Critical Planning with Ron Schmelzer and Kathleen Walch
KIMBERLY NEVALA: Welcome to Pondering AI. I'm your host, Kimberly Nevala. In this episode, I am so pleased to bring you Kathleen Walch and Ron Schmelzer. Kathleen and Ron are the dynamic duo behind Cognilytica, which is now a part of PMI - also known as a Project Management Institute. Today we're going to be talking about the nature of AI, what is required to manage AI projects successfully and perhaps, engage in some critical discourse about the nature of critical thinking.
So Ron and Kathleen, welcome to the show.
KATHLEEN WALCH: Thanks for having us. We're so excited to be here.
RON SCHMELZER: Yeah, thrilled to be here.
KIMBERLY NEVALA: Awesome. Now, we talk a lot about multidisciplinary teams and the importance of that in AI and beyond. And in looking at your profiles, it strikes me that Cognilytica is very much a case study for this. So can you tell us a little bit about, introduce yourself, and give us a brief synopsis of your background and how you came to join forces for Cognilytica?
KATHLEEN WALCH: Sure. So I'll get started. I'm Kathleen Walch.
I was one of the co-founders - am one of the co-founders - of Cognilytica, which has now been acquired by PMI, Project Management Institute. So we're really excited about that. But Cognilytica started in 2017 and actually, Ron and I had been working together prior to that as well at Tech Breakfast, which was a morning, demo-style event. Where we would have different companies, a lot of startups, but different companies of all sizes come and demo and showcase what they were working on.
Around 2016, 2017, we really started to see a lot of demos around AI, and we said, there's something here. This is really hot. And so we started a boutique analyst firm covering the markets, covering the AI markets, and then quickly realized that folks did not have a methodology for running and managing AI projects. So we developed the CPMI methodology, the Cognitive Project Management for AI methodology. But I will let Ron talk a little bit more about his background and then the methodology as well and how we came to be at PMI.
RON SCHMELZER: So thrilled to be on your podcast. And as you know, we're long-time podcasters. We have our AI Today podcast. Some of you might see it here in the background. Here, we have our little background presentation. One of the top three podcasts in AI, the series with well over 100,000 monthly downloads. And we've been doing 470 episodes, oh, my goodness, for so many years.
And you know what, we never run out of things to say about AI, and it's always focused on what people are doing with AI today, hence the name AI Today podcast. And that's how we had evolved this idea of running and managing AI projects. We were doing this podcast while we were working with clients who are working to put AI into production.
Nowadays, pretty much anybody can pick up a tool and do something with AI. That was generative AI, the big revolution. But when we were talking in 2017, 2018, it still took teams of data scientists and machine learning engineers and all those sorts of folks to really make AI projects work. And when you spend all that time and effort and the AI project fails, as you know, 80% of AI projects fail, you don't want to be taking that risk.
So we had to resurrect an old methodology that was more focused on data mining called CRISP-DM and say, well, it was great for data projects. And AI is a kind of data project, but we need more guidance on running AI projects. So we developed the CPMAI, cognitive project manager AI methodology in 2018 along with some government institutions and some financial and other industry folks who are putting into place. And since then, it's been fantastic.
KIMBERLY NEVALA: This is awesome. I am embarrassed to admit that I've been aware of CPMAI for a long time but when I have talked to folks about it I have said, oh, this is certified project management for AI. And just the other day I was like, you've been saying that wrong for a really long time. Because it's cognitive project management and I think that's important. So I want to come back to that point in a minute.
But we use the term AI. It's the royal AI, and it means a lot of different things to different people. So just as a grounding, because we are going to be talking about what is new novel and maybe not so new relative to project management of AI projects; when you guys say AI, what is included under that umbrella?
KATHLEEN WALCH: Yeah, that's a great question. So actually, back in 2017, when we first started Cognilytica, a lot of people would get tripped up with what is AI, what is not AI. And we said, look, because there is no commonly accepted definition of AI despite the fact that the term has been around since 1956.
So around 2018, 2019, we actually said, why don't we just break it down one level deeper and say rather than talking about is this AI, is this not AI, what are we trying to do with it? So we came up with the seven patterns of AI as a way to say, what is it that we're trying to do. And then this helps you understand is this a project that's suitable for AI? Is AI going to be the right solution, or is it not?
So at a high level, the seven patterns and they're in no particular order, because we present it as a wheel.
One is hyper personalization. So this is treating each individual as an individual. So we can think about this with hyper personalized content, which is a marketer's dream, but also, personalized education or health care or finance.
Then we have our conversational pattern of AI. So this is machines and humans talking to each other. We think about AI-enabled chat bots in this pattern, also large language models.
Then we have our patterns, pattern of AI. So this is being able to make sense, look at a lot of different data and be able to spot patterns in that data and outliers in that data, as we think of fraud detection.
We have our recognition pattern. So this is making sense of unstructured data, which we think about how much data do we have at an organization. 80 plus percent of that is unstructured. So think about emails, audio files, like a podcast recording. None of that is going to be queryable.
Then we have our autonomous pattern. So it's important to understand automation and autonomous are not the same. So the goal of the autonomous pattern is to pretty much replace the human. So we think about autonomous vehicles, for example.
Then we have our goal-driven systems pattern. And so this is really around reinforcement learning and finding that optimal solution to the maze.
And then we have predictive analytics, so that's not replacing the human, but helping them do their job better, looking at past or current data.
KIMBERLY NEVALA: Yeah, I really like this because a, it doesn't require us to at all levels be able to speak to the deep technology. But it also forces a bit more of a conversation to start with. As we always go back to what problem are we trying to solve.
Now, I'm going to break my own rule here right off the get and use the term AI broadly. And then allow you to bring some examples that are most relevant with the pattern that makes sense. But as we well know people's perceptions of AI in any of these flavors vary. And their perceptions of what is required to bring these projects to fruition can very often differ from what really happens on the ground.
So in your experience, what are the biggest hurdles or roadblocks people perceive when it comes to AI? And then we'll turn the corner on what they're really going to run into.
RON SCHMELZER: Well, there's a few things that we would say like the actual roadblocks and then the perceived roadblocks, as you mentioned.
Especially now that I would say generative AI has made AI available to the masses, and in many cases, even with or without you intending to use it, now, there's that little magic sparkle that you get in some document, and you're using AI to generate new text or new images or to do some analysis or to help you fix something.
That's going to be the predominant mode. I think that most people are going to be interacting with AI just in the context of what they're doing every day. Those people won't see roadblocks, per se. They'll just see capabilities.
But the people who are building those tools, trying to make them work, as we say, there's the common set of reasons why AI projects fail. A lot of times it's because of data: data availability, data quality, but also, about trying to solve the right problem.
Sometimes people throw AI at a problem when they don't need to throw AI at a problem, or it's not necessarily the best solution. And then of course, there are issues around product market fit and all the standard technology issues.
So those, I would say the real practical roadblocks, but we also talk about fears and concerns which are: people don't want to use AI because of, well, we can talk about this. Either they're afraid or concerned or the business doesn't allow them to use it for many reasons.
KATHLEEN WALCH: Exactly. And so we always talk about the fears and concerns related to AI because fears are more emotional based. But it's how they feel. So you can't diminish that because you can't tell somebody, oh, just go use AI. And they're like, well, I'm fearful of it. And you're like, who cares. We're not addressing that. So those are issues that we need to address.
And also, there's real concerns around AI as well. And so these are more maybe rooted, in fact, where people are concerned around privacy, data privacy. Or that they are concerned around data usage. And many companies are using this now, and so how is that going to impact their life going forward.
So we have to make sure that we address those fears and concerns. Both are important, and we don't want to diminish it because when we do that, then we find that people just get even more resistant to it.
And then we also have issues, like Ron said, where sometimes organizations are not being proactive about this. So they're saying, we're just going to have a blanket ban. And we said, OK, but now you're going to have people that are using it under the radar. So this is going to continue to be a technology that people are embracing. And so we can't just ignore it and sweep it under the rug and say, we're just going to have a blanket ban because people are still going to use it.
KIMBERLY NEVALA: And how do you approach and talk about both fears and concerns at different levels of the organization? Because I have to imagine which fears and concerns bubble to the top, and the gaps that they perceive are very different - although maybe we would hope they wouldn't be - but they're very different when we're talking to senior leadership, the C-suite, down to folks on the ground.
RON SCHMELZER: Sure. I mean, some of those concerns are realistic, especially when it comes to data. Where is my data being kept? Are you using my data for training purposes? Are you going to protect my data, keep it confidential? That's, I would say, a very valid concern, and people have reasons to be concerned because organizations haven't always been so responsible with data. Those can bubble their way all the way to the top.
But then the problem is when they say, well, because of these concerns, we are going to say, as Kathleen mentioned, no use of ChatGPT anywhere in the organization. That's impractical because while you might be able to say block the usage of people going to the website to enter it if the tool is embedded in your PowerPoint or it's embedded in your Excel, you can't turn off Excel or PowerPoint just because there's a little bit of AI into it. So practically, that's actually not a practical thing.
Some of the other concerns about job loss are that's where you start to see the separation. Because maybe the C levels like that part of AI. Where they can automate people's jobs and use AI in ways and maybe the lower levels don't like that. So even there, it gets weird because people want to automate their own work. So they don't like it when say, the employer says, oh, this whole job category, we're going to replace this with tools. That's a threat. But people don't mind it when they say, well, I have eight hours of work to do, but I can recover two hours of it if I just automate it on my own. That, they're happy to do. So I think this is kind of where we start to see the split between the individual value proposition and the organizational one.
KATHLEEN WALCH: Absolutely. And then to add to that, too, I think that from an organizational standpoint, we say this needs to be a top-down approach because people at the bottom will figure out, OK, how individually can I use this. But people at the top need to really be setting that tone and saying, are we going to look to replace certain jobs or roles, and if not, then we need to be sharing that.
So we talk a lot about this idea of augmented intelligence, where you're not replacing the human but helping the human do their job better. We call them give them AI superpowers, so that they're able to be more efficient, so that they're able to do things that maybe they don't necessarily need to do. That's kind of not really within their job scope, but something that needs to get done, like meeting minutes, for example. Why can't we use an AI tool to help create meeting minutes. That'll make it so much more efficient and save x amount of minutes or hours per week.
So that really needs to be that top-down approach as well to say, hey, let's embrace AI technologies. Let's help us do our job better, but don't worry about being replaced buy these tools, because it's just helping you do your job better.
KIMBERLY NEVALA: So there's something interesting in what you just said, Kathleen, in juxtaposition with what Ron said. Which is that sometimes the fears and concerns and even the motivations from those at the top are very different from folks doing the day-to-day jobs at lower levels of the organization.
So I have to assume that when we say there needs to be a mandate from the top, we're not just saying that that's enough. But that folks in the C-suite or executive higher level decision makers should be signaling acceptance and promoting the use of AIand that we should be working together with folks doing the job, doing the work so that they can determine how to best apply artificial intelligence. Is that a fair approach or perspective?
KATHLEEN WALCH: Yeah, it is. Another thing that we've talked about too - and this was related to RPA robotic process automation a number of years ago, but it is relevant for AI - especially when we're thinking about tools and technologies that we're going to be bringing in.
Whenever we're looking to do that, we always want to have stakeholders involved and say, what are your real pain points and then let's work to address that. So if your real pain points are meeting minutes, for example, and it's taking you three hours a day to summarize all of these meeting minutes, then let's bring in a tool to help, and let's immediately see that impact. Because whenever we talk about AI, we always want to be talking about that return on investment.
So what is that return going to be? Because there's money, time, and resources involved to either build these AI solutions or even just to adopt these solutions. Not many things are free. So what is that going to look like? And say, what are our immediate pain points, and how can we fix them. So how can we help us do our job better?
That's how individuals need to be approaching this, and that's how organizations need to be approaching it too. When we're making bigger impacts to the organization, that's where you need to see that top-down approach. So we say, hey, we're going to be embracing this. We're not looking to replace you. We're not looking to reduce headcount by 10%, because we're bringing in AI solutions.
So that needs to be something that senior leadership continues to iterate with the team so that they feel secure using these technologies. But then from that individual standpoint and working your way up, say, what is it that I need to immediately solve to help me do my job better. And then what can be some of those medium and then longer-term plays and start bringing in those technologies.
KIMBERLY NEVALA: I think people would be, in some circumstances, well within their rights to be skeptical if management is coming at them with some of these things and saying, but it's OK. Your job will be fine.
It is somewhat of a… you will, if you're at the top level, need to prove it to some extent. Because certainly what we see broadly, at least the PR around some of the commercial incentives, doesn't align with that. So in my mind, that's actually a lot of the hurdle that we get within organizations today. And so there's a lot of communication and change management. And then I think just showing up and proving that you're holding that promise with your employees.
Now, that being said, I want to go back to the core concepts around CPMAI, cognitive project management, which makes so much more sense. But anyway, what are you going to do? What were the specific aspects when it comes to managing an AI project that required this extension to good, old-fashioned project management methodologies?
RON SCHMELZER: The thing is that, it's interesting. If you think about the things that's required to put any sort of technology project into work, you always have three things. You have the technology itself, so AI, but it could be the web or mobile or some whatever else. You have the people, the people who need to implement stuff, the developers, the designers, whatever it is. And then you have the process, which is the method you go about doing it. And the thing about it is for websites, the process is pretty straightforward. You're building a website. You do the design. You put it online. It's pretty straightforward.
But for AI, it gets a lot more complicated, because the data determines how the system works. So you can have great people who are great data scientists, whatever. You can have great technology, the latest models, the latest technology but if you use the wrong process, you will get a bad result. And it happens even to the best people.
Andrew Ng is well known for talking about how they put together a radiology application for landing AI. They basically built an AI system to analyze radiology images and it was working great at Stanford Hospital. And the moment they tried to use it somewhere else, it was failing terribly. And Andrew was like, why is it that it was working so well in this one place, with this good quality data, with good quality machines. Then we went down the street to an older hospital with an older machine that people were using slightly different protocols and it was failing. And the answer is, it's because things are different. The data was different.
So you could have said, well, maybe you should have started with the bad quality data. Maybe you should have started with the bad quality machines. So that wasn't a change in people or technology. That was a change in process. So really, the origins of CPMI were this idea that if we can do things in a rational process here, where we consider what problem we're trying to solve, specifically what data needs to solve that problem, how we prepare that data to solve that problem, then what technology approach do we use based on the data that we have, then how do we test it to make sure it works. And then how do we deploy it in a real-world environment to test it.
By the way, those are the six phases of CPMI I just mentioned. If we can do that, in short, then we have a higher chance of success. Because a lot of times now, people are really just throwing stuff at the wall and hoping and praying that stuff works. And we see it first hand in a lot of organizations.
KIMBERLY NEVALA: And especially today. I think in some of the ways that we are engaging or interfacing -- I think I tend to focus more these days on the power of LLM as an interface. But engaging with these systems, in the radiology example is a good one too, where we have to rethink the process a bit as well, and people need to be really trained.
So this idea of change management isn't new, but the idea of how much or how little we should rely on the outputs; I don't ever question what I get out of calculator if that's actually the right answer. But we really do have to do that with almost all forms of artificial intelligence. Some are more prone to error than others. However, there is always that aspect of how do you balance your own judgment with that.
And again, we've talked about this in analytics for a really long time. It's one of the reasons I hate the term data driven because it's hard for people to understand what that is and it tends to assume a preference just for whatever a model or whatever the KPI spits out. And that's really not what we're talking about when we talk about data-driven decision making.
But I am wondering in what you see is that understanding of how people interpret, understand, and then actually apply the outputs of these models - whether it's a recommendation or it's a number or it's a metric - does it require some extra care? Is that interpersonal change management perspective also heightened here?
KATHLEEN WALCH: Yeah. I mean, AI is probabilistic, not deterministic. So you're never going to get 100% accuracy. So you need to really understand. That's why we say you have to have this step-by-step approach for running and managing your project. And so understand the data that goes into it, understand what problem you're trying to solve, and then understand the outputs as well.
And it comes to anything. It comes to using AI technology or building AI technology. So large language models, I think, everybody is playing with at this point in some shape, or form; from personal or professional or both. And we always say you have to apply critical thinking skills. You can't just take the response that is given, especially the first response, and take it at face value. Apply some critical thinking there.
Same thing happens even if you build an AI system. You need to continuously be iterating it and there is something called model drift and data drift. Over time, your model will decay. Over time, the data that goes into this system may change. So you constantly need to be retraining this model. You need to be managing and monitoring that output.
We always say AI is never a set it and forget it, and we say that a lot because it's really important to continue to stress that. It is not a set it and forget it.
We always like to have ripped from the headline examples where Ron brought up an example before. Because if other organizations are getting this wrong, you will too, if you don't think about this. So Air Canada, a few months ago, maybe almost a year ago at this point, said, hey, we need a large language model to be put on our website so it can be a chatbot that can answer any question to anybody, any time of the day or night. Sounds great, in theory. But in practice, the chatbot was actually getting answers incorrect. And same thing happened with New York City. They had a chat bot that was actually giving false information and illegal information about tenant laws.
So you can't just set it and forget it. You have to make sure that it's giving the answers that you need. In the case of Air Canada, they were sued. They were brought to small claims court, sued, and lost. Same thing with New York City. They were getting a lot of bad press for this.
So you need to make sure that you're checking the answers that come out of your AI systems, whether that's a chat bot, a large language model, or anything else that you're building and constantly be making sure that it's staying up to date, use it as a tool, and really understand. That's why we say the seven patterns of AI is so important too. So make sure you understand what problem you're trying to solve. Make sure that AI is the right solution, and then understand that it's probabilistic, not deterministic.
KIMBERLY NEVALA: And certainly, folks will be familiar with the idea of model drift, with data drift. I wonder how much sort of people drift is something that we should be adding to this as well. We used to talk about this - again, way in the way back - with predictive systems.
Which is if we are successful in using these systems to influence people's behavior, their behavior will have changed. And therefore, the basis of your model will have changed.
And so even if your model is still running and doing what it was aligned to do originally, it may no longer be in alignment with that. So there is that external factor as well, which comes into play. And I'm wondering if you see organizations addressing that explicitly, or is that just part of doing business in their minds?
RON SCHMELZER: It's really interesting insight.
Sometimes people think of people drift as being represented in the model. People's behavior is changing. That's why the model's behaving differently. But nobody has thought about that explicitly, thinking, well, it's not just because people are randomly behaving differently. Maybe they're actually changing their behavior. That's a big insight.
Hey, ask Google about that because that is changing their business model. People are starting to change their behaviors because they're stopping to search and they're starting to ask questions on LLMs. And that's hurting their fundamental business model, which is advertising. You're not getting as many search queries, and that's a whole other question.
But I think you're right. I think organizations, as people start to get more day-to-day experience with AI, they will be changing their behavior. On the one hand, that's something you have to respond to. But on the other hand, that's actually a challenge for us as individuals.
Sometimes I'm doing something and I'm like, wait a second. I think I could be doing this better if I just used a model, use an AI system. Maybe I'm like, why am I manually entering things into spreadsheets? Maybe I should just ask the model to do it. It's a rewiring of our own behaviors, and I think it takes time for people to learn new behaviors.
Of course, everything is changing simultaneously. So you learned something today. That doesn't mean like six months from now, there might be a completely new model that can do something completely amazing that you couldn't have done six months ago. That might change your behavior even more.
So I think we're at this really weird, hyper accelerated timeline where everything feels like it's moving faster, because I think it is. But also, people are responding faster to it. And then that's motivating other people to respond faster to it. And it's kind of like, what is it, the Red Queen theory where if you're not running, you're falling behind. And it just takes all the running you can do just to stay in place. And it feels that way.
So if you, as an individual, are not staying up to date with the AI tools and capabilities, you might find yourself like Rip Van Winkle waking up and the world has changed around. I don't have anything more to add to that, but that's kind of a cool insight.
KATHLEEN WALCH: Yeah, it is. We talk about this idea of the AI first mindset too. Where, I think, even just a few years ago, maybe even a few months ago, it was really people's behavior if they needed to search something to go to a Google or any web browser and do a search. And now, we're starting to see people going to large language models to do searches and having that as their default homepage, rather than what they used to have.
And so it takes time for these behavior changes, but we're starting to see it. And so again, we always say never start with a blank page. How do you, at least use AI as a first draft, whether that's for slides or whether that's for an image that you want to create or whether that's for a document or text that you need to work on. Always have that augmented intelligence help you do your job better. So never start and write it from scratch. Always have AI be that tool to help you.
KIMBERLY NEVALA: Now, particularly with LLMs, there is certainly a lot of, I think, justified concerns about things like their environmental impacts. And we've gotten a lot of hyperbolic statements about we're never going to catch up with climate change, so we might as well run as hard as we can. And it doesn't matter if it uses a bunch of water because eventually, it'll solve itself. I'm not sure -- I actually still don't quite understand the logic behind this, I gotta be honest. As is evidenced by my weird ramble there.
But how are folks bringing in or are they, I guess is an even better question, this concept of ethical or responsible AI into the project?
RON SCHMELZER: It is like one of the most important set of questions you can be asking about AI.
It's hard to talk about AI without talking about the ethical and responsible and trustworthy, transparent, and governed, and explainable, and the impacts of AI on the environment. They're all so tightly connected. A while ago, we built what's called a trustworthy AI framework. Because just like when we were talking about with AI - and it's a combination of a whole bunch of things, but if we just talk about it as one unique entity - it's kind of hard to really talk about it when you're trying to do something.
Same thing with ethical and responsible AI. There's a lot of interconnected ideas, but to talk about it all at the same time at the same level is hard because you want to do something about it. So we isolate it to there's straight-up ethical concerns. Do no harm. Machines that are acting autonomously without our control, the superintelligence, these are things that are not really beneficial for humanity in any sort of way, shape, or form, except for the people who might want it to be like that. You're right. There's some psychopathy involved in that.
But then there's also responsible use of AI, like, for example, facial recognition. Is it good? Is it bad? It's neutral. There are good ways to use facial recognition, and there are bad ways to use facial recognition. So how do you decide and determine which ones are good and bad?
Then we have this layer called transparent AI. And people might think about that as the algorithmic stuff. That's actually explainable AI. That's a whole other thing. It's more like giving people visibility into how their data is going to be used, whether people even have the ability to consent to the use of AI or basically, opt out of the use of AI. Or even the ability to dispute or let's say an AI system was used in a hiring position and it made a recommendation that's bad. Do you even have the ability to dispute or doing that sort of stuff? Is there a chain of a human accountability? This is not even human in the loop. Is a human even accountable for the decisions that the systems make?
And then we even have governed AI. Are there rules in place? So all of these things, when you divide it out, then you could say these folks should be concerned about this part. These folks should be concerned about that part.
The power is really interesting because in the recent news, there was an article about how Google is funding this nuclear power company to build seven nuclear reactors to power their AI and power and data centers, which is interesting. You might have heard that Microsoft has actually funded the restart of the Three Mile Island nuclear reactor, which was on hold. It's like, whoa. And so clearly, I mean, people somehow right now, seem to think that AI has no environmental cost, but it does. And you have to face the music at some point with that.
KATHLEEN WALCH: Exactly. And specifically to that, what we've seen is that when it doesn't impact you right on a daily basis, you're just on your computer and you're using it, you don't see that environmental impact. So that's also why it's really important to have these conversations around trustworthy, ethical, and responsible use of AI, and that it continues to get brought up.
Because otherwise, it's just going to get buried. Because the folks that are building these, obviously, don't want to be pushing that narrative too much. And I think that sometimes that can get buried when people are just trying to figure out how they can use this technology to better do their job or to help improve different processes at their organization.
So this is why it is really important to continue to bring that up, because it's not something that you see necessarily on a day-to-day basis.
KIMBERLY NEVALA: So I want to take a slight turn here, although I think it's related to everything we've just talked about, which is this idea of critical thinking.
Somewhat like the term data driven back in the day, which, like I said, was my least favorite term. Not because I didn't think it was valid, but because it didn't have a whole lot of definitive weight behind it, so it was really unclear for folks.
And so we hear a lot now about why critical thinking is so important in the age of AI when we're both identifying, deploying, developing, consuming AI systems. No one is going to disagree this is important, that this a soft skill. I do think, though, there's a difficulty in connecting the idea with very discrete actions and behaviors.
So first, let's talk about why critical thinking is especially important in the context of AI projects. I, somewhat tongue in cheek, but not really, said to you before that one would have hoped, especially in the analytics realm, that this was always standard practice. And maybe it was, to an extent. But why is this especially important in the context of AI?
RON SCHMELZER: Well, as they say, common sense is neither usually common or sensical for some people.
So critical thinking. Actually, it's interesting. So Kathleen and I also write for Forbes. We have a column we talk about it, and we dug quite a bit into soft skills. We also did it on our own podcasts as well.
And there's a couple of ways of answering this. Kathleen and I have a lot to say on this subject.
The first is that when you think about it, you have to be critical about what you put into the machine, because as smart as we think they are, machines are still garbage in, as garbage out. They're data - well, I'm not going to use that word - I'm not going to say data driven. They're not data driven.
KIMBERLY NEVALA: You can, as long as you define it.
RON SCHMELZER: They’re data forward, which is what we liked to talk about. Which is like data is one of the more important things, amongst many other things. So if you are just say a naive user and you just put in some -- let's say it's a prompt, right. And you ask for something and it comes out generic, you're like, well, it's not the machine's fault that it did that. It's you, you are the human providing the input right into it. And so don't be surprised by the inputs that come out.
So part of this is the critical thinking is like, well, that's a human skill because machines aren't good at it. And machines probably will not be good at critical thinking and common sense machine reasoning for a while. That's actually an area of technology we have not solved.
We tell people, we look at this thing called the DIKUW pyramid. I don't know if you're familiar with that or your listeners are. It's the idea that as you go up the stack of starting from the base of data, the D. As we tried to get more value from data, we have to do harder and harder things.
So the first layer above D is I, which is information, where we basically say data stored in a database by itself isn't valuable. You need to look for correlations and connect things together. That's like reporting and analysis and things like that. OK, great.
But what are the patterns in that data? Well, you gotta go up one more level. That's the K level, the knowledge level. This is actually where machine learning is trying to identify the patterns in those relationships. And then apply those patterns to new data.
Then the next layer is the U layer, which is interesting because it's missing in many traditional diagrams. They called it the DIKW pyramid, which is once you go from knowledge, you go to wisdom. It's like, well, you can't get to wisdom without understanding.
And this is the problem we see with machines; that they can sense the patterns, but they don't actually understand the patterns. So if you go into an LLM, although supposedly ChatGPT o1 has fixed this problem, and that's why they called it Project Strawberry, which for our listeners, don't understand why it was called Project Strawberry for a reason. But if you ask an LLM to do math, it usually will get it wrong.
If you go what's 3 plus 5 plus 27 times -- and you do all that, and you're like look how stupid the LLM is. It can't get the math right.
It's like, well, you're probably asking the wrong question, because it's really just trying to predict tokens. It's not doing math. It doesn't understand math. It just uses its internet trained quantity of data to figure out what it thinks the next token should be, the next word should be. So it doesn't even think of 3 and 5 and 7 as numbers. It thinks of them as words. And it's like looking up the answer to a math problem in a really big dictionary. It's probably not going to be there. And this is because the machines don't understand it.
Now, the reason why ChatGPT's 01 was called Project Strawberry is because they say, how many R's are there in strawberry. It's kind of funny. You ask your kids that, sometimes they get it wrong. There's three R's in strawberry. But if you ask ChatGPT 4.0, if you ask it now, it'll say there's two. It just doesn't get it right. It doesn't understand that you're asking it to count the number of letters. o1 adds reasoning, right now into this chain of thought, which that's the clue that we need that extra level.
So this is a long way of answering your question, that machines are not good at reasoning, they're not good at critical thinking. And that if the human is not doing it, you're giving to the machine the thing that machines are really not very good at. But we could talk more about critical thinking on that topic.
KATHLEEN WALCH: Yeah. So we also need to be thinking about the responses that we're getting as well. So Ron brought up some examples, and you look at it and you go well, that's silly. It can't count the number of R's.
But let's say that we're trying to have it help us do something for work. Well, we're not going to take the first response that we give it and say that we need an outline for a document and say, OK, whatever outline it gives, we're just going to take it, copy, paste it, and use it. We've seen that, and sometimes it says, insert company name here or insert date here. And people will literally copy it, and paste it into a document, and then send it out. They're not going to read it. They're not going to check it for errors. They're not going to add in their own information.
We always say, dig one level deeper. Say, what's the first response? Let's work to iterate this, to get the response that we need. And that's where critical thinking skills need to be applied as well. So you have to say, how, as a human, am I going to dig one level deeper? How as a human, am I going to work with the machine to make sure that I'm getting the responses that I want.
And then at the end of the day, the human needs to own this. Because we can't just take a response that comes out of a large language model and say, OK, let's just copy it, paste it, never read it, never proofread it, never edit it, and just move forward. And it's tempting to do that, because we look at this and we go, wow, they get such great results, and wow, this looks so good. But if we're not actually really applying those critical thinking skills, then we're doing a disservice. And so that's how we put things out that have insert whatever here.
KIMBERLY NEVALA: So I'm going to do my typical bad host move now and ask two questions at once and let you guys pick from that. One is, is critical thinking something that can and should be taught, and if so, how do we approach that? And then secondarily, is there a relationship between our ability to think critically or critically critique the systems that we're being asked to engage with in basic AI literacy?
RON SCHMELZER: Well, we'll split this one up and go for it.
KATHLEEN WALCH: So yes, critical thinking can be taught.
And we always talk about this idea of a lifelong learner, and this idea of a growth mindset. And so just because you're not in K through 12 or higher education doesn't mean that you can't and shouldn't still learn. Critical thinking can and should be taught at all levels of education, but especially, in your professional development and your career.
We have seen a lot of different courses out there. Ron and I also talk about this on our podcast. We write about this, and people need to feel empowered that they should go out and learn this on their own. But it is something that absolutely can be taught and still needs to be like a reflex. Say, OK, just because I got this answer, is this right? Should I dig a level deeper? And that's where you need to apply critical thinking.
And it's not just when it comes to AI systems, it really should be throughout your professional career, even with colleagues and things like that. But it is absolutely something that can be taught.
RON SCHMELZER: And what was the second part again? I think I might have missed that. Yeah.
KIMBERLY NEVALA: So my question was, is there a critical link or a relationship between basic AI literacy and the ability to create appropriate critiques?
RON SCHMELZER: Well, there shouldn't be. There should be a link.
People shouldn't be learning critical thinking while they are doing AI, but unfortunately, I think it's not taught that way. I think there's too much of an emphasis on tooling and capabilities and functionality and look at the cool thing it can do and not enough about, hey, wait a second, you should probably be thinking about what you're putting into it. You should be thinking about how you're using these systems. You should be thinking about these outputs and what this really means.
I think the interesting thing is the tip of the spear of people thinking about critical thinking as it applies to AI is the educational institutions. Because I don't think they have much choice, up until very recently. And of course, there's always Wikipedia and computers and cutting and pasting and all that sort of stuff, and people learned, OK, well, if I'm going to give an essay online, I should probably make sure they're not copying stuff from other places. But now, GPT systems and the AI large language models, in general, really make that very difficult, especially when they're built right into that tool. When you open up Google Docs, it says, what do you want to do? It's so tempting as a 10-year-old or a 20-year-old in college or whatever, to let the system do the work for you.
So the educational institutions are rethinking what it really means to be critical and understanding. And now, they're saying, OK, fine, go ahead. Create that. Now, let's go ahead and think about the outputs. Let's think about what's happening here. Let's apply that. And do you spot the errors in your own work? Do you spot the biases in the data that might have created these outputs? Now that you know this, how would you actually apply this?
These are things that machines aren't good at, common sense and reasoning, so these are things that you can do. So I think we're starting to see some inputs there. It's interesting.
There's also -- because I have a son who's in high school now. He's actually going to be going through the AP exams and they had to change a lot of the AP exams because there's a part where you have to do practice and they're like, now the fact that GPT is here, we're going to have to change this. It's pushing them to do stuff in the old-school style, where you'd have to actually go talk to someone in person, like the old Socratic learning styles. Maybe that's all going to make a comeback. I mean, maybe education will look very differently a decade from now than how it does today. Maybe we'll be told, you know what, do your base learning with an AI system. Maybe it'll help you because maybe there's subjects that are really hard to understand, and if you can have a conversation with a smart system that can help it explain it to you in a way that resonates for you or allows you to dive deeper, rather than sitting, listening to the teacher who might do a one-size-fits-all approach for everybody in the room. Maybe AI assisted learning may improve education, but it will change, for sure, the way.
Now, I know it wasn't your question about critical thinking and AI and linking the two literacy together, but I do think it's linked in that sort of way.
KIMBERLY NEVALA: Yeah, and I think that's right. I think that's also us pulling back, right to that lens of critically assessing where and why and how. Not just for today but in the future. Kathleen, I might have cut you off there.
KATHLEEN WALCH: Yeah, that's OK. I was going to say we talk about critical thinking, but we also talk about other soft skills and they really need to come together. So critical thinking is one of them, but communication, that's also important and collaboration. And so we need to make sure that we are not just focusing on one soft skill, but really bringing them all together.
when we think about this, we say that it's two sides, right. So it's how do you apply these soft skills when you're using AI systems, and how do these AI systems help you with these soft skills? And it really is two sides of these coins. But you can't just focus on one in isolation. I like to look at many soft skills and bring them all in together.
KIMBERLY NEVALA: Awesome. Well, I could go on asking you guys questions for a very long time, and I'm going to stop myself. I say that on every episode, which is just true. But any final words of wisdom for the audience based on what we've been talking about today?
RON SCHMELZER: Yeah, I think the first part is like now, we're part of PMI. That's actually part of the news-making part is Cognilytica. We were independent for so long, writing this research and doing these podcasts and talking to so many companies and doing the training and certification on our own. And now, we're thrilled to have support of an organization that's been around for a while. They've been around since 1969. Project Management Institute, probably best known for their project management professional PMP certification. They have 1.5 million certified. They have a lot of people.
And I think the thing I'd like to end the note on is that there are people in some industries that feel like they may have the luxury of maybe sitting out the AI conversation or waiting for AI to happen to them or their industry. We've talked to so many PMI chapters, for example, where we've gone and we talked about the seven patterns or AI failures and things like that. And we get questions like, how is this going to impact me in the next 5 to 10 years? And we're like, 5 to 10 years? I think you'll be lucky if you have 5 to 10 months. And I think that's the final note.
Part of why it's good for us to be part of this bigger organization is because we have a bigger impact now. And I think that's what we're hoping is that to impact the world of folks who are trying to make stuff happen, be successful. And basically say, hey, look, we're here to help you. To give you the tools that you need, give you the support that you need, so that way, as the Red Queen not running as fast as you can just to stay in place, but to get ahead of all these changes.
KATHLEEN WALCH: Yeah, and I'd like to add that we always say when it comes to using large language models, there really is such a low risk for failure. Because just play around with it, right? Even if you're like, oh, I don't want this for work because I'm afraid just play around with it on your own. The risk for failure really is low. Get better at prompting, get better at understanding, applying these soft skills when it comes to using these LLMs.
And just keep up to date. Read on the topic, listen to podcasts like this or AI Today or other ones because the world really is moving so fast, and so you want to make sure that you're staying up to date. That you understand how, at least at a high level, what AI is, OK, the seven patterns of AI and then how to apply this to do your job better.
And for those project professionals that are looking to run and manage AI projects, make sure to look into CPMI so that you have a step-by-step approach to actually be successful with running and managing AI projects.
KIMBERLY NEVALA: Yeah, fantastic insights, and we will make sure to provide links to all of those resources and your ongoing corpus of work, which has been astounding, so everyone can continue to learn from you. Thank you so much for your time today. It's been great.
KATHLEEN WALCH: Thank you. We loved being on your show.
RON SCHMELZER: Yeah, Thank you for having us. Happy to spread the word of the Pondering AI podcast and get that out to our listeners as well.
KIMBERLY NEVALA: Likewise. Likewise. Thank you. All right. To continue learning from leading thinkers, advocates, and doers like Kathleen and Ron, subscribe to Pondering AI now. We're available on all of your favorite podcast channels and now, also on YouTube.
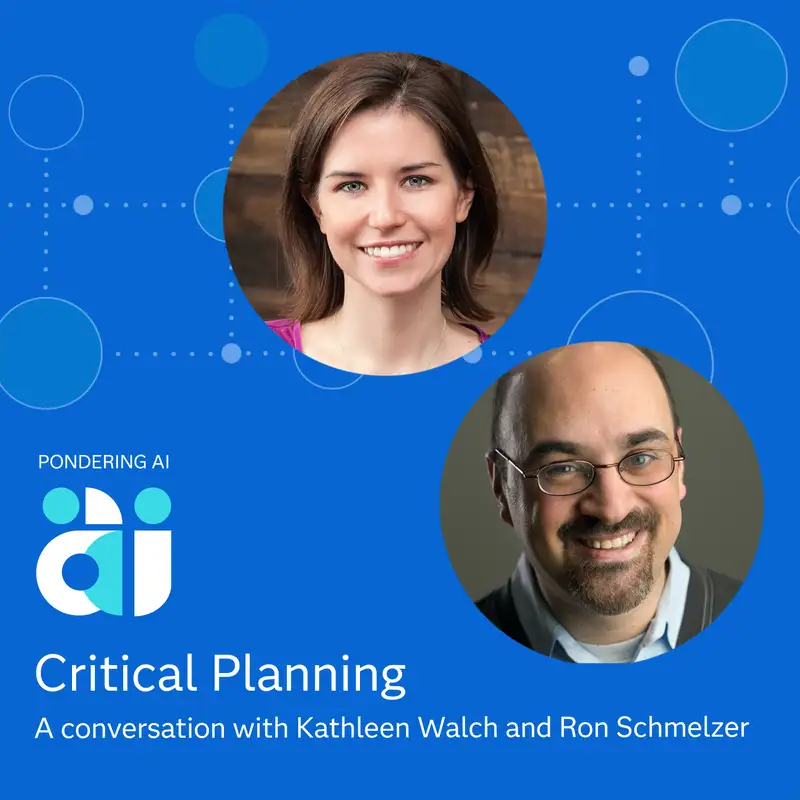