Is AI-Driven Sustainability Sustainable with Vincent de Montalivet
[INTRO MUSIC PLAYING]
KIMBERLY NEVALA: Welcome to Pondering AI. My name is Kimberly Nevala. I'm a Strategic Advisor here at SAS, and I'm so honored to be hosting our second season, in which we talk to a diverse group of thinkers, doers, and activists all working to ensure our AI-enabled future puts people and the environment first.
Today, I'm so happy to bring you Vincent de Montalivet. Vincent leads Capgemini's sustainable AI practice, and he will help us understand the yin and the yang of AI's relationship with our environment. Thank you for joining us, Vincent.
VINCENT DE MONTALIVET: Thank you for the invitation. Very pleased to be here.
KIMBERLY NEVALA: Now, I'm finding that everyone at the cutting edge of AI has really interesting multidimensional backgrounds, and you are no exception. Will you share a bit about how you came to be a practitioner of both engineering and marketing?
VINCENT DE MONTALIVET: Yeah. Actually, I studied as a Global Engineer/General Engineer. I studied supply chain at the very beginning of my career for two years. And maybe you know, but when you start diploma, you have to study this very beginning of the AI - what we call Operational Research Model - with Monte Carlo simulation and stuff like that. So it was a couple of years ago, to be honest, and the terminology AI itself was not really part of the studying there. But then, from time to time, on my career I switch from - I would say, the business analyst part - leveraging data, leveraging global digital technology, just to work on a better future.
So it was either on the circular economy or also for monitoring CSR within industries. So I had a couple of jobs that are really, I will say, at the crossroad of sustainability and IT and data, especially. So I'm quite honored to join Capgemini two years ago - as you said - to lead a global offer on sustainability. Data, AI, and analytics, to be more precise.
KIMBERLY NEVALA: So coincidentally, I am also an engineer by training. I'm a Chemical Engineer. So I always tell people that my first coding language was Fortran, which now dates me. But -
VINCENT DE MONTALIVET: Yeah. It's a good one.
KIMBERLY NEVALA: [LAUGHING] Tell us a more: what does your current role at Capgemini as a Sustainability Lead entail?
VINCENT DE MONTALIVET: So basically, my role is to help our client to leverage data, AI, and analytics for achieving their sustainable goals. So it is really crucial for Capgemini to now have a dedicated
sustainability program, and that is really part of it. We call it also Data for Net Zero Strategy Program, internally. So it's a worldwide program.
KIMBERLY NEVALA: Fascinating. Now, there is certainly a passionate debate about AI's influence - both good and bad - on the environment going on right now, and you're at the center of that discussion.
So we're going to talk about both aspects, but let's start with the good. AI certainly is already playing a role in helping us both improve our conservation efforts and protecting the planet's ecosystem. What are some of the most innovative and/or important applications you're seeing today?
VINCENT DE MONTALIVET: What we can currently see, is AI is currently leveraging for better understanding our environment, better monitoring our impact, I must say.
We are still in a time where we are doing our simulation based on some mathematical layer - some simulation layer - and AI and data has a good momentum that can bring real time measurement. So for example, we built a program for the European station that had the ambition to monitor the real time CO2 emission around the globe. So to be honest, maybe there is only a few people that are aware of this news. Because we are talking about climate change all the time, but we don't really have yet the capability to monitor real time the CO2 emission around the world.
So this is an example that what we are building right now, the emission is Micro Grab - something like that - you can have a look on the internet. So this is our first achievement, which is switching from simulation and simulating modeling - thanks to the mathematical layer - to a reality of measurement. And AI can bring a lot of better understanding, some specific measurement coming from satellites, radar, and stuff like that. I won't go into detail in the technical step.
And maybe the second example I can also share with you is regarding the environment itself. What we encounter right now when we are engaging in the discussion with the client is, mostly AI is here to, let's say do a job that a human can't do. Because there is also in the debate not only whether or not AI is good for our planet, but also whether AI will take our job. So I need to say a word regarding the fact that in this particular project - which is on the forest management project - basically, the AI here is there to monitor how the forest is sick or not. And the human that was there before, just to make sure there is no problem in a big forest, he did not have the time to go everywhere in the forest to see whether or not there is some sickness. So AI, leveraging satellite images, can then alert this specific guard to say, you need to go there, have a look. Because it could be a good starting point to look at these trees, to make sure we have our forests in good health. So this is another example that I can share with you, which is no more on the measurement itself, but much more on the monitoring and alerting system.
KIMBERLY NEVALA: I love that example because it's a good illustration of us thinking about AI not as an entity in and of itself, not as something that's coming at us autonomously, but as a tool for us. Something to extend our capabilities, and something that we can shape to really use to our advantage.
VINCENT DE MONTALIVET: It's crucial, yes.
KIMBERLY NEVALA: That's a fantastic one. Now, I saw a report recently - and I think it may in fact, have been on Capgemini's site - that was talking about AI's role in corporate sustainability. And you talked about zero net sustainability. And I know you also are very much tied into the UN sustainability development goals, many of which are looking at industrial type applications.
I believe I saw that AI has already helped reduce greenhouse gases for manufacturing - for instance, by 14%. And there was a projection that it could potentially help retailers - I think the end date was 2030 - reduce their carbon emissions by 40%.
Will you talk a little bit about what you're seeing - in terms of applications and trends - in that realm? In terms of industrial and corporate or business applications?
VINCENT DE MONTALIVET: Yes, sure. Again, to give you a rough idea of our current discussion with our clients here, we are leveraging data AI analytics to do mainly one thing for our clients. They want to switch from a reporting logic, like each year how much do I emit? How much - regarding all CSR KPI I put on my CSR report that nobody wants to read, because it's unreadable - to something that is more applicable on each layer of the value chain.
So it's been for, as an example, for the chief procurement officer what will be a new way to integrate sustainability as a currency and the carbon as a cost when I'm doing my trade off on whether or not I will choose this supplier or this supplier. Instead of just calculating an analytic based on TCO or total cost of ownership, I need now to integrate the carbon cost. Because I am committed to net zero, I need some new tools, some new analytics, some new data, to make this kind of trade along the value chain.
So this is something that are coming very strongly across all industries. The demand is very high, and this is mainly the reason why they want to switch for this reporting to - let's say - an acting perspective. Because otherwise you can't act, right?
We are currently in the decade of action. So it's good to commit - but now, we need to switch from commitment to action. So this is where, again, AI data analytics is playing a huge role. It's not the silver bullet, but at least it can help the CXO to better manage the operation.
Another example, I had a discussion a week ago with a big emitter in terms of CO2. It's a cement industry so it's awful the way they are emitting gas house emission and especially carbon. They don't want to rely anymore, again, on the simulation. They want to put dedicated sensors on top of their plant to see how much is the CO2 emitted in real time. And then, do some predictive analytic, or do some - at least recommendation - to change the way the operator are using the machine to build this one ton of cement, to decrease the amount of carbon emission regarding this one ton produced.
And at the end, what will happen is that this kind of corporation will be able to share not only a price at the end of the process of manufacturing this kind of cement, but also a carbon footprint. So depending on the way I manufacture my product, I will be then able to share a carbon cost on each of my prototypes.
This is the reality also of the demands. Because we are - as a customer - want to act also as a responsible consumer. And we want to choose also to some products that are no more carbon heavy. So this is the trends we can see currently in the manufacturing industry, and in overall, the corporate industry.
KIMBERLY NEVALA: That is such a great trend. And I love hearing that, because I think in a lot of cases when we're talking with folks - and what I hear and what I see when I'm reading - is certainly a lot of good intent. But a lot of individuals - maybe it's that manager of that plant and the engineers on the floor - would like to be able to support and drive these initiatives. But, they haven't had the tools. And frankly, haven't been given the authority or the responsibility to be able to act on those and make decisions that actually would make a difference today. As opposed to looking back and projecting based on that. And in that case, we're always projecting the past onto the future and trying to catch up.
So this idea that we're now sharing responsibility and driving that down to the folks who can make different decisions on a day-to-day basis is really exciting. It struck me, as you were talking about the supply chain, though, that certainly there's a number of challenges, potentially. We talk, even in the age of COVID, about folks trying to get visibility into their complete supply chain, which has been a challenge.
How do you coordinate all of the stakeholders? Because this is not just the manufacturer or the retailer. It's them and all those partners, the other vendors and suppliers in their supply chain. What is the mechanism that folks are using to get everyone involved? So that you in fact do get the information, and that complete picture.
VINCENT DE MONTALIVET: That's a good question. To be honest, it's a long journey. Definitely there is no silver bullet. In terms of architecture, we are releasing -I think we released a month ago - a new report (if you have the time, have a look on it) on that ecosystem.
Which is, I think, really the first foundation to gather all this data that you mentioned along the supply of the supplier and the suppliers to supplier. We had also a couple of projects where blockchain could be an enabler to build that trust with all these actors along the supply chain.
But for now how it is, it is mainly at the first level. And then, we ask the supplier - as far as I can see within the data system - also to address this kind of KPIs or this kind of question to level two of the supplier. And this is the way it is right now.
There is this data ecosystem that needs to be addressed, as a proper value chain. So what could be taken out of this data ecosystem? I'll give you an example. With only retailer and producer, there could be a lot of data that could be exchanged, that produce more value, and also for the sustainability.
For instance, the retailer do not have yet access to the real carbon footprint of the product. The producer can share that information with to the retailer. And the retailer can share, in exchange, for example, the reality of the sales. And some data regarding improving the sales, regarding whether or not - because these products are more carbon heavy - it's not sold anymore and stuff like that.
So we can see this as an opportunity for our client also to breed some some data ecosystem. But there is no typical silver bullet on that question. It's still something that we need to tackle, I must say.
KIMBERLY NEVALA: Well, I'm sure you've heard this before, as well: it's often said that we as organizations today are already data rich and information poor. And what I hear you saying is there's a lot of data we're not using and sharing and integrating today that can allow us to make a lot of progress.
So progress over perfection. And it doesn't sound like there's any barrier right now for us to make really germane improvements today, using the information we have.
VINCENT DE MONTALIVET: Yep.
KIMBERLY NEVALA: Now, we would be highly remiss if we didn't shift our focus to the other side of this argument. And namely, a lot of discussion about, that yes, AI may be able to support a lot of these sustainability initiatives, but perhaps AI requires its own sustainability initiatives.
So let's talk a little bit, and cut through that discussion, and get down to the basics here. You've observed that AI - like any other technology - will consume energy. And not everyone's finding this a very compelling argument, however.
So let's just ask the question, just how inefficient are AI algorithms?
VINCENT DE MONTALIVET: I think where we are, as an industry within the IT industry, still a mature industry that want to think about a way to build some more eco responsibility, or efficiency, or whatever we name it. But there is still some progress that needs to be done at the research level.
So just to give you an example, on one hand, it has been proved that the more data we have the more accurate is the model. So this is something that's been proven, so there is no solution here. Instead, finding a new way to implement machine learning and this is currently some research paper that are still on the progress. This is a fact.
But it doesn't mean that for all use cases you will need big data, you will need AI to solve your problem. So this is something where we can make a particular step. Say, OK, look another example where you can do some warehouse optimization leveraging simple models by implementing some clustering methods. By implementing some regression models that don't need to be trained days or weeks or whatever.
So then, on the other end, we can see that on the press we've seen some press release on the GPT-3 that consume - how many, I don't remember, 250…
KIMBERLY NEVALA: It was an eye-opening stat. It was something like every training run emits as much carbon emissions as five cars emits in a year, right? I think it was something like that.
VINCENT DE MONTALIVET: Yes, exactly. Five cars or five New York-Paris… - so I don't remember how big it was. But -
KIMBERLY NEVALA: And not electric cars.
VINCENT DE MONTALIVET: Yeah, yeah. We need to keep in mind that this carbon model need to be trained only once. After this model - most of them are released as an open source - to then use it as it is pre-trained, with all the weight, but of all this neural layer that can be reused almost as it is. And you can do what we call transfer learning technique, to then find the results you want to find when you implement such an upgrade.
So it is important to say that these figures are not the one that is used when we use these kind of models. And to be honest, GPT-3, nobody - there is just a few of cooperation that use this kind of modeling.
Currently, AI - to give you another example: AI at scale today - it's only 15% in the industry. So there is still a lack of AI maturity at scale worldwide in the corporation. And for the GPT-3, almost nobody and no corporation are using this kind of model. The main model that we are using - for example, when we are implementing some forecasting modeling, some - I don't know what kind of example - even the computer vision that seems to be very heavy in terms of energy consumed. With this example, with a dedicated client, we're trying to figure out how much we used in terms of train to retrain this model. Because it was for actually capture some egg and the state of the art of the pre-trained model that we could reuse wasn't trained to recognize egg.
VINCENT DE MONTALIVET: It was trained to recognize cats, human, plane, trees -
KIMBERLY NEVALA: Right. [LAUGHING]
VINCENT DE MONTALIVET: - or whatever, right?
But egg, it's quite different. So we had to retrain this model to make sure it recognize egg. And to do so we need 500 hours on single computers. So we then calculate how much emission, in terms of CO2 footprint, that would present.
And at the end, by implementing such an AI within this industry, it was to improve the quality control. We realized that after 10 minutes of production, it compensates the number of hours that was needed to retrain this model.
So I think we may have this in mind, when we are producing an AI, to at least calculate our emission, report on our emission, see whether or not there is no simplified way to at least give a result that could be OK for the client. No need to go directly on machine learning if it's not the need of the client. So go on the business need, instead of the technical stack, and see how we can help this client. Not only by leveraging machine learning only, but some of the technique in AI. That could be some constraint programming also that can be leveraged, and no need to be trained.
So yeah, AI is a very big topic, and vast topic, and there is a lot of technology under the umbrella of AI that is not at all a huge constriction, I would say.
KIMBERLY NEVALA: Two other, I'm going to say, models - although I don't necessarily mean those in the AI sense - that I've heard people talking about, as well. One, that we should start to project forward, and look at all of the good - where can we actually support conservation - subtract all of the bad, in terms of the energy and other effects. And then we sort of come to a judgment: is AI ultimately good or bad? That strikes me as maybe not the best way to think about this. Not the least of which is due to the fact that I think it's very difficult to put this genie back into the bottle.
But what you just said is that we probably can't do that on a smaller and an incremental basis. The same way we're thinking about how do we make our business operations writ large have a net zero impact, we can be looking at our business processes which use AI and making those net zero. And that calculation of what the analytic model is using and generating should be part of that.
The other argument I've heard is that, yes, but maybe it's 15% today, but it's increasing exponentially. But what I hear you also saying - and tell me if I've gotten this right is that increased - linear or even exponential - growth in things like artificial intelligence are not necessarily going to be matched by a linear or exponential growth in their carbon footprint.
VINCENT DE MONTALIVET: Exactly. I released - on one of my blog posts - a link that gives some figures regarding if we look at our 10 years back, the growth of data is incredible, we all know that. But nevertheless, the growth of the energy that are supplying our data centers, are flat - are almost flat. So you can see these curves are not in AI whole. So we are currently doing some progress also on the energy efficiency of our data center.
I'm not saying here there is no more progress to be done and stuff like that, I'm just trying to share some figures and that still we need even to do more, and more, and more to progress on that perspective. But if you think about some global figures, 4% is due to the IT. AI itself within this 4%, will be something like 0.0.5, maybe, or it's - I guess, I don't know - but it's almost nothing regarding this, to that percent.
It is true that there is a curve and growth, but as I said, if we rely on the past data that we have currently, we can see that even if the data is getting bigger and bigger, the energy that has been provided to this data is flat. The energy domain was flat.
KIMBERLY NEVALA: Excellent. So I want to turn the page a little bit. You do work and advise with a lot of organizations worldwide. Is there a playbook, or key mindsets, or best practices you advise those companies to adopt, in thinking about applying AI, or adopting AI for their own sustainable initiatives?
VINCENT DE MONTALIVET: Maybe to start with AI I recommend to start with data. AI is kind of a sex word, and we want to implement AI for attacking climate change. But at the very beginning, it's about data.
And so to start the journey - and we can see that the clients are moving on that way - it's fair to implement what we call some data governance missions to be able to identify regarding what you want to measure, regarding what you are committed. You're committed to net zero, so it's about carbon. Where are you carbon emitting along the value chain, where are your data, what are the systems that can provide this data on it, do we need to go external, internal? So it's really, a dedicated data domain on sustainability that need to be built, I might say.
So that's my first recommendation. To implement a proper data strategy for the sustainability, and a proper data domain for climate, for biodiversity, for inclusive diversity for green investment, from green procurement.
Or along, again, the value chains, you can have in mind the CXO, procurement, supply chain. So you will need some KPIs on the transport - reduction emission of the transport for the operation - the emission of your plant, the procurement, the green material included in your materials, and so on. So everything is about data, so you need to have this data cataloged in front of you, and see where are your sources, and then build your analytics.
And then, on top of that, you have this - after building the sustainable analytics along the CXO, you have this AI that could play a role, where you can leverage this data. For example, to do some predictive analytics. For example, to identify patterns where - let's take an example, otherwise, it's maybe too theoretical. If I come back to the supply chain, one of the most emitter let's say, a role within the supply chain, is the airfreight - airfreight consuming a lot of CO2. Instead of doing airfreight, we can do some rails, some cars, or whatever - some boats. But we can see there is here some different possibilities that we can choose. If you are, let's say an example, a car manufacturer.
And here, AI can identify why airfreight has been put as a solution instead of other low-cost solution, low carbon solution, and you've identified the patterns to then avoid this kind of event to be reproduced. And then, reduce the carbon emission due to using this airfreight. So that's another example.
So but again, first, you need to identify regarding your analytics, monitor what are the hot spots, and then, leverage AI to reduce where are your hot spots. So it's maybe in three steps, that's our analytics and AI.
KIMBERLY NEVALA: That makes great sense. And we've been having a lot of discussions about the need for diversity in AI. Around all aspects of this we talk a lot about bringing in the social science, and behavioral science, and ethicists.
And what struck me as you were talking is that we also, in areas like this - like sustainability - if we aren't going to be serious about that, we need to bring in the domain expertise for this, as well. As you said, it's its own data domain, it's its own subject matter expertise, how you think about it, how you piece that together.
So that's a great tidbit to leave our audience with. Now, you are speaking with customers and clients, and up to speed on all of the latest advancements here. What do you think will be the most consequential advancements in sustainability with AI over the next 5 to 10 years?
VINCENT DE MONTALIVET: It depends. Is it on the green AI aspect or the AI for sustainability aspect?
KIMBERLY NEVALA: Yes, please. [LAUGHING]
VINCENT DE MONTALIVET: [LAUGHING] Both. I think we can definitely find a way to implement AI more efficiently, in terms of - to be honest, maybe something like avoid this massive data to have some accurate modeling. This is something I hope we will be able to achieve in a couple of years. So this is the research part, where we mentioned earlier on the discussion, called the Green AI Aspect.
We can also think about AI in a more decentralized way, by leveraging edge computing, and stuff like that. Which I think also reduce the number of data that is sent across all the systems instead of having something centralized. Maybe think about an architecture that we call Data Mesh. So where the data are within the Department, the data owner decentralized where the business are, the data owner are, instead of just putting everything in a data lake, and then, reporting in the business department. So we need to think about some more maybe green modeling architecture way of thinking, when it is about data and in AI. It is something that is coming, it is not something as an idea, it is something that's currently coming out on this green aspect.
And regarding the AI for sustainability, I think we are not yet there. If you read the report, you may also have seen that in addition to the contribution of AI to reduce the greenhouse gas emission, there is also one little number somewhere in the report saying there is only 3% of the organizations that have implement AI at scale for all the climate AI use cases.
So for me, when I saw this number, I said OK, there is still 97% that the organization did not leverage AI, in order to tackle the CSR challenge - the climate change challenge - within the organization.
So for me, I'm not here saying, in 10 years AI will be capable of doing this silver bullet of climate change. I'm not quite confident about this theory, but I'm more confident on, in 10 years hopefully, 80% of the organization will have the capability to implement the AI scale, to reduce the carbon footprint, thanks to data AI and analytic.
KIMBERLY NEVALA: I think that's a fantastic challenge and goal for us to leave everyone with. So Vincent, thank you so much for helping us really understand that dichotomy between AI's appetite for energy, and its positive contribution to our environment and environmental sustainability. Once again, I think we've learned there are no cut and dry answers when it comes to AI.
[MUSIC PLAYING]
VINCENT DE MONTALIVET: Definitely. Thank you very much, Kimberly.
KIMBERLY NEVALA: Awesome. Next up, the discussion is going to continue with Baroness Kidron. She is the chair of The Five Rights Foundation, which is leading the fight to protect children's rights and wellbeing in the digital age. Subscribe now to Pondering AI, so you don't miss it.
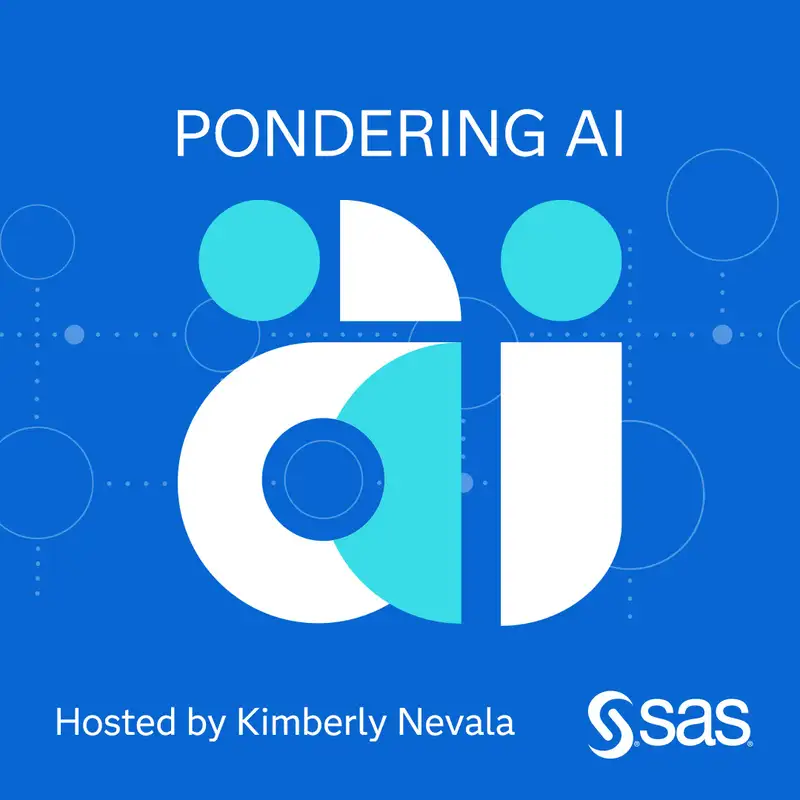