Regulating Addictive AI with Robert Mahari
KIMBERLY NEVALA: Welcome to Pondering AI. I'm your host, Kimberly Nevala.
In this episode, I am pleased to be joined by Robert Mahari. Robert is a Doctor of Law and philosophy, so a JD and PhD, researcher at the Harvard Law School and MIT Media Lab. We're going to be talking about the complexities of regulating addictive intelligence, as well as considerations for shoring up what is becoming a rapid degradation of the data commons. So welcome to the show, Robert.
ROBERT MAHARI: Great to be here, Kimberly. I'm excited to talk to you.
KIMBERLY NEVALA: I'm always a little bit excited when I find someone else who also did their undergraduate studies in chemical engineering, of all places, and then took the turn into all things tech. So tell us a little bit about your journey. How did you go, A, from chemical engineering into law? And then what was the journey to your specific interests today at that intersection of media and law?
ROBERT MAHARI: Yeah, so a little bit of a circuitous path, I guess. I grew up in Switzerland. I came to the US to do my undergrad in chemical engineering. I was very gung-ho about chemistry. And then I figured, well, I don't want to spend all day in a lab, so maybe I'll do chemical engineering. That's a little bit more applied. And then I started studying chemical engineering, which was great, by the way, at MIT. And MIT let me cross-register to Harvard Law School.
And so sophomore year, I took corporate law and thought that was really cool and saw this really interesting kind of intersection between technology, law, and business. Frankly speaking, I think the plan was maybe I'll be a corporate attorney who's focused on this intersection or something like that. So I took the LSAT, applied to law school, went back to Switzerland for a year to work in investment banking and then private equity.
And I think that in that time, I had the pleasure of interacting with lots of lawyers, and it became kind of abundantly clear that law was not leveraging technology in any meaningful kind of way. And so I ended up reaching out to a professor at MIT, at the Media Lab, Sandy Pentland, who runs the Human Dynamics group there. And he had written some stuff about being interested in computational law, using AI and algorithms to extend the practice of law itself. And I said I'll be up the road at Harvard; maybe I can pop by. And he said, you're more than welcome to. And so we ended up collaborating through my first year of law school and realized that there was this really interesting intersection.
We ended up petitioning MIT and Harvard to create this new joint degree. There was this ad hoc joint degree that I could apply for. And the focus of that program was really - insofar as there's an intersection between law and technology - you kind of have the law of technology. And lots of people are focused on that. I didn't feel like I was going to add that much new stuff there. But then there's the technology of law; like building tools for lawyers. And that's really where I focused.
Then the whole AI large language model boom happened towards the end of my PhD, and I felt like there was more room for someone who had a more technical focus to contribute in meaningful ways on the AI regulation side of things. So I kind of returned to that a little bit, wrote a couple of pieces on regulation by design. This idea of embedding regulatory objectives and safeguards into actual technology designs.
And the way I came to write this piece is I was talking to my friend Pat, who's a co-author, also a PhD student at MIT and I mentioned to him that it felt that one of the key risks of AI that it seems like no one's really talking about is its impact on us as humans and especially on human relationships. So we ended up writing a piece together in the MIT Tech Review on this idea of addictive intelligence and AI companionship.
I think this space, too, has kind of become something that more and more people are talking about. And as we'll talk about, I'm sure, in the next minutes, it's something that I think is really challenging to regulate.
So it's this risk that I think ought to be taken quite seriously. But at the same time, the piece that we wrote together didn't really offer concrete solutions. And I don't think that we'll come away from this conversation with really concrete solutions. It's something where I think more research is needed. But yeah, I'll leave that as an introduction and excited to dive.
KIMBERLY NEVALA: If we come away with some more concrete questions, I will consider our work here to be not done but perhaps beginning. So yeah, let's talk a little bit about the concept of addictive intelligence. And for all of those who are really hoping we would talk about computational law and the use of AI and systems in law, perhaps we'll do a part two on that one. But when we talk about addictive intelligence, what do you actually mean by that? How do you define addictive intelligence?
ROBERT MAHARI: Yeah, so even - this is not my field - and so obviously I started looking at literature about defining addiction. And even the definition of addiction is highly debated. It doesn't seem like there's the one kind of clear definition. So I'll sketch the concern and then I'll try to come up with a definition.
The concern is really that you have AI systems that can generate content that is hyperpersonalized, essentially in real time, right? You don't have to wait for it. And that will create a type of entertainment product, I guess, that could ultimately jeopardize, for some people, their ability to relate to other people. That this is just so engaging that it's hard to walk away from. And it feels like the dose here matters a lot.
So if you look at the definitions of addiction, it's usually something about a recurring behavior that has negative consequences. So you have to have both.
It's relatively easy, I think, to see how you could get recurring behavior here. That you have something that you can interact with that can generate text, certainly, images certainly, and eventually maybe video content as well that's hyper personalized. That kind of makes the current types of social media that are already, I think, quite optimized for engagement and quite effective at maximizing engagement look really kind of simple because they're still bottlenecked by human content. Or at least look really unconcerning I guess. So we see how the recurring behavior might happen.
Then there's a question of harms. Like does that behavior actually result in any harms? And I think the harms are a little bit harder to think about. So obviously, there's the wasting of time. That maybe is a harm. And if you look at some of the research that's both been done for social media and that's emerging for AI companionship, you'll often hear people report that they're spending more time on these platforms than they'd like to. So maybe that in and of itself is a harm.
The second harm that we worry about is the kind of degradation of your ability to relate to other folks. So if you think about what a relationship is - whether that's a romantic relationship or a friend relationship or a parental relationship - there's this inherent giving and taking. We take something from -- we have people support us. We have people entertain us. But we are also kind of expected to return the favor.
And the interesting thing about an AI companion is that you could imagine a type of relationship that only involves taking. Where at no point do you have to sacrifice anything. At no point do you have to question whether you're acting as a good friend or as a good partner or anything like that. And the question is whether that can become an overly appealing type of relationship and ultimately undermine the types of skills that you need to relate to others.
Then the third harm is a little bit more nebulous, but it relates to human dignity. And there is this kind of scary world that isn't, I think, that hard to imagine, where you see people living out their days in these AI-created realities. And you could imagine some situations, for people who really have nowhere else to turn, where this is of net benefit. But it seems to me that for most people, this is just kind of a very sad existence, an existence that I wouldn't wish on most people. And so in this very abstract sense, there's this question about the dignity.
And there's also a consent piece here that we can explore if you want, which is that if you think about how these AI systems have been trained, they've consumed the entirety of human knowledge and content. They can figure out patterns in your behavior to optimize the content that they produce to be optimally engaging. And it's not clear if there isn't, like, a massive power imbalance between the AI tools and their developers and what they know about us and us as users and whether you can really meaningfully consent to using these tools.
And if the answer to that is no, then I think that makes the regulatory piece all the much harder. Because if you look at things like the General Data Protection Regulation in Europe, consent is really a key premise, and this is a privacy regulation. But consent is really a thing that the regulation highlights as a basis for legitimate data collection and processing.
If consent is no longer meaningful in an AI world, we need to find some other kinds of regulatory tools. And that makes it especially tricky when we're talking about companionship because that's something where generally we don't really want the government meddling. And especially if we start questioning whether you can meaningfully consent to these relationships, it makes it very difficult to figure out what interventions are appropriate without overreaching. So yeah, bit of a rundown there.
KIMBERLY NEVALA: Yeah, no, that was brilliant and concise for what is, I think, a fairly broad landscape, both, of concerns and, as you rightly raise, challenges to how we have traditionally thought about regulation and compliance. And that does get interesting as well.
You made the comment these systems have access to the entirety of human knowledge and content. And I would challenge that statement a bit. I think that certainly has access to a broad swath of information that has been digitized and digitalized. But part of that allure, I think, that's with these systems is this belief, and sometimes a misplaced belief, in the weight we should give the answers. And part of that is by design and how we interact with them.
You've said part of the issue here with AI companions and some of these components is that sometimes the allure is in the submission itself. So what do you mean by that?
ROBERT MAHARI: Yeah, so really, that was an attempt to express the fact that these systems can be whatever we desire them to be in a way that kind of no human companion could ever be. So it's not to say that the systems behave in a submissive way. It's more that they will do exactly what you want them to do without any kind of will or agency over their own.
Now, of course, that in and of itself is a design choice, right? So it's not inherent to AI that it has these attributes. And it's really hard to talk about this without anthropomorphizing, so you'll forgive me. But I think that if when we're designing a system that was supposed to be a companionship provider, you would want it to be designed that way. You would want to figure out what does the user want and give them exactly that. And that's just not something that humans can do. That, I think, is part of the allure and part of where we see the potential for very, very high usage rates that could then lead to harms downstream.
KIMBERLY NEVALA: Yeah, I'm interested in hearing your thoughts on this as well. Which is in a lot of cases, and even the regulatory frameworks that we see in place, so we look at the AI EU Act-- cannot say those syllables in any sort of reasonable way without tripping over my tongue-- is very much focused on risks, on understanding who is harmed.
You've said it's the million-dollar question: who is susceptible or vulnerable? And that we really need to be careful about thinking about this as a small subset of populations who might fall-- I don't want to say prey, because I think that's pejorative-- but who are susceptible to this kind of influence.
ROBERT MAHARI: So there are two things here.
One is, what factors make you vulnerable to overusing these systems? And it seems, again, like we don't know because we haven't done the research because all of this is maybe two years old. But it seems like loneliness is likely a big factor. And so if you look at the kind of social context in which these tools are emerging, we are, it seems, in a time where loneliness is on the rise, where people are having a harder time relating to each other. And so if that is the risk factor, then that would be really scary because it would mean that more and more people are likely to fall into that vulnerable category. So that's one side.
The other side of it is the cases we're seeing so far often involve minors or people who have some preexisting mental issues and things like that. And I think there's a temptation to say, obviously, we should protect those people, but this isn't a risk for the general populace. And I'm a little concerned about that. I mean, I'd love to be proven wrong. I'd love to live in a world where AI companions provide a useful service to those who need it and, by and large, nobody really suffers any harm. That would be great.
But I worry that we will focus on protecting minors, protecting vulnerable populations, and neglect the possibility that actually all of us might be vulnerable to this. And I think we kind of saw that with social media. I mean, it's not the case that most people are somehow immune to overusing social media. And I think we've kind of cracked the code on developing entertainment products that are extremely engaging for everybody. And so this would be, I think, a scary world if we do the same thing for companionship as a service, I guess.
KIMBERLY NEVALA: And I suppose this issue of-- you're speaking of these systems as entertainment products where someone else may engage for a period of time and use it as a support. A human-like support, I should say and, again, be fulfilling the need that's not, strictly at least, the stated intent - I question this from a design perspective - but the stated intent of companies putting these out there.
ROBERT MAHARI: Yeah, I feel like framing it as an entertainment product is the most charitable way of framing it.
I'm very suspicious of the idea of using technology to cure loneliness, and we've seen claims like this, specifically because kind of at a really foundational level, it feels like someone who is lonely is someone who's not engaging with other people. And so a solution that's premised on not involving other people, like you are alone and not lonely, feels misguided and kind of missing the point. And so I don't know, it feels like a very symptomatic approach to curing loneliness.
And certainly, I could certainly see applications of AI for therapy, where you could imagine that there are certain contexts where someone just doesn't feel comfortable talking to another person and where technology can be more neutral and maybe somehow more approachable. And if that's the case, and if those tools have safeguards, amazing, right? That's a great application.
But if you have-- I mean, some of the tools we've seen that focus on, or at least purport to focus on, the mental health side essentially are platforms where you have user-created companions that other people can use. That doesn't feel like it has the safeguards in place that you would expect from something that is claiming to provide mental health care services.
And so, yeah, I think entertainment feels like the somewhat innocent and kind of legitimate application. But certainly, I think the ambitions are bigger. Yeah.
KIMBERLY NEVALA: Yeah, I would agree. And there's also-- we're going to talk in a minute here about the Data Commons as a whole and some of the challenges there, so in a completely perhaps divergent portion of this conversation.
But one aspect of all of these systems is that they are hoovering up immense amounts of data. And in some of these applications you've just referenced or even in that personal interaction that are very private, very sensitive. And it's not clear to me that users truly understand or that we've cracked the code on how we make really, truly informed users across the board, Both in cases where I could see a subtle danger in the user who says, well, I know this is just a system, and it's just fun to talk to, and we fall prey to our own human tendency to anthropomorphize and start to relate to these things. As well as someone who's actually in a critical state and not in a place where they're worrying or thinking about some of those aspects.
ROBERT MAHARI: Yeah, it seems like some degree of consumer protection is needed. The idea of just fully putting the onus on users in these contexts feels really unfair.
The other thing that-- since you mentioned people talking about really sensitive things, there is this question about who's behind the technologies, and do we trust that entity? And you could totally imagine that people would divulge details that could, at a minimum, be used to sell them products, at a maximum be used for blackmail.
And so there's this whole other kind of national security type question kind of looming in the background of all AI regulation, I would argue, because if we don't build it, somebody else will build it. And maybe they are less aligned with our values. And so we should get there first. It's hard to put up barriers in the web. All these things are true.
So yeah, I really wish that there was kind of an obvious good solution to this, but unfortunately there isn't. Or the good solution, the best kind-- I turn 30 later this week, so I still get to be like young and idealistic. So the best solution is to fix loneliness. Then we're fine. Then we're not vulnerable. And then if people use these tools, they'll do it in a positive kind of way. But unfortunately, we're probably not going to fix loneliness. And there's going to be a lot of demand for these kinds of tools. And then you could see all sorts of harms emerging from that.
KIMBERLY NEVALA: So let's talk a little bit about what we might think about or need to consider. And you've made the point very clearly that there's a gap here which neither law nor technology can solve or address alone.
And one of the points that you made was that regulation traditionally avoids intrusion on personal companionship. It does strike me a little bit that even that terminology - as you said, it's hard not to anthropomorphize - somewhat gives credence to the idea that the system that you are talking to is, in some way, an individual or a companion. I don't know how you get around that as well, but I'm a little worried about the language, if that cedes ground before we even get there.
But can you talk a little bit about where that approach to regulation and compliance in general just falls short; is really not prepared to address these issues today?
ROBERT MAHARI: Yeah, so at least two things.
So the first is I'm happy to live in a world where the government doesn't tell me who I can be friends with, who I can date, who I can love. I want to live in this world. This is a good thing. And we fought really hard to be in this world. So this is good.
That being said, it creates a challenge when you have the potential for someone to say, look, I'm not hurting anybody except for myself maybe. And I don't think that I'm hurting myself. I have fallen in love with, or I have decided to be friends with an AI, and who are you to tell me that what I want here is not what I deserve?
It's not even like smoking, where you can make an argument that you're either hurting others by kind of smoking around them. Or you're burdening others because you're going to have medical issues that society will have to pay for. Because it's not clear that someone who is spending all their days using AI companions is somehow burdening society. If anything, I mean, if you want to be really crassly utilitarian, you could say, well, that person's probably not going out, so they're traveling less, they're using fewer resources. So it's not clear that that argument holds.
So we really have a situation here where if there's harm, it's purely to the individual. And I don't know how we get around that. Where we say, like, OK, well, you may want this, but we, society, think that it's not good for you and so we're going to take it away from you. I think that's really tricky. And I think that maybe the silver lining is that we're talking about this relatively early, although I keep being surprised by how quickly things are moving.
But it's not as though we were in a world where it's kind of done, cat's out of the bag, everyone's addicted to AI, and if you try to outlaw it or limit it there would be mass riots. So maybe we can have an opportunity here to have a positive impact on the way these tools are developed and guide them. Again, recognizing though that this is kind of a highly fragmented international marketplace. And if there's a demand for something, then there's probably going to be a supply for it too.
And it's not like it's particularly challenging to use existing-- it's hard to make a large language model. It's not that hard to wrap a large language model in an interface and provide a companionship service. So that's kind of scary and challenging.
And then kind of more broadly on regulation, it feels like - not just in this case and not even just in the AI case - but often the optimal regulatory intervention depends on all sorts of variables. And it's traditionally, especially when you're regulating humans, been hard to take account of those variables.
I'll give you the example of speed limits when you're driving. The 60 mile per hour speed limit is not actually the speed limit that is going to minimize collisions on that stretch of road in that moment. That speed limit depends on whether it's raining, how many cars there are, how skilled the drivers are, and a bunch of other factors. But if you had speed limits that were kind of constantly updating like that, it would be a nightmare for human drivers. So we don't do that.
And so you could imagine that if you had self-driving cars, they would get an update every mile they're traveling down the road what the speed limit is that they should be driving at and that embedded in the technology was a mechanism such that they did not exceed that speed limit. This is this idea of regulation by design. And it could be the case that there's something to be said for that in the AI companionship case too.
So we explored briefly in the piece the idea that the kind of safest AI companion probably depends on the user. And there are probably some people for whom it's totally fine to have a very deep, very involved discussion, some users for whom it's totally fine to have a really sexually explicit discussion, some users who really need something that resembles AI therapy. And so in an ideal world, the guardrails we have in place wouldn't be one size fits all but rather would change and adapt to how the user is acting.
The scary thing here is that it kind of presupposes that you're doing a ton of data collection and a ton of modeling of users. And so maybe that's a good thing if you're using that data to keep users safe. Obviously, it's a bad thing if you're using it to take advantage of them.
And I do think it kind of comes back to, I hope, that we will live in a world where the entities deploying AI systems like this are entities that can be held accountable. Are entities that are in our jurisdiction and have to obey our regulations. Because if we live in a world where you have these kinds of fly-by-night providers it will be hard to get them to do anything. And then we're really limited to either some sort of national boundaries around the internet, which are going to be really hard to enforce and have lots and lots of other negative consequences or hoping that educating users is sufficient. And maybe we'll have classes in middle school about the way we've taught people about how to be safe on the internet, kind of an extension of that and hoping that that's sufficient. Who knows?
KIMBERLY NEVALA: I mean, there is a self-fulfilling prophecy here. It says for us to be able to manage this really well, we need to be able to first understand what we think the-- it's yes, the individual level. What's that balance between this might not hurt that individual but when it's at mass, all of the individuals operate in this way, then have a societal impact that is negative for all?
But we are still challenged today with even our basic ability to really understand. If you're interacting with a system and it's monitoring and recording you and trying to analyze your affect, what you're saying, your facial expressions. That science is still a bit of smoke and mirrors magic. It's not too far from, sadly, the physiognomy of way back. And so it also strikes me that some of this is very much dependent on us being able to realize some science and some capabilities that don't exist yet as well. And that makes this a particularly interesting point in time for that reason as well.
ROBERT MAHARI: Yeah, so in general, I'm just a kind of tech optimist. I guess like a pragmatic tech optimist.
So on the self-driving car thing, humans have certain limitations. Humans are remarkably good at doing stuff. And so it's been really hard to do better. But eventually - I just don't know if it will take 5 years or 50 years - but eventually we'll figure out how to make robotic systems that can respond as well or better than humans and that are more reliable. I'm just fairly certain eventually that will happen, but I don't know the timelines.
And for AI specifically, it's been such a-- you are always on the losing side if you bet against some technical innovation. And so, especially when it comes to the harms piece, I'd rather just assume we can figure out all of the technical stuff and then ask what that world looks rather than living in the hope, like, oh, as long as we don't figure out x, then we'll all be fine.
Now, you flipped this because I was saying, well, we need to figure out how to measure how people are feeling in order to have an effective intervention. But it could be that really simple things would work, like the number of hours you've spent on the platform. And this is where doing this human-computer interaction research is important because maybe it would be sufficient to just have a popup that said, hey, we noticed that you spent six hours on the AI platform today, is everything OK? Or the more extreme version of this is, like, you've spent six hours on the AI platform today. You can't use it tomorrow. And it will be available again in a couple of days.
I mean, this is a kind of mundane example, but there are retail brokers that if you want to do options trading, they essentially require you to apply to do options trading, and you have to have a certain track record. And they kind of, over time, give you different levels. So like, Charles Schwab is the example that I'm thinking of. They have these different levels for option trading. That seems sensible. I don't think anyone's really forcing them to do that. They certainly have financial incentives to have people behave somewhat reasonably. But for the most part, that risk is being carried by the retail investors.
And so, I don't know, kinds of interventions seem like they'd be a good idea. And it doesn't necessarily require science fiction levels of affective computing. Which maybe are something that we want to stay away from anyway because they are invasive in this kind of context.
KIMBERLY NEVALA: And perhaps maybe that is the takeaway, which is part of our tendency is to want to tech our way out of some of these things. Where someone might argue with the self-driving car issue: really the better answer is for us to think of an entirely different paradigm, where we have perhaps automated public transit that is just sort of perfectly connected to all of the right bits. We don't have that infrastructure today, but that doesn't mean we can't imagine one for tomorrow that looks different.
And to your point, in the same way, maybe part of the protections in this area are us being mindful of really not knowing what we don't know because who knows. We could be-- or not we, I'll say it, me-- could be dead wrong, and this just turns out to be a massive positive for individuals and society.
The possibility is there.
But one of maybe the inclinations we need to back away from is thinking that we can, A, regulate our way out of it, or we can technologically design our way out of it. So some of the guardrails may be very simplistic or not deal with technology at all. And so being open to some of that as opposed to just continuing to tech for tech.
ROBERT MAHARI: Yeah, no, I totally agree that. Neither just regulation nor just technology will fix this.
It feels like at a really high level, it's an incentive alignment problem. Where if you have economic incentives such that you have providers of AI systems that make more money the more you use the systems and you have users who are more harmed the more they use the systems, well, there's a misalignment problem.
And if we can find some way to align that, then, e.g. attacks on engagement where the platform makes less profit off of you as you use it more. Maybe that's the fix, or maybe that just makes it all worse because that tax will be passed down to the users who are most vulnerable. Who knows? But thinking about it in those terms. And I think there are technical ways that can serve as safeguards, and we need law to create incentives that get the technologists to do that. And so there's kind of a relatively obvious interplay between the two.
But I totally agree that one alone is not going to work. And because there are low barriers in the internet marketplace, you almost have to win. You want the safest tool to be the best tool because then people will use that. And so I think that the free-market solution would be the ideal one. I don't know if that's actually feasible. But that would be the best-case scenario. We find a way to design these tools in a way that people want to use them but that are designed in a way that's relatively safe.
KIMBERLY NEVALA: So we touched on regulation by design, not so much about legal dynamism.
But you also had proposed that one element or one portion of the solution, again acknowledging that there's no single solution to solve this fully just due to the nature of the problem and our complexity as humans, quite frankly, was agentic self-awareness.
Now, I, in looking at the phrase said, I bet people are going to think you mean one thing, and in fact, what you were talking about was different. So talk to us a little bit about that concept of agentic self-awareness. And when you say agentic, what do you mean?
ROBERT MAHARI: Yeah, so AI agents is the new buzzword for 2025. You heard it here. Well, probably not first because it's March. But everyone's very excited about AI agents.
I think a reasonable definition of what an AI agent is it's an AI system that can use tools. So, text-based, chat-based interface is not an AI agent because it can't do anything outside. The moment that that chat-based interface can book a flight for you or look up restaurant recommendations or even do a internet search, now it's using external tools, and now the lines get fuzzy, but maybe it's an AI agent.
And lots of people, as you might imagine, there are so many applications for this kind of thing, so lots of people are working on it.
But really what we meant here is different. So if you look at the EU AI Act, it is a risk-based framework. We talked about that before. And essentially what it does is it has an appendix. And in that appendix, it has a bunch of high-risk areas of AI application. And those include things like education or the administration of justice.
The problem is that if you take a general-purpose system, like ChatGPT, Gemini, Cloud, et cetera, DeepSeek, you can very easily imagine how you could use that system for a high-risk use case like education. So if I go to that system and I ask it: I never quite understood the history of Switzerland, could you explain it to me? Then it provides an answer that's probably fine. That's not education in that sense.
If a teacher, meanwhile, uses the same system to create a little tutoring bot where the students could do Q&A or an assessment bot, that's definitely in scope. It wouldn't take much engineering - I mean, it wouldn't take any coding - but suddenly you're in the high-risk area.
And the solution to that may be that the systems check whether they are performing something that is considered high risk under some regulation. And then they can do one of two things. So they can either say, hey, it looks like you're trying to build a classroom assessment tool. Are you located in Europe? Because if so, that's a high-risk application, and I can't proceed. Or it could say, and if so, I will put in place safeguards in the background that ensure that we do this in a compliant kind of way.
And I think one of the key benefits of this type of regulation by design-- I think this is a nice example of regulation by design-- is that it eases the burden on innovators a little bit. So if you look at the EU AI Act, it's 480-something pages long. It's really complicated. There are a lot of ambiguities and gaps that need to be filled. So the full amount of regulation you need to understand is hundreds and hundreds of pages.
And if you are a small company that is trying to do something creative or even a big company that isn't a tech company but is trying to use AI in some way to make things better, that's really intimidating and hard. And I'm definitely not the first person to suggest that maybe Europe is going to see less innovation in this space because they've created a really kind of complex and cumbersome compliance overlay. And if we can use technology in a smart way to ease that a little bit, that seems appealing.
So yeah, that's the whole kind of idea. And all of this has something to do with AI companionship in that maybe that's one of the places where we'd worry about it. But it's much, much more broad than that.
KIMBERLY NEVALA: Well, it also strikes me as this is a good way to also start moving back some of the accountability and even the liability for when you're putting systems out in the world.
There's a tendency today, and LLMs are probably the most obvious or frontier models of different flavors area for this, where there's a tendency to say, well, we can't guarantee 100% safety, we can't guarantee 100% regulation, and therefore, it's just sort of impossible. Instead of, as you say, putting in a best effort or making the best. And I think it's fair to also ask the question, if you cannot do that, should it be allowed? What is, in fact, good? What does good innovation look like? And is all innovation good?
ROBERT MAHARI: Yeah, I think, I don't know, we're probably all, as a society, going to have to become more comfortable with probabilities. Because you hear this thing all the time, where it's like, well, we can't guarantee it, so we won't do it at all. And that, to me, is like saying, well, there's a chance that your climbing rope will snap, so you should probably climb without one. It's like, really? That's your conclusion?
And then, for certain types of things, there's a benchmarking question. So we have all sorts of rules in place and mechanisms in place to govern humans. And we know that those mechanisms aren't perfect. And so when we apply them to AI systems, we're going to want to think about, well, what does good enough look like? And maybe in some cases, it's about benchmarking against the human system and saying, well, actually, now that we've checked the humans that we thought were perfect are only 99% or 80% or 70% accurate, so as long as we're above that threshold, at least we're moving the needle in the right direction. And you can start having these kinds of discussions.
KIMBERLY NEVALA: Yeah, I suppose the thing that I've observed missing in a lot of those human versus AI comparisons is the question of scale. Which is how much harm do singular or groups of people perpetuate – or benefit, and so I should say harm or benefit - from doing that? And it gets a little questionable.
So I did want to spend a few minutes at the end here, and it has to do with protection of what we're now calling the Data Commons, the digital Data Commons, and the ongoing or evolving debates, discussions, arguments about data set licensing and attribution for AI.
You’ve made the argument or have pointed out that perhaps with all good intent with what we're trying to do with enforcing, licensing or pushing licensing regimes for AI systems we may inadvertently be weakening the web and limiting data access for everybody. Can you talk to us a little bit about the tension you're seeing evolving here and how we might think about it?
ROBERT MAHARI: Yeah, totally. And this is a place where my own thinking has evolved.
So when I first started thinking about copyright and AI, I kind of took the position that, whether it's right or wrong, just the reality is that all the data on the web has been scraped and trying to especially retroactively pay people for the use of their content to train AI, it's not going to work, and we're going to spend a lot of effort on it. And it's not even clear if it will have any benefits.
And so that was kind of my thinking. And we started this research project where-- and by the way, kind of as an aside, but this was a research project with 54 co-authors. It was kind of a huge undertaking. This is not easy. But anyway, we wanted to understand, where does the data that is used to train AI models actually come from?
So we looked at this data set called the Common Crawl, which, for all intents and purposes, is just the whole internet. And we went down it-- we sorted it by the number of words per domain, and we went down the list for the first 15,000 or something like that. And we studied what is this website? Where is it coming from? And critically for this discussion, does it have any safeguards in place, or has it put any safeguards in place that might discourage AI crawling?
And one of the things we found was that in a world where there are few, if any, legal protections - I mean, we see a bunch of lawsuits, but it's not that there are great legal protections for content creators to limit the use of their content to train AI - one of the things you could do is you can try to protect it yourself. And an effective way of doing that is to put up paywalls or to put up some other mechanisms, but paywalls are probably the most relevant one for this discussion, put up paywalls and restrict access for AI crawlers.
And kind of obviously, this has an impact on real human users. So we see a "paywallification" of the web in an attempt to protect content from being used in AI that has had this kind of, I think for all parties, all involved parties, a somewhat unintended consequence.
The other thing that we're seeing that I'll mention briefly-- it's not what the article you're referencing is about-- but we're seeing more and more people using AI as an interface for the web. So instead of using Google Search, getting a list of domains and then clicking those domains and exploring the content, we're seeing AI interfaces that essentially perform that web search for you and summarize the information. You never have to visit the website. And it looks kind of anecdotal initial evidence suggests that there is a decline in actual website visits, that people are going to websites less, which means that monetization for things like news is likely going to get harder.
And that's different than you're using the content to train AI models. Now you're actually affecting the business model of news. And I think that, I mean, this is a whole separate can of worms but it's going to raise really interesting and challenging questions about how do we continue to incentivize the human creation of content. Because for the time being, I don't think AI is going to do journalism very well. I mean, it might write prose, but it's not going to go to war zones and investigate. And so we need that function, and we need incentives. So yeah, whole different can of worms, I guess, but interesting.
KIMBERLY NEVALA: Yeah, it is and even that idea of this becoming the new interface. I think there's plenty of discussion right now there about the obvious difference. Yes, it could come up in search and certainly Google's monopoly all sorts a whole different problem, but at least it forced you down then to get to where the content was, in theory, created or syndicated in a licensed way. Although that was not by any means perfect either. So there's just another way of-- as you said, it's a business pressure and a disintermediation of people from information and sources of truth and information, all these components.
So I think this is a really interesting area. And again, one of the elements that I found so interesting in that article, which we'll link to, was this question - and it wasn't actually something I think the article suggested - but I could see someone going to say, well, clearly, what's the least of two evils? Which is you have to give-- everyone gets access to all of your content-- because that ensures that folks who already have limited access to content don't get completely closed out because they don't have the dollars to pay for it or the means to pay for it or even just the wherewithal to go and find it. But then, also, it continues to incentivize the really bad behavior and disincentivizes content creation or at least devalues it. So it's going to be an interesting place to watch.
ROBERT MAHARI: Yeah, no, it's really interesting. And if you think about what is the original purpose of copyright was to support science and the useful arts. And so it might require some updated thinking on what it looks like to do that and what kind of policies achieve that.
KIMBERLY NEVALA: So to wrap things up, as you're looking out over this landscape and starting to do research and thinking critically about all of these areas, what are the questions that you're going to be pursuing or most interested in pursuing here in the next bit?
ROBERT MAHARI: So it's so much fun to be an academic at this intersection because there's just so much. And a lot of it is knowing when you're not the right person to answer the question, even though it's pressing.
So there are a bunch of questions around the actual impact of AI companions, what kind of safeguards and designs make sense. I'm not the person to do that. I really hope that we see more interdisciplinary focus on that.
And I mean, this is not what the podcast is about, but academia is going through its own chaos right now. And I worry, among many other worries related to that, academic institutions are going to try to play it safe. Interdisciplinary research traditionally is not playing it safe. And so I'm a little bit scared that we're going to not see the types of collaborations we need between psychologists and anthropologists and social scientists and technologists and lawyers to really answer these questions effectively. So that's kind of an aside and not really an answer to your question.
I'm really interested in taking the regulatory safeguards and trying to figure out how we can develop technologies that mesh with those. So we're doing some work now on copyright, like essentially creating AI models that cannot output images that are likely to violate the copyright of something in the training data. Which turns out-- I started this project, and I was like, well, we'll just learn what human preferences around copyright and specifically this idea of substantial similarity are. It turns out, obviously, that humans are not always consistent in what they consider to be similar. And so I'm scared that this project might devolve into a hmm, copyright might be fake because juries are really not consistent and we can't find any strong signal to capture in the first place. I don't know what we'll do about that, but I think this kind of technifying the law in a responsible way, maybe that's the tagline, leaves so many opportunities.
And then, of course, the other big piece is we see regulation in Europe, we see a little bit of regulation in various US states, a couple of other countries. But for the most part, the world has not decided how to proceed here. And it's a really exciting opportunity, I think, to offer evidence and paths forward. I'm a big believer in evidence-based policy here. I worry that it's easy to miss harms. It's easy to overregulate in one area, underregulate in another. All these kinds of things have unintended consequences.
So insofar as academia can provide the evidence needed to base policies on, I think that's an important dimension.
And then, finally, I realize that's not what the podcast was about, but there's also this access to justice crisis. And I think that AI and technology can do a lot to support that. And justice is this amazing field because, on the one hand, it's founded on language, so perfect deployment area for large language models. It is also so sensitive, and the human dimension matters so much. And you can really screw things up. And so I think it's a really interesting place to think about, how do you make things better with technology? How do you keep human dignity and the human dimension of these processes intact?
And yeah, I think it's clear that the current system is not optimal by any stretch of the imagination. And that's speaking for the US, which is bad, but by no means the worst offender when it comes to access to justice.
So yeah, I'm keeping myself busy, I guess.
KIMBERLY NEVALA: Well and I think asking all the right questions. And I would just want to underscore and underline that question that I definitely, in getting to know you and reading your work as well, even in a limited fashion, I know that you always come back to, which is: do I know enough to-- am I the right person to ask this question? So it's about both raising all of the right questions and then the humility to bring the right folks into the room to then answer them. And that is something I think we could all, certainly myself, but as a collective, benefit from having a lot more of.
ROBERT MAHARI: I mean, look, I don't think people are in academia for the money. If they are, they made bad choices. So we're here for the knowledge, right? And sometimes, by the way, the knowledge leads to companies and money. I mean, that's the reality of it, and that's totally fine.
But these kinds of collaborations, like aside from being genuinely useful, there's so much fun. You learn so much. And it is, I think, a real privilege to have a job where your profession is to learn, to discover things. And learning from others is a part of that. So I realize it sounds very idealistic. But genuinely, I think that these kinds of interdisciplinary collaborations can bring out the best of what academia ought to be. So yeah, I'm excited to do more of them.
KIMBERLY NEVALA: Well, that is fantastic. And we will look forward to seeing what comes out from those fantastic collaborations. And really just appreciate the time and the insights and thoughts you've shared here today.
ROBERT MAHARI: Yeah, thank you so much for having me.
KIMBERLY NEVALA: Yeah, anytime. We will definitely have you back.
If you'd like to continue learning from thinkers, doers, and advocates such as Robert, please subscribe to Pondering AI now. You'll find us wherever you listen to your podcasts and also on YouTube. In addition, if you have comments, questions, or guest suggestions for us, please write to us at ponderingai@sas.com.
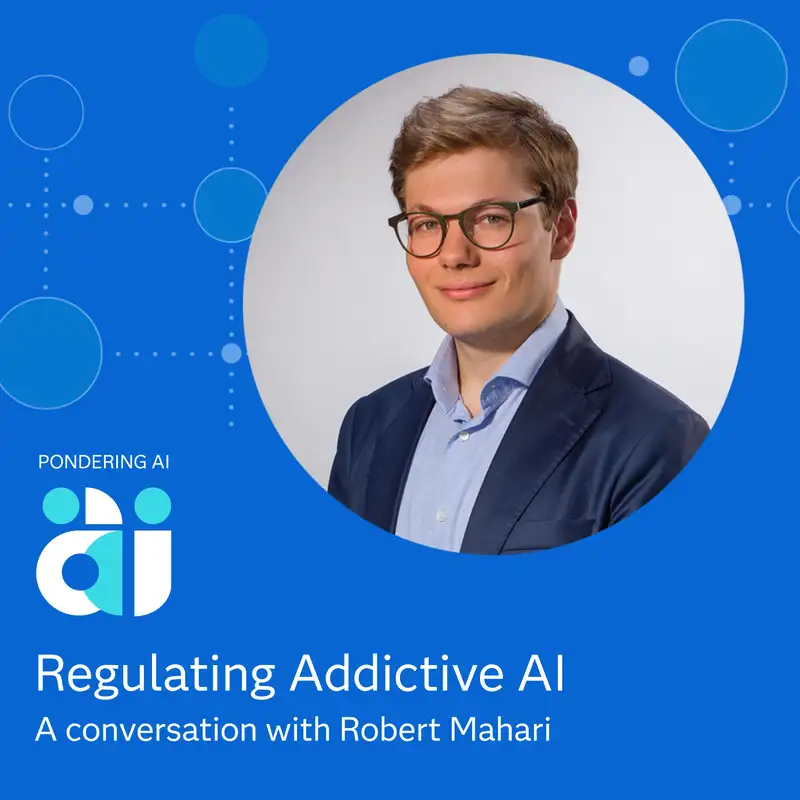