AI Myths and Mythos with Eryk Salvaggio
KIMBERLY NEVALA: Welcome to Pondering AI. I'm your host, Kimberly Nevala.
In this episode, we are so pleased to bring you Eryk Salvaggio. As a self-proclaimed AI critical artist who works with AI, Eryk examines the critical or creative misuses of AI and the myths animating AI tech design today. He is a hacker, policy researcher, and new media artist who also serves as a research advisor for emerging tech at the Siegel Family Endowment. And he is also an adjunct professor at RIT.
We're going to be talking about current AI narratives, the nature of creativity, and the role of AI in creative endeavors - if any. So welcome to the show, Eryk.
ERYK SALVAGGIO: Thanks so much for having me. It's great to be here.
KIMBERLY NEVALA: Awesome. Now, for those who have not had the pleasure of interacting with your work before today, can you tell us a little bit about how you came to be solely planted - or squarely planted - at the intersection of art and technology?
ERYK SALVAGGIO: Yeah, I've been camping out at that intersection for a long time. I got involved in internet art in the 1990s. I was a teenager, and I was very excited to find out information about my favorite band at the time. And turned out they were doing a show at the Walker Art Center, which was running the first sort of internet art focused exhibition online. So that's where I went. That was the first website I went to.
So to me, immediately, it was sort of technology was a place for play and experimentation. But it also brought me into thinking about, well, how can we use these technologies and why aren't we doing all this cool stuff all the time? Because a lot of this stuff that started happening as I spent more time online as I realized, oh, we actually could be doing things in much different ways. So, I started thinking a bit critically about the way we use and build technologies too.
So fast forward about 25 years, and these questions are as relevant as ever with AI, Artificial Intelligence, generative artificial intelligence in particular. And so those are now places where I'm spending a lot of time exploring and bringing that kind of mindset of tinkering with while learning about the systems. Not just in a technical way, but in a kind of social and cultural and economic way as well.
KIMBERLY NEVALA: Now, certainly the use of myths and metaphors have been a long-standing tool, if you will, to help promote or drive certain beliefs and behaviors and to aid in understanding of complicated topics. But you very much argue, and I'm going to use my own terms here, that a lot of the myths around AI and generative AI in particular today are really more tools of misdirection - again, my words, not yours - rather than true public enablement or education. Can you talk a little bit about that and why it's so important that we face or critically evaluate these myths and metaphors head on?
ERYK SALVAGGIO: Yeah. So my general feeling is that when people are building technologies, they explain the technologies and they explain the tasks that they are setting out to do in very specific ways. And oftentimes, these ways are quite narrow, because the scope of the project is to focus on getting the thing done.
But when we share those myths around the technology, something happens. We kind of lose track of what the technology is actually doing out in the world. We kind of refer to it through this way that designers have made shorthand to think about how they're making something, or the ways that they have been built, and the kind of logical connections or frames that have been deployed in making sense and explaining this to, again, the builders and the designers of these things.
But I argue that we need to know what this tech really is and what it is really doing if we want to make informed decisions about how we use it and whether we use it at all. And sometimes these mythologies don't really translate beyond the kind of design conversations that are taking place. So it's really important to think about how are these myths shaping our understanding as not designers, assuming we aren't designers. It's also useful for designers to be thinking about this too. Because these myths create this common reference point that can be useful, but they can also be kind of limiting and constraining. And if we want to reimagine a technology, it can actually be really helpful to break out of these frames too.
So I'm trying to think through a lot of the kind of storytelling we do and the common references that we have that come from this orientation to building something and getting it built and getting it deployed. And how they stop making as much sense and sometimes even create or perpetuate certain harms when they're actually deployed out in the real world.
There's no real reason that we have to adopt these myths. We can think about them differently. And a lot of what I'm trying to think through is how could we do that? What are the different myths that we can create? As an artist, I get to lean into that a lot. But it's also really helpful, I think, to articulate what those myths are in the first place.
KIMBERLY NEVALA: We're going to spend a fair bit of time today talking about creativity and the nature of creativity and the agency therein. But I think it's helpful, because I don't think any of these narratives function in isolation necessarily, to just quickly highlight the three categories of myths that you had noted in what I consider a fairly seminal article. And they were control, intelligence, and future mythologies. So talk to us a little bit about what the control myth is and why this might be problematic.
ERYK SALVAGGIO: Yeah, so the control myth is just basically a myth of control. That one's pretty straightforward. It's the idea that we actually control these systems to a greater extent than we actually do.
A lot of these are kind of based on probabilities. And they are not always - as we are increasingly, I think, becoming aware - they're not always factually accurate. They are essentially generating a statistically likely outcome. So, if we're using these to look up information. Information retrieval is a common use of generative AI. But you're generating a response. You're not necessarily digging into a response. And so it's really important to articulate that all of these systems are kind of navigating the probability of a correct answer against what we might assume is kind of a factual reference. They're not making factual references. They're making a kind of guess to be really straightforward about it. And so if it is accurate, it's actually kind of a coincidence, because it's not referencing anything. There's no reasoning occurring in these models to figure out: is this true? Is this not true? Instead, it's extrapolating from the words that we are using to ask the question.
So, there's different types of myths that go into control. And this factors into a lot of things, a lot of different frames. For example, the idea that we can have control over our time by using these systems is, I think, one of these big myths. Because a lot of the time that we save sometimes we spend adjusting or readjusting the materials that are given back to us.
And there's also a question about what are we saving time for? A big thing, I think, is sometimes we look to these to automate tasks that assume that the goal is the product. A classic example is a government accountability report being written with generative AI. That actually completely neglects the purpose of saving time. Because the time is meant to be spent in thinking through what is being written. And if we're not doing that, if we're just asking ChatGPT to write up some things that we're sorry about, that doesn't really feel very helpful in changing governments. It becomes this kind of weird exercise in the illusion of accountability.
So, this factors into the control myth because it's kind of about how we are using them and what we actually are doing when we do them. It's not real, right? It's not the real work. So that's the control myth. And tied to that is this productivity myth that I'm talking about as well.
KIMBERLY NEVALA: And how does that relate to or exasperate what you call the future myths? Which is predicated on this idea that these systems will scale perhaps indefinitely, perhaps ultimately into something that looks like AGI. And that there are emergent behaviors that we should expect and, in fact, perhaps proactively plan for, whether or not they appear imminent or otherwise?
ERYK SALVAGGIO: Yeah, yeah. So I work in, sometimes I'm surrounded by people who are working in, policy questions and we have a long history of this. Going back to some workshops I would run that would say, OK, there's these biases in these machines. And how do we work on these problems, very real problems that are affecting people in very real ways, immediately?
And oftentimes we would get into this conversation that would be like, oh, well, the AI will get good enough and it'll solve it. Initially, it was like a ha ha: the AI is going to become super smart and solve all our problems. But then it started becoming kind of like, no, really. People's faces were no longer smiling after they said that. And I think this idea of leaning too heavily into this kind of future scenario of machines that we don't yet have - but are expected to have - is kind of a bad way to solve problems.
It's leaning into a kind of myth that relies on a couple of things, one of which is scale. This idea that the more data we have, the more accurate these systems are going to become or the better these systems are going to become. And what we're actually sort of starting to see is that this is stalling. It's starting to hit some roadblocks.
But even when we do scale these systems, it's important to ask, what are we scaling? Because they're still based on probability. And so they might be able to work with language in a more sophisticated way. They might be able to transform a very long podcast transcript like this one into a very short memo. But it doesn't mean that it's necessarily getting better at understanding or in making inferences about the things that are being said.
So even summarization, what it chooses to omit and include is, again, it's probabilistic. So you can't really be trusted not to, or it can't be trusted to, include the most relevant aspects of the conversation. Or what the people in a conversation decided amongst themselves was the most relevant part of that conversation. We're relying on this sort of shorthand being made that's still probabilistic. And we're saying as it gets more and more data, it's going to get better and better at doing these things. But again, this isn't really the case. Factuality is not necessarily built into these systems. But hallucinations, so-called hallucinations are. So, if you want a lot of variation in your text, it's going to make things up. That's just how these systems work.
The other thing, and this is a great point that's made in a paper by Dr. Abeba Birhane, if anyone's looked for this work, is that as you scale up the systems, you scale up the junk in the training data. So if you have racist, misogynistic, or violent terms in these words or just really the nasty stuff, the more of the data you have, the more language you have, if you're not careful about where it's coming from, if you're not curating it - and spoiler alert, we aren't - then a lot of this stuff gets bigger too. So you don't eliminate that stuff by going bigger. You actually just get more of these problems.
So scaling is a kind of myth that the more data we have, we're going to solve more of these problems. And unfortunately, it's a myth that's pretty prevalent in industry. But I think we're starting to see, like I said, companies, the signs seem like they're kind of moving away from this. We're starting to see things like OpenAI referring on deeper levels of language generation to check on the language generation. Which they're calling reason or reasoning capacities which deserves another article on its own mythology.
But this is the idea of scaling, and it's a common myth that we have. If you ask most people, they say, well, the more data we have, the better these systems are going to be. But that's not ultimately true. Or it's a theory that has not yet been proven, and I don't think we should be relying on it to solve really complicated and urgent problems.
KIMBERLY NEVALA: Yeah, and it creates what I think is sometimes couched as a bit of a catch-22, which I think is a false problem. Which is, well, if you don't like the way the systems work today or the way that they're operating, then you should be providing more data to them to drive to an outcome or a usage or a level of capability that you believe is appropriate. And so you get into this really interesting bit where if you hold back from participating you are somehow then also responsible for the lack of capability or growth in these systems. Whether you believe those are beneficial or not long term.
ERYK SALVAGGIO: Right. And what if one of the problems that you are dealing with is data rights? Which a lot of artists are. For a lot of artists, this is the problem. So AI is not going to solve that problem by using more of their data. So these kinds of things do introduce some real tensions.
KIMBERLY NEVALA: Yeah, certainly, and I saved this one for last for obvious reasons, is you've grouped what you call the intelligent myths. So learning and creativity. We'll talk a little bit more about that in detail as well.
But one of the interesting points that I think you articulate so well in your work is that all of these myths - whether it's around control or the presence of intelligence or the ability for future capability - are somewhat predicated on establishing an equivalence to humans. So there's this comparative narrative that happens so that we're trying to establish these systems in a way that they're viewed as having human-like capabilities and therefore social value. And that's problematic in and of itself.
Can you talk to us a little bit about why these narratives which drive towards this view of equivalence are perhaps leading us astray?
ERYK SALVAGGIO: Yeah. I mean, to quote a recent post from Emily Bender, part of it is that the metaphor is inherently dehumanizing. If we are comparing ourselves to machines, we are already simplifying significantly what we think humans are capable of. And that in and of itself is a kind of harm that we are doing to other people, but also to ourselves.
The other issue is that when we talk about things, I mean, artificial intelligence is in and of itself a contested term. But this frame leads us to kind of an overreliance on shorthand in spaces of policy that compare this new type of machine, which is not all that new, to human beings. And almost as if they are then therefore deserving of human rights.
So I've seen some arguments, very real arguments made in policy spaces, about a right to learn. That artificial intelligence, because it is like a human, this is the argument, has a right to learn the way a human does. And so the idea is that, well, you can't deprive a child of a library. So why would you deprive an artificial intelligence system of the sea of training data that is the internet?
Part of this is actually it's not how the systems work themselves. The idea of learning is very different in an AI system, because the AI system doesn't exist until this data is given to it. There's a framework for building a model, but the model is the result of that data. So it's almost like saying, well, a child emerges from libraries. And that's not really the case.
What happens is humans go out and select this training data, or they point a bot in the direction of the training data, which I would argue is a curatorial decision. And the bot gathers all this data or scrapes the web for this data. But this is a human decision about how they want to build a machine. And once they decide how they're going to build that machine, the machine then finds patterns in that data.
And this is really, I think, a very weird thing to compare to a human child's learning experience. There's obviously, with all of this stuff, there's a way of saying, well, if you zoom out, it's kind of the same thing. But that's a fallacy. This fallacy of scale that kind of comes across in a lot of AI is, well, if you look close enough at the human brain or you look far enough away from the human brain, it looks kind of like an AI system does. But that's kind of like saying, if you squint at a tree, it looks like a person. Therefore, we should go around chopping down people.
We have to be careful about the terms and the metaphors that we use so that we don't confuse the metaphor for what's actually happening. And learning is a great example of that. Because if we give the AI human rights, we say, OK, the AI can go to college and should deserve a free education. Well, a lot of human kids don't have that. So why not focus on the real value of social investment into education in the same way that we're advocating for the rights for these machines, which are really not doing the same thing?
KIMBERLY NEVALA: It also seems to be it's a nice way, in some cases, of shifting liability and accountability while we're also projecting some unwarranted confidence in the competency of these systems, I suppose.
And I think I had mentioned to you before, I saw this really interesting article that was, it was a post, I think on LinkedIn, that was really railing against people being biased or prejudiced against AI. And again, I have preferences for different tools for different things. But the use of the term prejudice or bias struck me as a very human framing and also invites us to ignore the limitations or maybe paper over a little bit, blur the edges, of why context of use matters. And why the scale here matters when we're talking about a human drawing inspiration from something versus a machine.
ERYK SALVAGGIO: Yeah, and there's a couple of forms of biases about AI. I think a lot of very, the most adamant pro AI people say, oh, well, you shouldn't be biased against these machines. Or they compare it to being biased against certain groups of people.
But there is also other types of bias, which actually I think are also important to acknowledge, which is a favorable bias towards AI systems that makes us more inclined to believe them. We think of them as calculators, as if the texts that they generate is objective. A lot of people will lean into the things that a machine says with a lot more trust than they would a human expert with a PhD in the subject. And I think that's something if you're concerned about the nature of expertise or who we trust or what we trust - which is, I guess, more a better frame of the question - then understanding that type of favorable bias towards AI is equally important.
And sometimes this bias question, I think, misses the mark a bit. There was a recent paper about folks preferring AI generated poetry to human written poetry. Part of that is, well, how are we assessing that as value? Is value in an artwork really just that more people like it? That more people understand it at first glance? Is that the benefit of a piece of art? Or an AI image. Some people say, oh, I like that image. But why? Why do they like it? And what are they thinking about? What does it make them think about? What are they comparing it to?
So if people prefer an AI image that really doesn't surprise me. They're designed to be preferred. Aesthetically, you might like them more, but what are they really doing? What role are they serving in culture? That's, I think, a more relevant question. So this kind of AI bias conversation I think is an important one, and I wouldn't want to dismiss it. But it's important to acknowledge that there's two sides to it as well.
KIMBERLY NEVALA: And so this is great on ramp into talking about the nature of creativity and the creativity myth in and of itself. One of the things that you said that I'll just pose back to you is you mentioned that you're often - or that the question of ‘is the machine creative?’ or ‘can a machine be creative?’ is not a particularly helpful question when we're addressing these types of issues. Why is this framing, incorrect - I don't know if it's incorrect - maybe unhelpful is the better word?
ERYK SALVAGGIO: Because there is no machine that has not been somewhat involving the use of a human being, which is a weird way to frame that. But a human being is making a creative decision to use the AI to do something. This is something that oftentimes gets dismissed by the kind of anti-AI crowd, which is to say, well, you can't be creative with an artificial intelligence system. I think you can be. And I like to think that my own work proves that, but the jury's out.
But I think it's mindful to say, well, where is the creative process in using these machines? Because the creative process is not in the machine. The machine is not creative. The machine is following a set of steps and it cannot stray from those steps. It cannot reevaluate the previous steps that it's done in order to change or transform some meaning according to its own desire for personal expression. In other words, it's assigned a path and it follows that path. It has a structure. The information moves through that structure, and it does not transcend that structure. So in a way, it is a mechanical, probabilistic process.
That is very much different from creativity, which is the ability for an artist to look at something that they are doing and think through what they can do to challenge themselves or transform the type of task that they are engaged in, in order to reimagine it. And so one of these things is as an artist in creative AI circles, we're talking about the process as if the process is only there to arrive at a product. So what do we say? We say AI makes a pretty picture and therefore it's creative. But that picture is not really creative if it's only following a set of rules to come into the world.
So asking whether the machine is creative is really missing the mark, because there's just no capacity there. Rather, I think it's interesting and important to rethink what we mean when we talk about the creative process and the artistic process. To say, well, it's actually -- what is it? It's expressive.
When you sit down to listen to a piece of music, you're oftentimes trying to hear someone who you have sympathies with, that you feel like you've been in their place. You've had heartbreak. Or if you're looking at a work of art, you want to experience something that is transformative. Not just another thing on the Instagram feed, which is designed to look like all the stuff you've liked before on the Instagram feed. When we seek out art, we're actually seeking out something radically different than what we've seen before. We want variety. We want transformation.
And artists want to work in different ways with their medium. They don't want to make the same thing over and over again. And so that, I think, really gets to the heart of what it means to be creative, is to think about: what have we seen? What do we want to do differently?
How do we reimagine our relationship with our tools and with our artistic practice? Whether that's the canon, the other paintings that exist out in the world or the other movies or songs, but also our own ideas. What do we want to do differently? What do I want to do differently as an artist that other people aren't doing but also from what I've done in the past myself?
So that is the question of the creative process that I think gets to the heart of the issue around creativity and AI in a much more productive and, I think, clarifying way. I think it actually points to more possibilities for AI in a way than just asking whether the machine is creative which I think is a kind of a closed door. And it's one of those metaphors that kind of, again, to focus on this idea of dehumanizing us, it restricts us too much.
KIMBERLY NEVALA: Yeah and as you were speaking, I was starting to grin to myself. Because I've got a good friend and we like to go to modern art museums. Because, to be frank, I would say I don't like most of it. But I get unholy glee in the active lambasting of why this is important or why that is art. And then every once in a while, there's that thing that strikes me and I'm like it speaks to me. And I don't know why.
But for the most part, I have to say, if I was just going through a style of art that I prefer, I probably don't get as much - I shouldn't admit that out loud and on video - unholy joy, if you will, from admiring those things. It's a different sense or a different type of satisfaction I take from doing that.
So again, this then leads me to wonder, in a very basic sort of way, if what we are so enamored with is, as you said, we didn't really ever consider that machines could create these outputs. And so it's the fact that they can. It's more the how than truly the outcome that we're impressed by. The fact that it's just a capability that exists.
ERYK SALVAGGIO: Well, what you say I think actually gets to the heart of something, which is that when we are looking at a work of art, there's a couple of ways to engage with it.
One of them is to say, do I like this? Do I like to look at this thing? And so there's an aesthetic appreciation that comes from that. But there's also, well, what is this person doing? What is this person saying? Why did they make the decisions they've made? And so a lot of that stuff can be easy to mock or dismiss.
This just happened. A banana got taped to a wall and it's selling for $6.2 million. But this is the art market, which is very different than, I think, our personal experiences of creative expression and meaning and meaning making. Which is, I mean, there are ways of engaging with that banana. I'm just going to say, I don't like that banana piece. It doesn't strike me. I'm not impressed by it. But I can ask questions about it. I can say, well, why did he make this piece? What decisions went into this? And I may not be satisfied with those answers, but there might be other pieces where I do scratch into that surface and I say, oh, OK that actually makes me think a little bit about this thing or I actually I dig that. Or I hate this, but why do I hate this? What makes me hate this piece of art so much?
And those are questions that we can ask about a kind of human expression where someone has made these decisions in a way sometimes to intentionally provoke us or intentionally comfort us. Whereas, again, when we compare this to the model that is going through the series of steps to say, a cluster of pixels that appeared here is most probably going to appear next to another cluster of pixels that goes over here. And I don't know what this area is, but I'm just going to fill it in with something that's kind of blurry.
There's this kind of regimented process that it goes through and a series of steps. And diffusion models really are a series of steps, just refining pixels, and the likelihood that certain pixels are appearing that match your prompt. That's very different than navigating ways of thinking about the meaning that is being made in the image and interpreting that image as a viewer.
So AI art can generate a kind of certain thing and we can have a response to that certain thing. But I think more and more, we're responding to that thing as AI. We're not even really thinking about it as what it's saying about the world. We're thinking about what it's saying about the training data that informed the shape of the image. And so I think there's just very different experiences between the two of them.
KIMBERLY NEVALA: Yeah. In some of your writings - which are gorgeous, I will say.
ERYK SALVAGGIO: Thanks.
KIMBERLY NEVALA: A complete fangirl here. So I'll just say that out loud for everyone for fair warning.
But you had said that with generated media, you get images that look like images or music that sounds like music. And perhaps if we look at a generated image, we should consider that as an infographic. It's a representation of data. Which, I don't know if that changes how we think about it or whether we can appreciate it or not, but it was a really striking turn of phrase. I think that punctuates a lot of the points that you're making there.
ERYK SALVAGGIO: Yeah. I think there's an important emphasis there. So this came about when I was looking at sound generation and music generation in general. We can get… I'll try to spare too much technical detail here. But really, what these systems are doing is they kind of create something at random. And something else, another model, is looking at that thing and evaluating whether it is actually the thing that has been requested.
So I write a prompt, I want a sad song, or I want a sad picture of a person sitting by a tree. A bunch of pixels get generated, and then this thing looks for sort of clusters of pixels that look like a tree, looks like a person, looks like some representation of sad. And if there's a certain enough threshold is met, we see it. And if a certain threshold isn't met, it gets sent back and some other random clusters of pixels get thrown together and refined over and over again until we see it.
And so really, what does that mean? What it means is that we are not exactly looking at an image, per se. We are looking at an image that is designed to be plausible. We are listening to music that is designed to be plausible as music. In other words, we're listening to music that sounds like music.
Now, it's also music, and I'm very skeptical, I don't like, ‘this is art, this isn't art’ arguments. Because, listen, I'm an avant garde artist. I worked on the internet. I worked digitally. I've heard over and over again what is and isn't art. And I think again, it's too much constraint to say something is art or isn't art. It's art if we want to interpret it that way. But the question is, what kind of art is it, and what is it actually saying? What is it actually doing?
So we can create AI generated music. It is music but it is music designed to sound like music. Which is a different intention than music that is designed to express a feeling or reference a time period that we're nostalgic for or reference no time period in order to arrive at something really futuristic sounding. What we're listening for is something that based on the data available to the model represents or references that data in a plausible way. That the outcome is closely enough aligned to what has previously been encountered in the training data in order to pass as something that might appear in that training data. So it's designed to sound like music.
And I think if we engage with that, if we engage with pictures as things that are designed to look like pictures or sound like music, we have a different relationship to them. And we understand, I think, how algorithms and algorithmic products more generally reflect data as opposed to real human expression, real human experience, or experiences of the world beyond the human. There's all kinds of other ways of experiencing the world. So I think it's worth taking that in. and considering when we look at these things, what is it exactly representing
KIMBERLY NEVALA: And I'm going to take perhaps a bit of a tangential turn but I think it's connected. I don't know that it's completely tangential.
You also talk about the difference between a data set and something that's like an archive or a curated archive. And it relates a bit, I think, to what you were just saying, which is what is really the context and the intended use? And then what can those different collections of information, what are they intended to do and for what purpose?
And I particularly like your framing of this question, as not about trying to understand humans - or AI as a sort of a proxy for humans - or humans as a proxy for AI. But by really looking at the contrast, it tells us a little bit more about understanding ourselves. Not as something to be replaced or replicated or vice versa, but just about how we work.
So tell me a little bit about how we should think about or frame our understanding of what a data set is: whether it's going into a generative AI model or any other kind of predictive or prescriptive algorithm, for that matter.
ERYK SALVAGGIO: Yeah. So I've been doing a lot of work with archives. A lot of my work is based on archives. And a lot of my work is also, because I work with AI, working with data sets.
So I've built my own data sets out of my own images, for example. And so I've learned how we have to structure data sets. And I've gotten involved in thinking through what a data set has to be in order for it to work for training a model.
I've also worked, as I said, with archives. And there is a distinct sort of difference in the state of mind. And I think a really important difference, because when we're thinking about an archive we're really thinking about who its audience is, and the audience is human beings, A. And what we are doing with it is preserving contexts of memory.
In other words, we have a bunch of photographs, we have a bunch of objects. But they are not being preserved simply to be analyzed as objects or to be looked at to figure out which colors go next to each other in this historical collection of photographs. It's being used to say, well, this is a moment in time. And the people in this photograph have a connection to these objects and to this story and to this place and to this time.
And so what happens when we go to an archive is that these images, these objects, they elicit memory. They invite remembrance. Depending on the archive, this memory could go from really sort of fundamental to really almost sacred. And all of that is centered on this idea of care. We curate, and literally the root of curate is the word for care, we care about the things. We care about, the context of these things.
And it's imperfect. We often say, well, humans make mistakes too. Humans are biased, and humans do all kinds of bad things. Well, yes. But the difference is that we can correct each other. We can intervene. We have a way of saying, actually, this object that's in your museum probably shouldn't be there. It was taken from other people who didn't give you permission to have this thing. So let's think about how we deal with that. Or these photographs are taken with a real bias. There’re real problems in the way you're representing these people. And so we can address that. We can think about how we show those works. We could contextualize it. We can not show certain works at certain times if they're not deemed appropriate.
Whereas when we're talking about training data, and specifically the ways that we've been building training data sets up till now, we are just grabbing stuff. We are pulling books off the shelves and throwing them into a giant pile. There's no categorization, there's no sorting. We're letting the machine sort it.
And a lot of this, particularly when we come into image data sets, there's a lot of problematic content in there. I've seen all kinds of stuff, again, referencing violence and misogyny. It's very challenging to talk about, but there's child sexual abuse material in these training data sets. The largest training data set for generative AI, LAION-5B, had to be taken offline for this very reason.
And that to me is the opposite of care. There is literally no curation. Meaning no one has gone through this stuff and said, yes, include that. No, don't include that. There's just an absence of care in the sense of what are we even going to point our scraping system at? We have a bot that goes out and scrapes the web. Where are we pointing it at? Well, we're pointing it in the direction of as much data as it can get based on this scaling myth. And what we're losing is any kind of sense of intervention into whether or not that data should be there in the first place, and any sense of how that is going to inform the outcomes of these models.
So not to go on too long, but one of the research projects that I've been able to engage with as a research fellow with the Flickr Foundation was the type of material that ends up in the data set that ends up shaping the kinds of images you get from certain prompts.
What I discovered is that there's this whole history, this archive that's been gathered by the Library of Congress to show, well, images during the period of colonization in the Philippines. And it's contextualized by the Library of Congress. They're telling a story. They're saying they're not saying this was a great thing.
But when you prompt image models for something called a stereo view image, which is this kind of media format that was very popular at the turn of the 19th century, kind of the equivalent of 3D glasses. It was this thing - you'd hold it a foot from your face and would sort of float. But the images that were often used and the stories that were often being told because of the time is this story of that colonization effort by the US, in the Philippines. So it is informed with a very particular view of people who were living there, and a very particular view and a very biased view of that occupation.
And so when you generate this image for something completely unrelated to the Philippines or completely unrelated to America, you get this kind of weird colonizing or colonized imagery that should make us second guess like, well, how did that get there? And why is it there and why didn't anyone - is that really what we want? - when we ask for this unrelated keyword of stereo view images.
It's not just a problem in sense of making better image models. It's a representation issue. It's about how we think about other people and how we think about our own role in the world and our own history. That we are kind of normalizing it when we just show it over and over again without thinking about it. Well, we have images that are making reference to victims of the Holocaust, because we've of for a series of photographs, which is also something that comes up.
These kinds of things really make me sit kind of uneasy about what the history of these images are and how we're losing that history and that context. And then generating images that kind of flood out a lot of real archival images. That's a real problem too.
So it's a complex problem, I think. But really, what I want to see more of is a kind of care and attention paid to what we're saying yes to and including in these data sets. And also understanding how those inclusions get filtered through the model, which is always compressing. There's this kind of central tendency or the most basic, most representative pattern of what's in the data for any given word or prompt. I've talked for a long time, so I apologize.
KIMBERLY NEVALA: No, I think it's so important.
And it's gotten me thinking more about AI in this context as almost a memory distortion device, but also as a continuation of this idea that we have of data as a sort of an inherently neutral nugget. And so it's disintermediated from the experience, from attribution, from context, from all of those components. And so there used to be that old trope which says all data is neutral. It's how it gets applied or how it gets viewed. And maybe not.
But I think that concept is being used in a lot of cases and will generate actually real harm and sort of perverse outcomes, intended or unintended, by a user. But also somewhat feeds into even some of what we see now in the regulatory and legal frame. Which is as long as I don't talk about this as a piece of specific material that's copyrighted or which has certain implications, certain rights attached to it - I think you call it the image to data translation frame - all of a sudden, there's fair use. Because, well, it's just this one small piece and therefore it, for some reason, the scale of it, we seem to think it's OK to utilize or pilfer these elements, as long as we take a small bit from a lot of people in this sort of way.
So somehow this idea of scale sort of desensitizes us to that harm. Whether it's very discrete harms, like protecting IP and challenging folks' rights to their own creative products, or whether it is disintermediating us from understanding the relevancy and the harms we may be implicitly supporting or leaning into by using these tools.
ERYK SALVAGGIO: And things like the rights to our own likeness. It goes beyond the art and copyright question.
I think when we think about it in terms of our right to our own likeness, is my face in the data set that is being used? And if so, how is that face going to appear in other ways? What aspects of it? I think it's absolutely reasonable to say that someone could be uncomfortable with being in a training data set. That having their photographs that they've taken of their families in a training data set.
And what you point to, I think, is really, really true. And it's something that I encountered initially around this idea of data surveillance. That, well, some of these social media companies are collecting so much data on so many people that why would anyone look at your data? There’s almost this idea of - Orwellian gets overused these days - but it is this Orwellian kind of view that, mass surveillance is anonymity. That all of us are being surveilled and therefore, nobody is going to be bothering to look at us, is a weird way of thinking about the types of data that we want to protect and the types of information about our lives that we want to protect. And the same is true now, I think, increasingly that logic is being applied to these data sets. And you're right, it is about artists and it is about copyright.
But it is also, I think, just a data rights question. If I want to share a poem, if I want to share a picture that I've made, there's definitely the sort of copyright issues. But there is also just a personal issue of, well, why do I lose that right? Why do I lose that right by publishing it? Which has always been the bedrock that once you publish it, it's yours. Now once you publish it, it's not yours.
That's a really, I think, problematic reversal that where for some reason, we're allowing scale to transform. That if I copyright something, it's a small thing. But if somebody takes billions of copyrighted pieces of material, it's absolutely nothing at all. That's not a big deal. Go ahead.
Because so much of that - this is a problem, I think, with the idea of the archive versus the data set too - is we tend to look at the data set as an object rather than the composite parts that make up that object. Whereas in an archive, yeah, we talk about the archive. We might go to an archive. The archive might do certain types of work. But when we go to an archive, we're there not to just look at the shelves. We're there to look at the things on the shelves. And with a data set, we lose some of that. We kind of just say, well, it's a data set. And this happens over and over again. And it happens when archives become data sets, which has also happened a lot.
Flickr is a great example. These training data, they made a training data set that was representative of Flickr, which is a huge photo sharing website. It was designed to say, well, what's going on with Flickr? Here's a representative sample. If you want to do some research and tell us what's going on with Flickr, feel free. But then, because it was a data set, people stopped looking at the individual components and started saying, well, how do we calibrate other models so that it mimics what this data set does? Or how do we build a generative model that can create from this data? And all of those individual pieces, including whether or not the photographers themselves ever said, yeah, you can use my image for x or y purpose was kind of thrown out the window and it was just sort of assumed, well, it's a data set. We can use it.
So this is another kind of problematic transformation of the archive into data sets, in a really practical way. Which is our entire relationship changes too when we stop looking at it as trees and just look at it as one big forest.
KIMBERLY NEVALA: Now, there's also a natural tension, which you've called out as someone who is concerned about the harms or misuses. As well as very cognizant of the ability to use these in ways that are really interesting and creative and as part of the bigger scope of work.
How are you today, I mean, outside of reconciling the tension between maybe by virtue of using and engaging with these at all, as you said, you're somewhat complicit in their perpetuation. But to not use them means that you also can't understand or think about how to mitigate the harms.
ERYK SALVAGGIO: Yeah, I mean, a lot of this is just personal for me, because this is how I've thought about understanding technology is I need to know how it works. I need to know how to manipulate it. And I want to know how to use the technology. But I also want to know how the technology is using me. What is it asking me to do? What is it asking me to give up? What is it allowing me to do? What is it saying I can't do?
These are the types of things that become clear when you use the tool and become fluent in the tool. And it becomes a really useful avenue for critique. Even if you're using the tool. Even If I am paying OpenAI for generations or whatever. Now I'm an artist who's critiquing the tool but I'm paying the tool for the right to critique it. There is a real complicity there.
And a lot of people will say, well, let's just not use it at all. The only morally correct way is to say no and refuse to use it all outright. I am sympathetic to that in many ways, but it is not how I would be able to work. And I think that the type of critique that I'm able to do I hope people get some benefit from. But yeah, it is oftentimes uncomfortable.
So one of the things I'm trying to do is navigate in my own practice. Not just as a researcher, but also as an artist; because there's that part of my life too as I am trying to make work in an artistic context. But then the researcher side of me is like, well, why do I want to be using this training data? Why do I want to make stuff?
If the researcher side of me is saying, well, you're kind of recombining stuff from the data set and the data set's problematic. Well, one of the things that I'm trying to do then is think through, well, how can I use the system without referencing the data? What might that look like? And so I'm glitching them. I'm trying to think about what are the things that these systems can't do and how do I ask them to do that anyway. And what do I get when I ask them to do it?
One of those things is for example, asking you to generate noise, as I kind of gave in my rough description or presented in my rough description. It's kind of generating random pixels, and something is checking to see if I've asked for a tree, it's looking at these random pixels and seeing if there's anything tree like in them.
But if I asked for noise, the pixels themselves, that random generation of pixels is noise. And so it doesn't actually pass that test. It breaks. It's almost eliding part of the structure of the generation process. As a result, it doesn't really know what to do, and it ends up trapped in this kind of feedback loop of asking the recognition program, the recognition tool, whether or not this is noise. And it's always going to be told that it is noise. But then what? Then it takes it back and refines it. It tries to find patterns to clarify. And it can't, because it's noise. And so it's generating these abstractions.
And so I've been playing with this idea of abstraction and what gets through. If you ask for certain things, sometimes those things come through. Really basic shapes will still be recognized. But you get these noisy, swirly, very colorful abstract patterns that are an artifact of the process breaking down. As opposed to an artifact of the training data being referenced in order to produce something.
To me, this is what I enjoy about this process, or what I feel is satisfying about this as an artist, is that it is a way of using the tool that isn't prescribed by the tool makers who have defined a kind of view of creativity as just the object. You want to make a photograph that looks beautiful, something that references photography or references painting, references cinema. And now we're doing something that kind of doesn't. And I don't think that's the only way.
I really want to be careful to say there's a lot of ways to make art, and creative intent expresses itself in many ways. But for me, I found this really satisfying to explore what my relationship is and how to break out of that relationship. Because I'm so interested in the constraints on creativity and the constraints by definitions imposed on creativity by these tools, that being able to find ways that escape those constraints is really satisfying. So that's just one example of the types of things that I'm trying to explore in my work.
KIMBERLY NEVALA: And so is there one, whether it's a question or a point to ponder or a lesson, that you would want to leave with those of us like myself? Unfortunately, I'm highly uncreative which is why I think I appreciate those who really are. But who are using these tools either as part of artistic endeavors or even in more mundane corporate contexts that we should be thinking about asking or understanding as we move forward in this complicated, complex world?
ERYK SALVAGGIO: Yeah. There's a couple of questions I think that are really important.
One of them is ‘what are we actually referencing in these things’? When we are relying on an algorithm to answer a question about the world, how much of the answer is the algorithm and how much of it is the world? I think that's a really important critical question to be asking in all kinds of ways. Whether we're building a system, using a system, or critiquing some thing that we've learned from one of these systems.
The other question I think that's really kind of related to that is, ‘what are we doing that we are not doing when we use A’I? And one of these things with generative AI in particular is the process of thinking. When we look at a blank page, are we really just desiring to fill it? Or are we really being invited to think about the thing that needs to be on that page. And I'm not saying that you can't do that with generative AI. But I am saying that it really seduces us into not brainstorming but relying on a kind of list of ideas that are generated by the tool.
When we hand over that power, we are really surrendering a lot about our own contributions to the world around us. And that's a disservice to us. Whether we think we're creative or not, the ideas in our heads, the expressions of our life experience and our expertise, even our lack of expertise, which is also something I think is important. If we don't know how to draw, that's OK. Sometimes you draw anyway just to express an idea in a rough shape and stick figures.
So what do we surrender when we say, well, we're going to let the AI generate this visualization of what our office space is going to look like? Or we're going to use ChatGPT to generate a kind of outline of a paper that we want to write or a report on this fieldwork we did or an investigation or a proposal. Where do we get lost? What is getting taken away from us when we rely on the algorithms to sort a blank page on our behalf?
And where might we find some confidence and courage to express that agency even if it is faulty, even if it is flawed? Where can we engage other people to strengthen that, as opposed to relying on fundamentally an abstraction of the average? I think that that's a really important question for all of us to be asking.
KIMBERLY NEVALA: Yeah. And I think with no further ado, all I should do here is thank you for your insights and for provoking thought in these areas. It's really critical to how we move forward as individuals and as a society. So thank you again.
ERYK SALVAGGIO: Thank you.
KIMBERLY NEVALA: I really appreciate the time and the thoughtful framing, if you will, of the frames of AI that we're dealing with today.
ERYK SALVAGGIO: It's been a real pleasure. Thanks so much.
KIMBERLY NEVALA: Excellent. Well, to continue learning from thinkers, advocates, and doers such as Eryk, please subscribe to Pondering AI now. You'll find us on all your favorite podcatchers, as well as on YouTube.
Creators and Guests
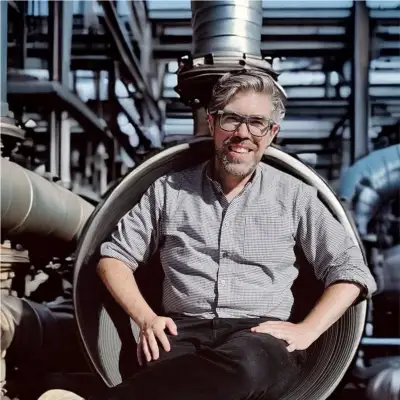
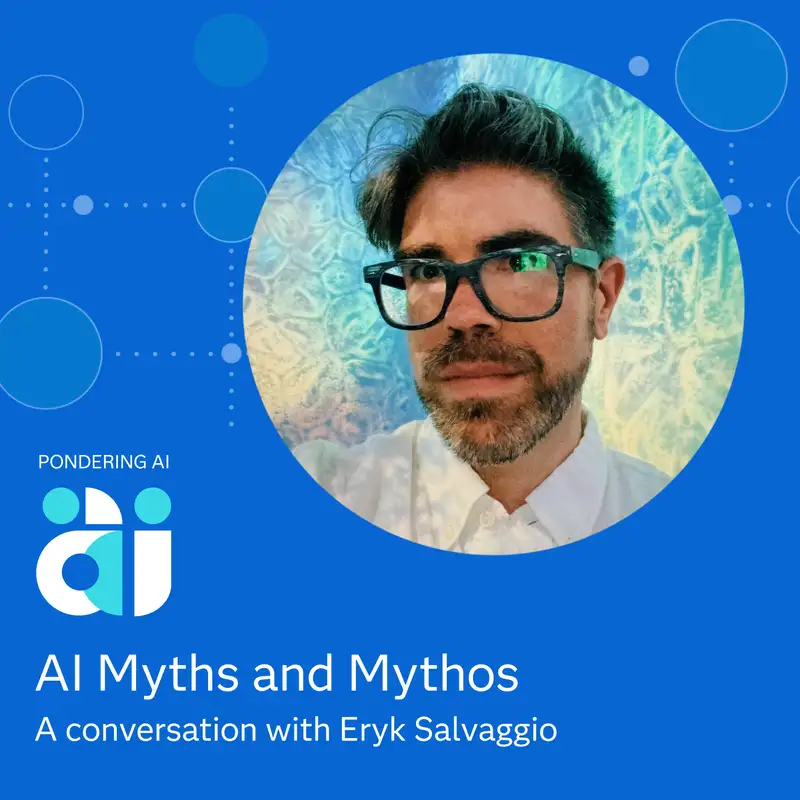