AI Literacy for All with Phaedra Boinodiris
KIMBERLY NEVALA: Welcome to Pondering AI. I'm your host, Kimberly Nevala.
In this episode, it is a pure pleasure to bring you Phaedra Boinodiris. Phaedra is a global consulting leader in trustworthy AI at IBM, and author of a fantastic book, AI for the Rest of Us. Amongst numerous other activities and accolades, she has been honored by the UN as a Woman of Influence and was AI 2030s Responsible Leader of the Year for 2024.
We're going to be talking about learning, participation, and accountability in AI today. So, Phaedra, thank you so much for answering the call and joining us on the show.
PHAEDRA BOINODIRIS: My pleasure. Thank you for the invite.
KIMBERLY NEVALA: Now, you have a long interest and enjoyment with gaming, both personal and professional. I'm interested in knowing if that interest, that engagement, has influenced or colors how you approach your work in the broader AI sphere today.
PHAEDRA BOINODIRIS: Oh, goodness. [LAUGHS] What an interesting question to start with. [LAUGHS]
The answer is, absolutely. Actually, through gaming is how I got first interested in the subject of artificial intelligence. Sort of the integration between AI and play has always been very appealing to me. And I really had some opportunities in my career to explore that space, which was tremendously fun, in particular around player engagement. What does it take to really engage a player?
And, in fact, just this past Christmas-- actually every Christmas my husband and I, we design games in order for our kids to unlock to get their presents. I know this is totally sadistic, and it really is.
KIMBERLY NEVALA: This is awesome.
PHAEDRA BOINODIRIS: Like, it's a set of puzzles and gameplay where they actually have to work together in teams. Because we have four children, big variation in ages. And we've been doing this since they were little. And the older they get, the harder it is for us to design these puzzles and these games.
In fact, for years we had to start around Thanksgiving time so that we could have enough runway to be able to do this. We got to a point, Kimberly, where we had to have, like, an entire library with, like, cryptography books, because that's how sophisticated these puzzles have had to get. I know by the time our kids all graduate from college, they're going to be ready for, like, the NSA and God knows what because of their ability to crack codes.
But a couple of years ago we started to play around with AI to see, gee, could we use AI to speed up this game design process, which has been so interesting, really interesting, because it gives us both a better understanding as to where gen AI is today, like what are still its limitations, where has it really advanced. And it's just a really fun exercise to do.
KIMBERLY NEVALA: That's awesome. So for everyone out there, we now have our new mission for the holidays, whichever holidays, whatever holidays you participate in the next year.
Now, I really enjoyed the book, AI for the Rest of Us and frankly, your corpus of work at large. One of the things that you've really spent a lot of time emphasizing of late is the need for us to lean into literacy. And I want to start first by asking if, in your perspective, has the onslaught of generative AI changed the focus or the imperative for broader AI literacy? And if so, in what ways?
PHAEDRA BOINODIRIS: It absolutely does, because, as artificial intelligence is being used more and more and more frequently in our daily lives, it's incredibly important. It's crucially important for us to understand, how is this technology made? How is this approached? Who does this technology give power to? What is the kind of data being used to train these models? Who's accountable for these models? How accurate are these models?
I mean it's really, really important for us to have an understanding about this technology. Because as I said, it's being used more and more and more. And I am concerned that when people do not have that baseline level of literacy they will make some very big assumptions about this technology. Like everything that this model says must be correct or that there has to be some organization who is accountable for the outputs from this model or et cetera, et cetera, et cetera. So having that level of literacy, I think is going to be very empowering for people.
KIMBERLY NEVALA: And is generative AI different from the perspective that it is just so broadly and publicly available? A lot of the issue in the past has been this question of, well, people don't even know they're using it. Or they don't know it's being used on their behalf or for them, right, or against them, depending on the use case and your perspective. Has that ease of use also created or expanded the - I don't know if I want to call it the risk landscape - or the spectrum of risk that's out here today?
PHAEDRA BOINODIRIS: I think certainly the ease of use, the level of accessibility of the technology itself, paired with the lack of understanding and the lack of accountability is a dangerous mix. I think that's the part that I'm deeply concerned about, and why I think there's a real opportunity for us to be rethinking how we approach the subject of literacy.
What do we even mean when we say literacy? Does that mean we need to teach more coding in computer science classes? Is that what we're talking about? The answer is no. That is actually not what I am talking about.
Most people think 100% of the effort to develop these AI models is coding. It's not true. It's not that there's a group of computer scientists sitting in a room by themselves and then coding or programming something. Well over 70% of the effort to develop these models is choosing data, picking data. Right.
So we have to ask ourselves really tough questions about the nature of data. And my favorite definition of the word data is that it's an artifact of the human experience. Right. So we humans, we generate the data, we make the machines that generate the data. But we have over 180 biases. So it's really important that we understand our biases, because these models act like a mirror that reflects our biases back towards us.
So we need to have people trained to ask really tough questions about the nature of human data. Like, is this representative of all these different kinds of human beings? Was it gathered with consent? According to domain experts, is this even the right data to be using to solve this kind of problem? Right.
And then questions on top of the nature of data, like, is this an appropriate use for artificial intelligence? Is this augmenting human intelligence? How do we know if a human being has been augmented by AI? Have they been given power, empowered? What is the experience like of a human being if they've been augmented by AI?
And all of these questions, like, these aren't technical questions. This is not questions for programmers in a computer science class. These are questions for everybody, certainly for multidisciplinary environments, for philosophers, linguists, lawyers, psychologists, anthropologists, other forms of domain expertise. I mean, this is why, when I'm saying the words literacy, it means holistic literacy.
KIMBERLY NEVALA: And so we're going to talk a little bit about what it takes to have a fully engaged and participatory environment for AI. But I want to linger on the definition of literacy for just a minute longer and also what you think the objective of a literacy program should be and if that differs by audience.
So you were just talking about, in the context of organizations, thinking about, how do people understand the data and the information that's being fed to, consumed by and then being spat out, if you will, by the systems themselves. So there's an organizational lens, and still a development lens, but it's a development lens that goes beyond just developers. And then there's the perspective of, what about all of those out there, all the rest of us, that are either engaging with companies who are using these systems to make decisions? Sometimes opaquely, sometimes not. Or where we're engaging with services that almost by definition and by design require us to engage with AI systems that are integrated into these process flows.
And I've started to wonder and I think this might not be an either question. It might be and or. But should the focus of our literacy efforts be to help people learn to work with it or should it be so that they learn how it works? And I think there are fundamentally different trajectories and outcomes that might come with those two.
PHAEDRA BOINODIRIS: The answer is yes and yes. [LAUGHS]
Yes and yes. It is fundamentally important, I think, to understand, what is AI? What is gen AI? What are the limitations of predictive models? What are the limitations of generative models? Understanding or asking the questions, what data was used to inform this model? Why did I get this output? Who's accountable for this output?
And then to be thinking about now how might I use this in order to augment my intelligence or augment what I do at work? How should I be a critical consumer of this technology so I can glean the kinds of insights that I need to in order to be better at what I do? But you can't really do that until you understand, what are the limitations of the text so you can know how to be a critical consumer.
KIMBERLY NEVALA: Do you think …today, like where we sit today, is it a reasonable expectation for us to look at what I'm just going to call, the rest of us. Can consumers at large today reasonably be expected to be self-protective and responsible users of the technology in a timely fashion? And if not, what is it going to take for us to sort of bring them along as this progresses rapidly?
PHAEDRA BOINODIRIS: I think that is the question. I really do, like how fast can we move? And I think in order to bring people along as fast as we possibly can, we really need to recognize that we have a lot to unlearn as much as we have to learn.
And when I say the word unlearn, what I mean by that is unlearning who gets a seat at the table in these conversations. So if you're lucky enough to take a class on the subject of data science or artificial intelligence, you're likely in a higher ed institution in a school of engineering. And as we've just described, the hardest parts of getting this right have nothing to do with coding. It has to do with answering social questions. Right? Is this person being empowered? What are the human behaviors that we are trying to measure that we wish to see more of? Right? Again, the human psychology. Is this gathered with consent? Is this representative? Is this going to solve the problem?
And in order to be able to unlearn this question about who gets a seat at the table, there has to be that kind of introspection first. And an understanding and a realization that the school of engineering has to now work with the school of government, has to now work with the school of philosophy. Like, we've been doing it wrong for a very long time, to have all, like, the humanities program over here, and the school of IT and tech and engineering over here.
What we're seeing now is a result of that fractured, siloed approach to education. And so I think with that level of introspection that we can move forward with our educational institutions and be able to craft things that are far more holistic, far more intentionally accessible and inclusive to a wider variety of
different kinds of disciplines.
KIMBERLY NEVALA: Do you think that division, if you will, what we've learned, which is these discussions of artificial intelligence, of computer system design, development and deployment being siloed in at the academic level - and here we're talking about graduate or post-graduate secondary education - then has naturally trickled down into what we see within organizations where you don't have folks who represent those other disciplines and perspectives?
You might not, right, unless you're in a very large organization. And then the question is always, are they motivated to do this? You may have folks who are UX designers, user experience, or human-centric designers. But you may not have philosophies. You may not have linguists. You may not have some of these other social science or humanistic-related domains within the organization. And we may never have really thought we needed them in some ways. So is this, do you think, an output, a natural output of the way that we have taught this for a long time, in that we don't --
PHAEDRA BOINODIRIS: I do.
KIMBERLY NEVALA: -- then hire into those skill sets within organizations?
PHAEDRA BOINODIRIS: 100%. 100%. Because you have technologists that are building models that do not understand disparate impact at all, because they weren't taught it in school. They were taught, you don't need to take those humanities classes. You don't need to take 'em. You go study computer science and learn how to code. And so what we have is a lack of understanding about how a technology like this can be built in ways that represent us all and can truly be empowering, and in ways that specifically address risks of disparate impact.
One of the clients I've had the pleasure to work with recently has been a very large police department. And it's been fascinating to work with them, because so many of the domain experts in this police department who, of course, are experts in policing or social work, as an example, right --
KIMBERLY NEVALA: Yeah.
PHAEDRA BOINODIRIS: -- did not realize how critical their roles were, their skill set, their competency in conversations about AI models that are going to be used in their domain. So people who have domain expertise don't even realize how critical their domain expertise is into getting these models right. And again, that goes back to unlearning. Like, who belongs? Who doesn't belong? The truth is, we all need a seat at this table.
KIMBERLY NEVALA: How do we ensure that happens? So this is a good example of the idea within an organization of that participatory design, even though this is still, to some extent, I presume, within the boundaries of, for instance, this organization, the police service that you're working with.
A lot of the pushback we will get is that, listen, the business of business, right, is not to have to worry about, is not necessarily to have to worry about these things. And I don't agree with that. I'm just saying it's a pushback. Or that corporations and organizations are only going to be as accountable as they need to be.
In your mind, is that just a blatant, you know, way to just avoid the problem? And if we start to retrain how folks think from an earlier age, would we see this approach take root more organically? Or is it taking root in our people who think that this is just nice talking points, but it's never going to happen and organizations are never going to step up to the plate in this regard, are just not seeing it happen?
PHAEDRA BOINODIRIS: Well, there's some interesting statistics coming out that says 85% of AI projects fail. 85% is a huge, huge percentage. And there's three reasons why 85% of projects fail. One is the investment was never directly tied to the mission or to the business strategy. That's one. The second reason? Lack of skill sets and talent. The third reason is people don't trust the models.
So, again, we circle back to trust. What does it take to earn trust? And you cannot, cannot talk about trust until you talk about accountability. And one of the things that I have seen shift - and this I would say in the last year, year and a half - is more and more organizations are tapping individuals to be accountable for these models. Right. And it's a big job, and you have to have a funded mandate to do that job, and you have to have a lot of power. And the job is growing, right, because not only do these people have to get value alignment within their organization, meaning everybody has to recognize how critically important it is that these models reflect the values of the organization, that they're behaving in the way they need to behave in order to be compliant and ethical. Right. So that's value alignment.
The second is, you've got to understand what you've got. You have to build an inventory of all the AI models that you've ever bought or that you're building and keep track of them. Like, what's the metadata associated with these models? Right. You have to keep track of all the regulations and the new ones that are coming down the pike. But there's a recognition that you can have AI models be lawful but awful, which means you have to push into ethics.
And as soon as you start pushing into ethics you've got to be a damn good teacher. Because you have to start teaching people, what are the ethics of that organization? And not just to those governing those models, because you have to teach people how to govern them. Like, how do you audit a model to make sure it's behaving the way the organization needs it to behave? You have to be teaching those buying models on your behalf to make sure that the models they're buying reflect the ethics of the org.
And all of this is really, really hard. None of anything that I just described to you, none of it is easy. It's really hard to create AI models that are trusted, really, really hard. And I think that is one of the first things we need to be teaching is, what level of effort it takes in order to make sure that these models are indeed safe and trusted and reflecting our values. Because I think people, for whatever reason, think this stuff is easy, and it's not. And it takes a lot of different kinds of brains, different kinds of human beings in order to build these well.
KIMBERLY NEVALA: And I suppose there's an element of this where, to some extent, these days, developing a model or starting to engage with any of the broadly publicly available LLM-based systems is in some ways easy. And maybe that ease of accessibility or the perceived ease of just developing an algorithm is leading us astray. Is that fair? I don't know. Is that a -- [LAUGHS]
PHAEDRA BOINODIRIS: Because it's so easy to grab. Like, let me just grab this and then ask the question. But again, it goes back to being a critical consumer and asking the question, like, is this output right?
I think the more we learn to be critical consumers of the tech, we can start asking ourselves the question, like, is this fair, does this represent us, what do we need to do in order to have this better represent us or better reflect us, or even better reflect me? What does it take in order for an individual to be empowered by AI, using their own data that they trust?
KIMBERLY NEVALA: Yeah. And a lot of times those conversations still come down to how do we phrase or approach the conversation in a way that we are asking, like, really focused on people accepting the systems that we are creating. As opposed to here's mechanisms or ways to have a step back and say do I want to do this at all? Do I want to engage at all?
And back to that question of what is our focus with literacy and enablement? Even with the public, do we need to be raising up the conversation about how they can also push back, or how they can ask questions; even to their trusted brands and the consumers and the folks that they want to engage with and wish they could in a trusted way?
PHAEDRA BOINODIRIS: Agreed. And I think it doesn't have to be just in formal school settings. I think there is a role for museums and for libraries and all kinds of places that are embedded in our community that we trust and that we use in order to extend our knowledge. To be able to lean on them more and more to teach us to ask the questions like: what's the data lineage and the data provenance of this output?
[LAUGHS]
You know what I mean? Just to give us these primers so that we can be better informed about how we can use these models to their best advantage, and again, be critical consumers. Also, just looking, for example at what happened with the fires out in California and just thinking about the role of artificial intelligence with natural disasters, just to understand the multifaceted way in which AI can be an incredibly powerful ally.
And noticing, recognizing, for example, I know there's a lot of research going on right now to use AI-powered drones to fight fires even with high winds, as was the case in California. Right now they're using AI to predict fires and to do public outreach and emergency notification services, which has been incredibly helpful.
There's also the use of artificial intelligence to help people who've been directly affected by the fires to quickly fill out the FEMA forms. Like, you could take a video of this room and an AI could say, OK, that couch back there, I'm estimating this amount of money, blah, blah, blah, and it will quickly fill out a form so that you might be able to get some kind of recompense.
But also, we've got to recognize just how much energy it takes to train these models, just how much water it takes to cool the data centers that power these AI models. So again we just have to go into this with eyes wide open so that we recognize how we can best use this. And then again, what are the limitations? What are the considerations, including the environmental cost?
KIMBERLY NEVALA: And what are some of the emerging practices you have seen, either organizations or public or civil institutions starting to leverage to really, not only get that message and that engagement, but that accountability, that feeling out to folks who feel like, yes, I can have a say at this table and I can understand this. It's not some high-minded mathematical, technical things that are just beyond my ability to sort of perceive.
Have you seen and again, feel free to divide this however you see, both broadly at the sort of civil society level and public institutions, and then also within private organizations. What are we starting to see that works well, even if it's today still just microcosms or small exemplars of where we could go with this?
PHAEDRA BOINODIRIS: Well, there are certain school systems that are really pushing this holistic approach, that are building the bridges between what has been historically fractured silos in order to create those holistic programs. So hats off to them. Just really, really pleased to see that. And I know, because I speak to many of their deans, you know, like, did you pull this off? [LAUGHS] I asked them outright, like, what did it take in order to be able to do that? So I think lifting up their examples, I think is really important.
Also, even on a state level, there are more and more states that are standing up centers of excellence for responsible AI that include government agencies plus academic institutions plus private industry and nonprofits. Sort of collectively coming together as an ecosystem. So that too, I think is very heartening, because again it amplifies the message: we are all in this together. We are all in this together.
And then on the ground, like tactically on the ground. I mentioned this police department. But I do this globally and across industry. And when I go in to help clients, we're very, very the intentional about explaining, this is a holistic challenge. It is sociotechnical. The hardest part of doing any socio-technical challenge is the socio part. Right?
So the hardest effort, the biggest push is going to be focusing on your organizational culture, and explaining, like, what is the right organizational culture to curate AI responsibly? You've got to have humility. You have to have a growth mindset. You've got to have diversity and inclusivity. It has to be multidisciplinary.
And in order to really hit this home, we do applied training. Not go read this book or go watch these videos. But, like, you're going to work together on those diverse multidisciplinary teams with real domain experts, and your domain experts in order to be thinking through does this use case that I'm interested in actually align to my strategy or my mission? What are the unintended effects of this model? What is its primary intent, secondary positive intents, and tertiary unintended potentially negative effects?
Given these potentially negative effects, what are the principles or human values that this organization expects to see reflected in these AI models?
And then given those principles, which are the functional requirements, meaning the requirements that you expect to see built into the AI model, and then the non-functional requirements, meaning the requirements for the systems around these AI models, in order to reflect those principles?
And then that's when they start to realize. As soon as we start to get into, OK, you said that your principles included fairness, it included explainability and transparency, safety. It could be data privacy, et cetera, et cetera. What does it mean to someone buying a model on your behalf? What do you expect to have built for you, given the levels of risk of this use case in order to reflect that principle of yours?
And when we start to do this - again, use cases that they care about - reiterating, like, these are design-- we use design thinking. So this is a human-centric approach to these questions. That's when they start to realize, like, oh crap, I really belong here. Like, I'm not a computer scientist or a machine learning expert, but given my domain expertise and what I know as a police officer or what I know as a social worker, like, hell yeah, I have a seat at this table. Absolutely. Like, none of this stuff that I just described is strictly technical at all. And that's when they start to realize their role in terms of getting this right.
So giving people this kind of practice and this sort of understanding is incredibly helpful and incredibly useful in terms of getting them that hands-on practice of getting it right, knowing what it takes in order to build these models responsibly.
KIMBERLY NEVALA: And has there been…are there any particularly striking examples of, whether this is work that you facilitated directly or that you've seen external to your own direct work, of companies, organizations that have made a really germane and interesting change to the product or service they were providing in a very meaningful way? Whether that was deciding not to move forward with something or just adjusting their approach to the scope, the context, how they deployed a given system, based on that that you think would resonate with folks and just, again, demonstrate the value that can be created by doing this?
PHAEDRA BOINODIRIS: All the time. All the time, with every single one of these engagements. And just to give you an example, there's a company out of California that wanted to explore using AI models to address inequity in educational assessments, traditional educational assessments. There are many reasons why traditional educational assessments are inequitable. There's a lot of research. If English isn't your native language or if you're neurodivergent or you come from a different culture, taking a standard test, you're going to be at a distinct disadvantage.
So they were exploring ways of using AI. And one of the first things that we did with them was to think through, what are the principles, what are the human values you would want to see reflected? Actually they called them student-centric values, and how would you operationalize them? And it was interesting, because some of the principles that they came up with, this think tank, included things like kindness.
So if you're saying that AI models that are going to be used by children to assess their skill sets and competencies have to reflect the human value of kindness, what does that even mean? What does that mean in terms of, you're telling an architect, go make this model be kind. [LAUGHS] Like, what does that mean in terms of what you expect to see built?
And it was just a phenomenally interesting and important exercise to be thinking through, again, bringing in communities who are neurodivergent, bringing in communities where English is not their first language, bringing the community so that we can have their input to reflect on things like, what would you want to see in such a model if we're expecting it to reflect something like kindness?
And again, having that kind of human-centric multidisciplinary approach where you're focused specifically on, what are the human outcomes from the use of such an AI model is powerful, just so much more powerful. Because now you have a better understanding of what to expect in this kind of a model, especially if your goal, your intent is to address inequity.
KIMBERLY NEVALA: Right. Right. Yeah. And again I think this then helps walk that line so you have an opportunity to influence the outcome while also not assuming that outcome and that usage is inevitable or a must do either.
PHAEDRA BOINODIRIS: Yeah.
KIMBERLY NEVALA: And I think that question, though, being able to say no or to say no to aspects of things, it's very hard within organizations. It's very hard for the public at large. My really simple example of that these days is at the airport where they want to use facial recognition or they'll call facial verification at the airport and it says --
PHAEDRA BOINODIRIS: Opt out. You want to opt out.
KIMBERLY NEVALA: It's optional. But folks really don't feel that it's optional. When you tell them, no, like walk up and say, I don't want to do that. And someone after me said, I really didn't think I could actually do that. I said, no, it is actually optional and sometimes faster, weirdly, to walk through in that way.
So I think giving people permission to not…you know, how do we do that to make it OK to say no? Make it OK to be the squeaky wheel, make it OK to be the customer or the user that pushes back? Is that also a mindset shift in how we look at it and think about that kind of feedback?
PHAEDRA BOINODIRIS: It goes back to what I was saying about, what is the right organizational culture required to create AI responsibly? And that first premise about humility and growth mindset has to include giving people psychological safety so that you can push back. There can be concerns.
And then for those who are going to be accountable for things like AI governance, part of their effort is standing up all these processes, including gathering feedback from your community, from your user base. What concerns do they have? Are there whistleblower protections? For example, let's say they're worried about expressing their concern about a model because they're worried about losing their job. What kind of whistleblower protections would you have in order to make sure you're giving them the feeling of psychological safety that they're going to need in order to give you the kind of impact that you might be looking for.
All of it. All of that is really core, and, again, why that human-centric approach, that design thinking exercises I was describing that says, no, we want to hear from you, what you have to say with your domain expertise, your understanding of disparate impact, for example, is crucial in terms of getting this right.
KIMBERLY NEVALA: Is there a key element, as we start to wrap this up here, something that we haven't talked about, I'm not asking about, people broadly are either overlooking or underestimating? As you've done this work and you have many, many of these conversations, are there a few or even one element that always pops up in your mind as, man, we really need to -- I wish people would understand this or I wish we could reset that?
PHAEDRA BOINODIRIS: I think people don't understand how hard it is to get right. I think that is key, and again why most of this conversation has been about education and about literacy and about culture. People just don't understand what it takes to get this right.
And I think now that we're shifting into this the next stage, which is agentic AI, where you have an AI that acts as an agent on your behalf. It might take the input as an input, the output from another model. You have fewer humans in the loop, fewer potential for human oversight for these different models. It's even more critically important that we talk about things like accountability. What does it take to get this right? Having the right AI governance in place.
And I can also tell your listeners that what we've been describing, what we've been talking about, like, this affects everybody. This affects all organizations. This isn't just organizations who are using AI for their own purposes or for malintent. These are organizations that have truly the best of intentions with respect to how they want to use AI that can end up inadvertently causing individual societal reputational harm.
And that's the part that I think people don't-- they don't understand. And I'm saying the best of intentions; which, again, is why it's important to understand, what does it take to actually build this in a way that is trusted and represents our intent?
KIMBERLY NEVALA: Now we could spend a whole other hour or many on topics like agentic AI. But you've been doing this work for a long time. And as we started talked, you talked about something you took from gaming, which is the idea and the need to really have fun, right, to be engaged in a fun and interactive way. And your work, your speaking, even when we're talking about some of these topics that are tough and hard, is just refreshingly positive.
So what just continues to fuel that hope and that joy for you? And what would you like folks to really focus on in their own lives, in their own work, as this progresses forward?
PHAEDRA BOINODIRIS: I think that I have always been very much an optimist. I'm an optimistic kind of a person. Even though I work in responsible AI, and I'm sort of hammering about risk and disparate impact, I know that I think when there's an intentional approach towards giving people the seat at the table and explaining, like, no, no, no, this conversation is for you, and then demonstrating that in a way that is truly inclusive, I think we're going to see that 85% number go way, way down. Which I think will benefit us all, including those who lead big corporate organizations or government institutions and are trying to figure out where to put investments and money.
So additionally, I mentioned, as I said, the stories out of California and how AI can really be used to solve some tremendous problems of our time, I mean, huge, huge challenges that we have. But again, it goes back to voicing and consistently messaging and communicating how critically important it is to have that holistic approach in the way that we've defined it here on this show to teaching the subject of artificial intelligence.
KIMBERLY NEVALA: Well I think that's a great insight and a great direction for us to all continue rowing in, if you will. So thank you so much. I so appreciate your time. I appreciate the work you're doing, broadly on behalf of, in fact, all of us - or the rest of us, as your book so cogently calls out. So thank you again, both for your time and for the work you have and continue to do.
PHAEDRA BOINODIRIS: Oh, the pleasure is all mine, Kimberly. I am so thankful for your thoughtful questions on this somewhat thorny subject. I do appreciate it. Thank you.
KIMBERLY NEVALA: If you want to continue learning from thinkers and doers and advocates such as Phaedra, please subscribe to Pondering AI now. You'll find us on all your favorite podcatchers and also on YouTube.
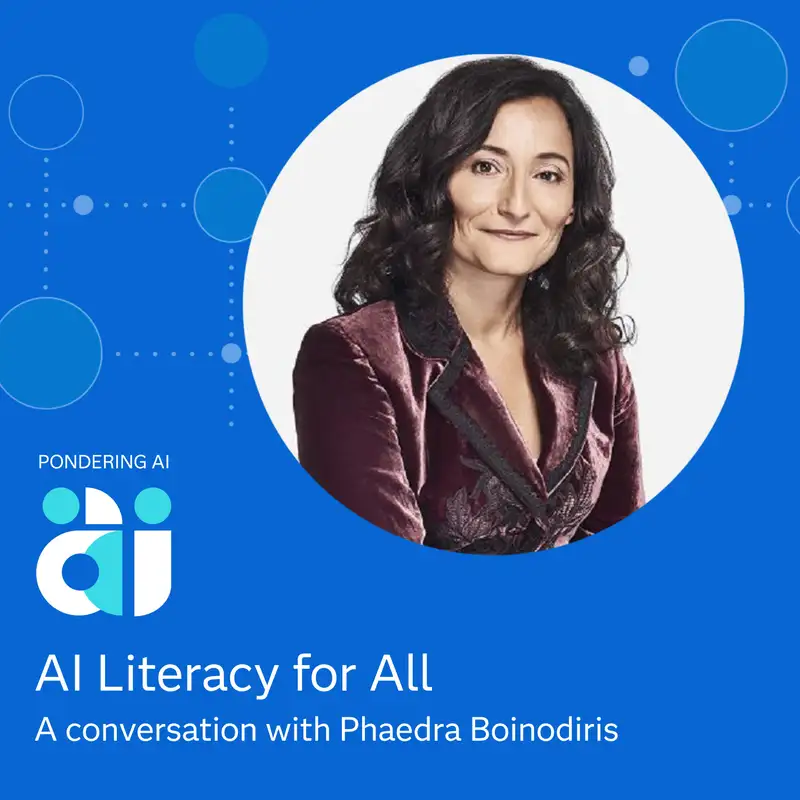