? And what is the final decision that a human can make and apply to that? So that's one example.
Another one is in the area of retail. And I'm sure you've probably seen these store concepts come up in like Chicago, New York. You've probably seen Amazon Go. And Amazon Go is an interesting case in both manufacturing and retail, because you basically have this cashier-less store. Which-- you guys out in the audience, if you're not familiar with Amazon Go, they basically use artificial intelligence and apps so that you can literally enter into a store, make your purchases and your transactions, walk outside of the store, and never have had to have a cashier interaction with a transaction.
Why? Because there's cameras that are picking up with computer vision, the sensors are being used on each of the items that you're picking up off of the shelves. And with every interaction, AI is picking up your behavior. It's learning a lot of analytics on the consumer themselves, and then it's understanding that it needs to charge you. And you'll get charged on your mobile app.
Now, the thing here is that this technology has gone way beyond just Amazon Go. It's being sold into stadiums, airports, other retailers. Whole Foods has jumped on the bandwagon, and they've went ahead and partnered with Amazon. So the thing with this is that organizations that are leveraging this type of technology, they get asked what you're asking, Kimberly, which is like, well, what happens with human agency? Somebody asked, are there humans working in these stores? You may be asking as well.
KIMBERLY NEVALA: Yeah. Absolutely.
GISELLE MOTA: Yeah. If you look at their FAQ section, they say that humans are working in the store still. What it is that they can greet customers upon entry, they are helping to provide more of a consulting experience as the shoppers are going. They're basically saying that they're amplifying the work of what that cashier would do. Now, even if they've had to decrease the amount of people working in stores, the backside of this that people often don't think about is, when you move something with your right hand, something is moving with your left hand.
So if you look at what's happened with Amazon, they've had a super increase of other job opportunities come up. So increase in fulfillment orders, like the centers where they have warehouses. Those warehouses are equipped with robots that work alongside human beings. And now, you have humans who are working with iPads and certain AI-powered automation, and they're having to work alongside. This creates more jobs and more opportunities.
There's gig workers that now are working to assemble at home. So they'll go to your house. They'll assemble something that you ordered from an Amazon store. That's another opportunity. You have an increase in technology roles and security, research and development-- all kinds of things-- because now of the e-commerce aspect of what Amazon has become in retail.
So while that cashier may not have a physical presence in a store anymore, they could still pick up either other aspects of the work that they do within the store or they can go work in these other areas. So again, concurrently, whenever we see one thing happen and it does kind of take jobs and take certain tasks, you also see that-- there has been an increase in cybersecurity, data security because of what I explained about how these transactions occur.
And now, you need people to work in that area. So now, if you work for Amazon and you're in tech, you can go work in that area. If you're a diversity and inclusion expert, they need people to influence the ethics and the data governance of those tools that they're using. So I think that automation actually breeds an increased focus on human agency.
KIMBERLY NEVALA: That's interesting. It's a really different perspective, I think, sometimes where we see this as a sum game-- add something, take something away-- and see where we come out on balance.
Kate O'Neill talks sometimes about the difference between meaningful work versus meaningful jobs. And to some extent, what I hear you saying, too, is we might be automating some of these things that are pieces of jobs, but we should really be leaning in then to figure out what the meaningful work is that humans in fact want to engage in, can create value, and feel good about doing. Is that a fair assessment?
GISELLE MOTA: That's fair. And I think the other part of that is, as what I represent also in the Future of Work world in the space that I am speaking to many different prospects and clients, I always bring up diversity, equity, and inclusion. I bring up a positive future of work. How can you think about the people? So there must be also voices internally that kind of disrupt and hold organizations accountable. So as much as I'm a proponent for AI and automation and all of that, I am also a proponent and a voice for people and humanity. And I think more people like that are holding any type of AI and automation accountable for human agency.
KIMBERLY NEVALA: Interesting. So again going back to people making the decisions about where and how we put this technology to work and how we work with it.
GISELLE MOTA: Yes.
KIMBERLY NEVALA: Is that correct?
GISELLE MOTA: Correct.
KIMBERLY NEVALA: OK. Now, you have talked about AI in the context of HR and talent management. And as you were speaking about this previous example, where, hey, you may no longer be a cashier, but there's a lot of these other opportunities. Some of those might be what we would consider a lateral move. Maybe you're not cashiering but now, you're doing some work in the factory. But others might be moving into something like cybersecurity, as you said, or becoming a better customer advocate in terms of a sales representative and almost consultative -- which might take a different mindset and some different skills.
How should organizations be thinking about transitioning? Because it's not just move you from job A to job B. It's thinking about: what is the skills required? What's the aptitude? What's the attitude in these situations? Maybe the equation is we're taking x jobs away and we're adding these different jobs. But those different jobs are going to different people which, ultimately, leaves some behind.
How do people think about that transition?
GISELLE MOTA: The transition, definitely organizations have the onus and the responsibility. And I think years ago, when we were talking about learning and development, I remember, when I was in that world quite a bit, organizations put the onus quite a bit on the people. And it's like, well, learn and train and develop your skills. However, organizations have to give the time, the resource, the pathway to do that. So let's take this approach just kind of looking at steps backwards. When you think about an organization who's planning for their future.
You have organizations who invest their money into the client experience. And with that, they work in partner with the CIO, the CTO, the chief product of marketing, et cetera. At that time, they already know the direction in which they're investing and where they're moving in the future.
So for example, back to that store that's automating. They knew that, as we develop this for our customer experience and as we invest in these AI tools and they're over here thinking about the cameras that they're installing and the real estate of where they're opening these stores, they had to know that they were going to displace some people out of their jobs. So what pathways do you create from that very moment? It should be ingrained in the process of development and growth and expansion-- that, at that very moment, you are partnering with HR to conceptualize what are we going to do with people? What pathways do we create?
Then you can start to break it down and get very specific. OK. What are the skills that are going to be required for the roles that are going to formulate as new roles, or how do we take our current people and start creating pathways? There's technology today that's even AI-powered, Kimberly, that you can help understand the data of the skills, ontologies of a job. Right? You can break it down and understand. What are the skills required for that job?
You can then take your people and look at their skill sets and start to develop pathways and create pathways to get them from point A to point B. So it's a matter of just taking a step back and thinking about your people as much as you would consider any other resource in your organization. And how you would plan for that, I would say start workforce planning and skills planning to help your people get to where they need to get.
KIMBERLY NEVALA: We often talk about customer experience. We map customer journeys. So as you were talking, I'm thinking we used to, back in the day, sometimes talk about employee experience. But I don't know that we ever talked about employee journeys, per se, or at least as a broad conversation outside of maybe the sainted walls of HR--
GISELLE MOTA: Right.
KIMBERLY NEVALA: --in the back rooms there.
You mentioned something interesting, which is using technology to identify skills. And certainly, we have seen examples of AI, in particular, going wrong in hiring. When we think about where do we apply technology to either identify what skills are required or to match that with candidates, where's the line?
Because I could see the technology perhaps saying here's what we think the hard skills are, if you will. And I use "hard" and "soft" with air quotes. But I'm not sure I see the connection or I haven't seen examples of where the technology itself can make that connect between someone has skill A that is something that's translatable into skill B even though it may not be the same thing.
GISELLE MOTA: Yeah. Well, it does exist. I'm going to answer this with a little analogy before and get you there.
KIMBERLY NEVALA: Perfect.
GISELLE MOTA: Don't think I'm not going to answer the question.
So I was seeing something the other day, and it was a very cool application of AI. And it's basically taking product placement. So when you're watching a show or you're watching a movie and you would normally see a can of soda or you would see a bag of chips and your eye goes to the label and, obviously, it's product placement. Not a new concept.
They're now using green screen capabilities, which is also not a new concept, but they're taking like a templated version of a can, they remove the logo and the label. They remove the logo on the label on the bag of chips. And now, they're reimagining the experience by using AI. So follow me for just a second, because I'll get there.
So when you think about that, nothing is new. But the application of AI is reimagining the experience of marketing and product placement. Because what it does is, let's say it knows that, hey, Giselle's watching and Giselle's watching this particular show. It's tied to Netflix. Netflix already knows my past watching history, my profile. That's why it makes curated recommendations to me.
And now, it's going to take that information and show me-- I don't really drink soda-- it's going to show me something like a sparkling water or something that may be an organic option or it's going to show me something that's a healthy version of a chip or something. Because it knows that that's what I'm going to be looking at, because it understands me as a buyer. So it will no longer be you have to have the physical asset there. It's going to be personalized to the user, and that's how you're taking a normal experience that you would have had before-- sometimes, also very manual and tactile-- and you're making it into a personalized experience powered by AI.
The same concept exist with HR. We've had to reimagine our processes, especially during COVID, especially during the pandemic. So onboarding, for example. Now, if you apply AI to onboarding, you can now personalize the experience to someone that you are onboarding remotely who never had to step foot into your office.
Or maybe they are going to step foot into your office on a hybrid approach. And without them ever having to come and make a physical interaction, based on their data that you picked up from them and what they've inserted, you can say, hey, based on where you live, these are some routes that you might want to take in order to get to the office. Here are some places you might want to have for lunch and some breaks. Because we understood, from your data and your profile of what you put in, here are some people that you should connect to in the organization before you even show up because we know what kind of affinity groups you might be interested in based on how you self-identified in your data or what skills and experience comes on your resume. So all of that are ways in which we can reimagine the experience and tailor it.
And if you think about the application process, you can use AI-powered chat bots to help screen candidates before they even get through a recruiter. And so that's one way that people are using AI and communicating between the-- adding a little human element as well. Because we all know the abyss of when you apply to a job and then you get that little email, "Sorry. You did not meet our qualifications," or whatever the generic things are.
Now, there are AI tools-- and we even use this at ADP-- to help lead the candidate and guide them in their application process of, hey, you might want to consider this role. You should kind of look at this. Questions that you might have. It helps you to actually interact with someone. And if the chat bot is not answering the questions, the candidate will have the opportunity to go directly to a human being. There's so many different ways in which you can use that. I could go on and give you more examples, but I'll pause. I'll pause.
KIMBERLY NEVALA: Well, one of the questions that comes to mind-- for me, at least-- when we talk about using things like artificial intelligence, these systems are good at reinforcing behaviors or replicating actions or outcomes that we have seen before. They're not creative. Right? They don't necessarily come up and invent new pathways or new ways of doing things. We might apply them to do things in a different way. But they're not fundamentally, by themselves, going to say here's a different way.
And so when we look at things like in hiring, for instance, where we might be really looking more for an attitude and an aptitude than explicit skills. More and more today, I think we're seeing that, if we want to bring folks into the fold and break down some of what have been the traditional funnels, I suppose, which have been very homogeneous. They reward the same kinds of backgrounds with exactly the same kinds of sort of skills, whether it's schooling and the path or how fast you've progressed through the path, certain personalities…
Where does AI or technology make sense and where do we really need to think differently in terms of that? What's the line between the human and the machine in this area?
GISELLE MOTA: There's definitely a pass-off. So there's a point in what you're explaining that AI can surface insights, make predictions, make recommendations. It's basically only taking historical data and making decisions and synthesizing that data to either sell you, hey, here's a prediction of something that may happen, the likelihood of what can happen in the future based on historical information.
So to your point, do we really want to put the decision making of hiring a candidate and saying if their skill set matches another's completely on an AI or are we using it as a tool to help sort through the thousands and thousands of applications and resumes that can come through and help you sort through? At the end of the day, the human has to make the decision.
So there are certain tools. We have one called "Candidate Relevancy" over at ADP. What I like about how it is using the skills matching is that it's taking this data, comparing it, and making recommendations. But it's not eliminating the candidate. So it's up to the hiring manager to decide, hey, OK. AI said that this was a low-, medium-, or high-fit candidate. But it's up to me at the end of the day to look at all of them and understand if I want to hire them or not even if it said it was a low match. That's still up to me if I want to keep them. Versus other cases that we've heard and seen. Again, Amazon, there was a case with the recruiting that completely eliminated candidates through the algorithm. With this, there is a need to inspect the algorithm and determine areas of bias.
So if part of the analysis is to recommend that someone is high, medium, or low in ranking because they went to a university that's historically Black college and university or because it was a woman group that the person was associated to, obviously, those are areas of bias that we want to mitigate. And so with our tool that we use, we remove all of those pieces and it's strictly looking at the skills part. Right? What is it that someone is saying from their LinkedIn profile of skills? What shows up on their resume? We're not looking at their address, their name, what schools they went to-- none of that. So that's important.
KIMBERLY NEVALA: Yeah. And there's certainly that example-- I think it was LinkedIn-- where the screening process was shown to be biased against women even though there was no gender identification specific in the resume or information. But in fact, you can sort of use proxy variables for doing that.
GISELLE MOTA: Yes.
KIMBERLY NEVALA: And so, yes, it called out women, but also maybe some other folks that would not traditionally have been hired. And it was sometimes based on, what schools you attended and pronouns but also just based on rate of promotion and things like that.
GISELLE MOTA: That's true.
KIMBERLY NEVALA: So how important is it-- in the situation that you have where it's not eliminating anyone, but certainly, it's intended to optimize the process. Right? So it's intended to say, for the folks that's making hiring decisions, trying to help you sort of prioritize who you look at. How important is it that you have a process-- or is it… I guess I should ask that question differently. Is it important? Someone once said to me fairness lies in the outliers. Right? It lies in the things where someone got marked lower down or got disqualified that you also have a process to interrogate--
GISELLE MOTA: Yes.
KIMBERLY NEVALA: --where those recommendations may be going wrong. And not just looking at here's what went right or what the input data is. But let's really look at and analyze the outputs through all of these different lenses to see if, in fact, something might be going awry that we didn't (anticipate). Do we do that enough? Do we need to be building that into our practice?
GISELLE MOTA: Yes. It's extremely important, and we don't do it enough.
And I actually was on this group for AI leadership. And we were talking and thinking through, how can we start to bring more accountability to AI? So not just the whole concept of people call this like "black box," which even that term needs to be reexamined there. So really, what it means is what we're trying to say is stop hiding or concealing how the algorithm is coming to its final decision and how it's synthesizing data.
So I believe whenever you're using a tool, if you are going to a vendor to use a tool-- let's say it's an AI that's helping you take video recruiting and you're getting someone on camera and that's telling you who you should hire or not, you should ask that vendor how it's coming to that conclusion. How can you ascribe friendliness characteristic to someone because you're seeing them on a camera? And the AI is basically using points on someone's face to determine on other points of faces that it's been fed of data that that's what happiness looks like and so this person is friendly. Because what if an individual had a stroke? What if they had Botox? What if they had anything else happening that you cannot ascribe those certain characteristics?
And like you were saying before, aptitudes, how do you figure out aptitudes on someone when it's not so easily trainable? So there is a point where you have to have the AI have explainability, clear explainability and accountability. How did you come to this conclusion? What data factors were in place? And allow the human, at the end of the day, to make a decision based on all of the information.
Maybe the AI is right. In many cases, it can be. But sometimes, it's flat out wrong as we've seen in many really bad cases of how AI even ascribes, for example, beauty. It has looked at Black faces. As a woman of color myself, I can say that this is important-- that it's looked at Black faces and said that it's ugly or that it's an animal or it's a man if it was really a woman. So we have to be careful how much we really put on the AI to come to our conclusions. There is a pass-off. Let the AI tell you what it came up with, but the decision ultimately has to be the human's.
KIMBERLY NEVALA: There also seems to be some critical thinking and common sense we need to apply, as humans making decisions about what decisions we're letting AI inform or influence based on the information that we have.
So you had that fantastic example of looking at someone's facial expressions or micro-expressions or all of those pieces and maybe asking ourselves, is that actually scientifically valid? Is that really how I want to think about it even if it is-- which it isn't. But if it was, is that really how we want to operate? Or what other factors might have, for instance, influenced someone's poor performance in one situation that won't apply in this new situation?
So I like this idea of, again, being able to look at this very holistically. So it's not a silver bullet. It's also not the end of times either in terms of that.
Now, you did mention bias. And you and I have spoken before. Artificial intelligence in these systems are implicated in exasperating or perpetuating biases and poor practices. But that's really not the only story. You also talk a little bit about how AI can actually be used to unearth or expose those issues. So can you tell us a little bit about that side of the coin?
GISELLE MOTA: Yeah. So we've created this tool at ADP called the "Diversity Dashboard." And basically, it's looking through organizations from hire to retire from all of their practices and understanding, where are your gaps in diversity, equity, and inclusion? So do you not have enough people who are going through the pipeline to be considered for a role, and are they not diverse enough?
But if we take it steps deeper, we could start understanding even behaviors. Who's leaving the organization? Why are they leaving? At what rate are women leaving the organization over men? Why are they leaving? Start surfacing those insights.
Then get really deep. By what department? Is it a departmental issue? Is it by a manager? Because then you need to focus your attention and take some action on that particular manager. Is it a particular location? What is that?
And so I think the potential that AI has in general is to be able to look at what story is your data telling you. And again, it's up to the individual at the end to make decision, to take action, and to drive accountability. So the AI can only go far enough in all it's doing because it's not magic. I'm sorry to break it to anyone who thinks that AI is like this magical thing, but it's not.
It's just literally taking data and enhancing that data, often being able to surface insights that our human eye and our human capacity cannot because of the amount of data. And even the intricacies of the data, like sometimes we cannot piece together a story based on all the information in front of us. But AI helps to do that with algorithms behind the scenes trying to piece together information.
So, yes, it's that. It's being able to understand even areas of diversity. Where do you lack diversity? What are some opportunities? Get deep into the candidate or the employee and understand, if you are having an area of promotions and leadership, by what areas of intersectionality are you missing out on certain people? Not just a woman-and-man conversation. It's not just a Black-and-white conversation. Maybe you're having issues with women of color in general and maybe even some of those that have disabilities. And so we can surface some of that information to help organizations figure out what's really going on under the hood.
KIMBERLY NEVALA: Now, it strikes me that the systems could do two things. One, they might serve up insights you weren't aware of before. And that might be a little bit uncomfortable to look straight on or require a bit of change. And sometimes, the systems are going to be wrong.
GISELLE MOTA: Right.
KIMBERLY NEVALA: So is there a particular mindset organizations or decision makers need to adopt when they think about leveraging these systems or trying to put this type of insight to work?
GISELLE MOTA: Yeah. Absolutely.
And remember Amazon, I gave you the example. I got to go back to them because they're such a case study just in general. So they have this metric, and they were using AI to come up with productivity and performance of their employees.
So remember, now you have employees who are working in these environments alongside robots. They're using iPads. It's like this whole thing. Now, they started to use this metric called "time off task." And what that would do is start to identify, if you're not at your station, if you're not doing your work for a prolonged amount of period of time, it's going to count against you and your performance.
What ended up happening is what we all saw in the headlines. We saw headlines that people were having to handle their natural business in bottles, or we heard all kinds of crazy things that were hitting the headlines. Really, what's happening at the end is that people were feeling like the algorithm or the AI-driven metric on them was making them have lack of health and safety and psychological safety. Maybe some people had disabilities and certain needs that they could not physically always be there that way.
So what ended up happening is that HR had to step in, the organization had to step in. They had to reexamine their policies, reexamine that algorithm approach. And now, they've taken action on it. Right? So much so that they've also now invested in robots that help people function with more ease, so it lifts up heavy items. It takes things off of shelves. It moves something from one end of the warehouse to the other all because of these concerns.
So why I say that? The mentality is, if we try something, we need to have the ability to fall on our sword if it fails and quickly put humans as number one. Number one has to be the human element in your organization. If you tried something for automation and AI and it goes wrong, take responsibility, accountability, act quickly. And if you have to make more of an investment to meet whatever the need is for your people, then do so.
KIMBERLY NEVALA: Centering on the human at the end of the day and coming back to that ability to admit or confront the fact that we might have made a mistake. And then the ability to sort of shift gears and to do that openly, honestly, and quickly, hopefully, in the right cases is really important. But that can be really hard for us as individuals, individual entities, as well as organizations.
GISELLE MOTA: Very true.
KIMBERLY NEVALA: Now, you're talking a little bit there also about some of the different intersectionalities and these components. How should organizations be thinking and approaching everything AI or everything, full stop, from an inclusive lens?
GISELLE MOTA: I think the answer is in the question. It is to approach it with an inclusive lens.
So first of all, I would say take a look at your processes today and see where you're lacking without even putting AI into the mix. Just start on the basic level of, do we have everyone on our leadership team? Or the people who are working on our products, do they all look the same? Do they all come from the same backgrounds? Because if you do, you're going to come up with confirmation bias.
Katica Roy was recently talking about pay equity. And she was saying how, even performance-wise-- historically speaking-- even if a woman, does the same-- she's on par with her performance and her productivity and what she's doing on par with a man, still performance is always docked lower. Still, pay is always docked lower. So if we start taking that historical data and apply AI to try to fix a problem without fixing some of the data that's going in to begin with, then we're going to come up with a confirmation bias at the end of the day. Right? Bad data in, bad results out.
So I think that it starts with a human analysis. Start there.
Then start saying, where can we solve this problem? So if it is a problem with bias, then start formulating your AI to detect the bias. So let the algorithm look for, whenever a threshold, whenever we're seeing that there's too many women or even too many men over women or women over men or a certain demographic of people over the other, we need to flag this. We need to look for this and determine like, hey, let's take a look at this. Let's send a notification to a manager or a team.
Or if it is coming up with a recommendation-- let's say, again, we were talking about a candidate. So if you know that your organization has a lack of diversity, yet I applied to your position and I may have ranked as a low, that algorithm should also now have the added buffer in there that, hey, Giselle ranks as low, but she's a diverse candidate. You should consider her and see if there's actually a path to get her where you're trying to go.
I'm going to put all these conversation points together. So for example, if she comes with the direct skill set that you need for that role, it should then recommend she is a low fit, but she is diverse and she has skills that are adjacent to where you're trying to get to. All she needs are these certain courses, to get with this certain mentorship. We need that level of recommendation, and that's what I think is missing. It's robust, but it can be done and that's what I think is the opportunity and the key that's missing there.
KIMBERLY NEVALA: Yeah. Mapping out that human journey. I love that.
So as we talk about all of these things, clearly there's a mind shift that's required. Whether you're a decision maker, somebody trying to think about where do I put AI to work, or whether you are somebody who's being asked to work with AI and incorporate (it into your work).
How do I know, as someone as a hiring manager, when I should and should not or when I am and am not allowed to take the input from this system as sort of the solid gold truth, or how should I be weighting this? Is this input? Is it not? What's my agency in the process? Critical thinking all the way down to folks at the level of developing these systems.
Does the data we have actually reflect the future we want? In which case, if it does not, we may not be able to create a data-driven system that's projecting the past to create that sort of new future. So what are the practices or pillars, if you will, that organizations should think about when they're thinking about both cultivating and training talent in this age of AI?
GISELLE MOTA: So first, the talent that's actually working on these tools and solutions that are AI-driven, I think that, if you hire someone that's just concerned about getting something done because it's cool and because it's neat and we can do this, I think there needs to be a little bit of a reality check when it comes to when you're applying these to human decisions and things that affect humans, like in HR in the workplace. You should not just apply AI to everything just because you can. There should be a real problem that you're trying to solve, and it should be in the interest of the human.
Then you go back and you apply AI. Don't just do it. Because like I said earlier, like, hey, somebody probably came up with the idea of how cool would it be if we can interview people on video and then apply AI to determine levels of aptitude and personal characteristics? Unfortunately, that's--
KIMBERLY NEVALA: Yikes.
GISELLE MOTA: --not the best way. Yeah, A little cringy. Right? So unfortunately, that's not the best way.
And we have seen that because it's been damaging and it's been biased. And now, we know that that's actually not the best-use case. So I think it's, one, when you hire someone that's going to be working on these tools, make sure that they have a problem-solving and a human-centric problem-solving approach. That's one.
The second is diversify the people that you're hiring. If everyone is the same, they're all going to come up with the same conclusions. And I don't just talk about race and ethnicity and gender, but I'm talking about age, lived experience, tenure. Diversify that thing because you need to have as diverse as possible.
Why? Again, to approach the diversity of humanity. There's such a diversity in humanity. And in order to make decisions, you have to have many inputs. For example, autonomous driving. You cannot apply autonomous driving that you developed in Europe to say that that's what's going to work in the Middle East. Because some of the decisions that the vehicle needs to take, whether it values age, if it needs to hit the brakes, are you going to go over the person who's older or save the person who is younger? Yes. This is a real thing. These are real considerations.
KIMBERLY NEVALA: They are. They are.
GISELLE MOTA: So if you apply certain ways of being in cultural aspects that worked in the UK but they don't work in the Middle East, then you're just going to create safety issues and a lot of issues when it comes to that. So what I mean is you must apply diversity of thought, but there has to be localization, too. So to apply a scientific method, apply logic.
And what problem are we trying to solve? To whom are we trying to solve this problem? Who are the people, the persona that we're trying to reach out to? So there's just a lot of consideration. And in general, if I answered that, is that. We need to have consideration when we're doing this. Yeah.
KIMBERLY NEVALA: More thinking.
GISELLE MOTA: More thinking.
KIMBERLY NEVALA: If I'm going to oversimplify this by 1,000, I would just say more thinking.
GISELLE MOTA: Yes.
KIMBERLY NEVALA: More people thinking--
GISELLE MOTA: There you go.
KIMBERLY NEVALA: --about these ways and in other ways. Now, this is a breath of fresh air, by the way. I love this.
GISELLE MOTA: Awesome.
KIMBERLY NEVALA: So you're really thinking and looking forward and seeing how people are applying this. And we're going to have you come back and we'll talk about adaptive learning because I know that's a key passion area for you as well.
But what are you looking most forward to seeing kind of come to pass over the next couple years as we apply AI in these areas?
GISELLE MOTA: I'm hoping to see AI start to be more accountable for itself and to be able to detect issues in itself. I think we started to see the development of what's called "auto machine learning" - "AutoML" - and where it's able to kind of work on itself and notice where there's an issue and then solve for it automatically. I'm looking for that and hoping for that internal to every algorithm that's applied, so to detect bias and to fix it automatically. And in that fixing and in that automation, I'm hoping to see more accountability. I would love to see a report that comes out.
For example, now a big deal is biometrics. And we're taking people's health information. Because of the pandemic, we're storing their physical assets that they have, whether they've gotten vaccinated or not. These are all issues that require data privacy. I would like to see if we're using these contact tracing methods, if we're using biometrics-- all these things-- you should show an employee at the end of the year, what did you do with that data? How was it stored? Who had access to it? How many times did they have access to it? I would like to see some sort of accountability report that we normalize to those who we're using their data from. So that's something else I'd like to see.
KIMBERLY NEVALA: That's fantastic. And again, because - for some reason this morning I'm going straight to short taglines - I was thinking, well, we need that self-help for AI, really.
GISELLE MOTA: That's right.
KIMBERLY NEVALA: So how about for those of us humans out here looking to try to develop and that will be intersecting with these solutions? What advice would you give us?
GISELLE MOTA: Ask questions. I think this is a prime time for a mind shift.
I think, as a society-- as a global society-- the pandemic made us all slow down and start to really ask. And not just the pandemic but some of the ramifications of the pandemic. So we saw a lot of racial and social injustice globally. We saw a move of people getting fed up with certain things and wanting to spend more quality time, whether it's taking care of their mental health, spending more time with their family, getting out into nature.
I feel like there's been this like global mental shift that's occurred. And I think we can start taking advantage of that, and let that continue. Let's not lose the momentum. Ask, people. Ask your vendors in your organization. How are they keeping accountable and having ethics baked and involved in their AI processes that they're using?
If something doesn't exist today and you think that you have a solution, raise your hand and say, I think we could apply either a human practice to this or we can leverage technology to address this certain problem or issue. But I just say that. Let's put the human at the center, and let's all start uniting and raising our voices around certain solutions.
KIMBERLY NEVALA: Well, if anyone can be said to model the behavior that they are talking about and preaching, it is certainly you.
GISELLE MOTA: Oh, thank you.
KIMBERLY NEVALA: I love the curiosity, the insights, and your ability to help us think about how we can really put AI to work for the benefit of all. So thank you so much, Giselle.
GISELLE MOTA: Oh, thank you, Kimberly. My pleasure.
KIMBERLY NEVALA: All right. So next up, our second season is going to conclude with Kate O'Neill. She's known as the tech humanist and is the author of the recent book A Future So Bright.
Kate is going to discuss how we can lean into the future of AI with optimism rather than being either overconfident or overwrought. Subscribe now to Pondering AI so you don't miss it.
[MUSIC PLAYING]
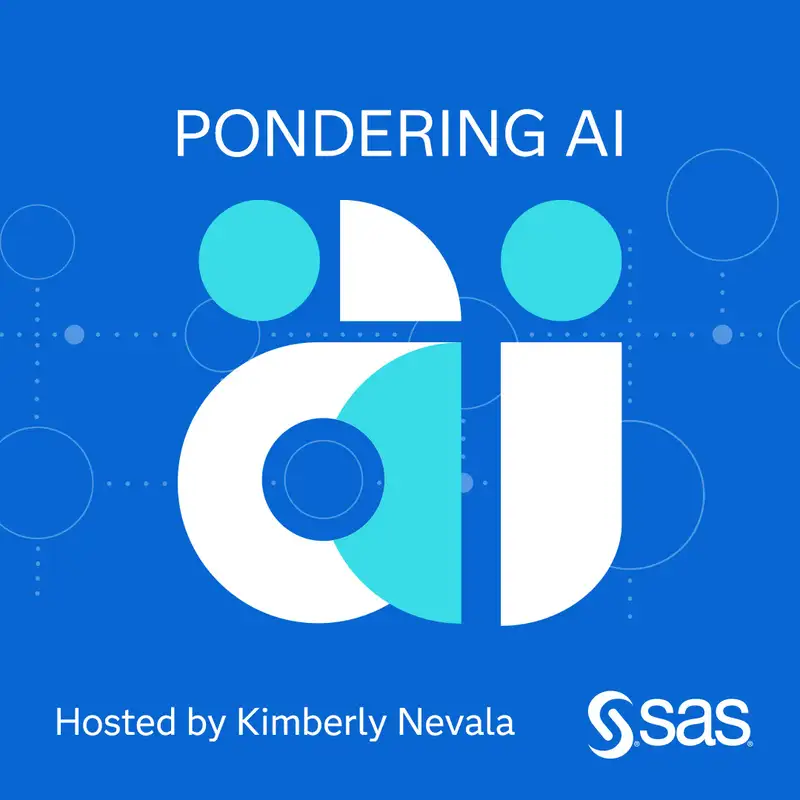