Beyond Bias in AI with Shalini Kantayya
KIMBERLY NEVALA: Welcome to Pondering AI. My name is Kimberly Nevala. I'm a Strategic Advisor at SAS and your host to this season as we contemplate the imperative for responsible AI.
Each episo-- yeah, each episode, we're joined by an expert to explore a different facet of the ongoing quest to ensure artificial intelligence is deployed fairly, safely, and justly for all.
Today, we are joined by Shalini Kantayya. Shalini is a storyteller, a passionate social activist, and a filmmaker who documents some of the most challenging topics of our time.
I've also been blown away by her amazing ability to tackle sensitive topics with serious humor. Shalini, your short, Doctor In Law, made me laugh out loud.
More recently, Shalini directed the film, Coded Bias, which debunks the myth that AI algorithms are objective by nature. So welcome, Shalini.
SHALINI KANTAYYA: Thanks so much for having me. It's an honor to be here.
KIMBERLY NEVALA: So have you always been a storyteller?
SHALINI KANTAYYA: I have. [LAUGHTER] I think all of us are storytellers. And we all have stories.
I think there's a saying that says, the universe is not made of molecules. It's made of stories. I'm a scientist, so I believe both are true.
But I think that stories are something that we've had and passed on since we had fire. It's one of our oldest human traditions. And it's how we engage with issues that are difficult. And I think it enables us to cross boundaries and to translate our values.
KIMBERLY NEVALA: And what drew you specifically to the format of filmmaking and documentaries in particular?
SHALINI KANTAYYA: Well, I think that cinema and filmmaking is an extension of my engagement with the world. And I think that films are the sharpest tool that I have to make social change.
And I sort of believe that what Roger Ebert, the film critic, said that films are empathy machines, that we sit in the dark, and we identify with someone radically different than us.
And in that seat of empathy and that power of emotional feeling that films invoke is the seat of social change. And Bertolt Brecht said, we do not act not because we do not know what lies beyond the factory gate, the prison wall. We do not act because we do not feel.
And so it's my hope that in the films that I make that I create these complex characters that spark emotion and empathy and make us feel.
KIMBERLY NEVALA: That's really interesting, I think, particularly in the context of what we're talking about, which is artificial intelligence and algorithms and math, which can feel very cold and very distant.
You've said that before Coded Bias you didn't know what an algorithm was. And I imagine now you see them everywhere. But how did you get interested and involved in this project?
SHALINI KANTAYYA: Well, you're absolutely correct in that three years ago when I started making Coded Bias, I did not know what an algorithm even was. I think my street cred in the game is that I'm a science fiction fanatic.
And so everything that I knew about artificial intelligence came from the imagination of Steven Spielberg or Stanley Kubrick. I'm definitely someone who's watched Blade Runner way too many times.
And I don't think that my background as a sci-fi fanatic really prepared me to understand how AI is being used in the now. And I stumbled down the rabbit hole when I read a book called Weapons of Math Destruction by Cathy O'Neil.
And I don't think until then I really understood the ways in which algorithms, machine learning, AI is increasingly becoming this invisible gatekeeper of opportunity and the extent to which we are trusting these technologies to make decisions about who gets hired, what quality of health care someone receives, even what communities receive on due police scrutiny.
And that's when I really-- I think that at the same time as I was learning about the ways in which we are outsourcing our autonomy to machines in ways that really change lives and shape human destinies, I came across a Ted Talk that Joy Buolamwini gave and realized that these same systems that we are putting our blind faith in have not been vetted for racial bias or for gender bias.
More broadly, they haven't been vetted to make sure that they won't hurt people, that they won't cause harm. And I was kind of alarmed to learn that in many cases these systems haven't even been vetted for some shared standard of accuracy outside of the companies that stand to benefit economically from their deployment.
And that's when I really began to understand that everything that I love, everything that I value as a free person living in a democracy, whether it be access to fair and accurate information, fair elections, equal employment, civil rights, that communities of color aren't over brutalized by police, over scrutinized by law enforcement, that all of the things that I hold dear about justice and equality and civil rights are rapidly being transformed by algorithms and by AI.
And that's when I really began to see quite what Joy Buolamwini articulates so clearly in the film Coded Bias that we could essentially roll back 50 years of civil rights advances in the name of trusting these algorithms to be fair, to be accurate, to be just, to be unbiased, when in many cases, that just isn't a fact.
KIMBERLY NEVALA: Yeah. And I think in Weapons of Math Destruction and in some of the other discussions that I've seen, one of the interesting conversations-- and I believe you've spoken about this as well-- is that this is really innovative technology.
But in a lot of cases, we're applying this innovative technology to historical practices. Or in some cases, we're predicting and, therefore, recreating the past with these technologies.
And you certainly in the film give a lot of examples where the technology is entrenching historical bad practices and stigma and biases. Are there also examples that you've seen though in this journey where the alternate is true- where we really can rethink what is possible and create a normal that's truly new?
SHALINI KANTAYYA: It's such a good question. And I think human beings can do that. But machines can't.
I think the only way to try to train an algorithm is off data from the past, a past that is written with historic inequalities. And sometimes, even in spite of the best intentions of the programmer, these systems pick up on those inequalities.
Take for instance the algorithm that Amazon created to level the playing field. It was designed actually to make hiring more fair. And lo and behold, the system starts looking at who got hired, who got promoted over a number of years, who got retained.
And lo and behold, it starts to discriminate against anyone it can discern is a woman. And just the fact that that can happen in spite of the best intentions of the programmers is a testimony to the crude logic of how these algorithms work.
These models are essentially sorting us into these crude categories based on past behavior and based on people like us or that it perceives like us. And I think that sort of crude logic of how algorithms work prevent it, inhibit it from having the kind of transformative power of creating a new future that you're talking about.
And I think to create a new future, that might require some intelligence. And I would define intelligence differently than I think that math conference in 1956 where they said what artificial intelligence is.
And I would question any system that doesn't have ethics, that doesn't have morals, that doesn't have empathy or compassion. I would question any system that doesn't have those qualities as being truly intelligent.
And so I think it should be in the hands of human beings to shape a more transformative future. And I'm not sure that machines can lead us to do that.
KIMBERLY NEVALA: So it sounds like by extension, or maybe just restating what you've said in a different way, that even-- because Coded Bias is looking at examples where, for instance, facial recognition performs differently based on the color of your skin, based on your gender, and particularly on that intersection of gender and color of skin.
But even if those systems work perfectly, it sounds like you also think we may be over-indexing just on this idea of making them fair or unbiased. So am I hearing correctly that you think there's some categories of applications that just fundamentally require more due diligence or more nuanced and vigilant discourse before we shoot down this path?
SHALINI KANTAYYA: Absolutely. I want to just start by saying I'm a huge tech head. I made this film because I love technology. And I do think it is rapidly changing our world.
And I think oftentimes I'm posed with the question, don't you think there are good uses of this? Don't you think there are applications that could be fair and unbiased and make our world better?
And I think absolutely. I think the question that I hope Coded Bias poses is who gets to decide? Who gets to decide what is a fair and unbiased application? Who gets to decide when we use facial recognition technology?
Right now, what we have is a system where Big Tech is selling directly to law enforcement. In some case, Big Tech is deploying at scale with no safeguards in place. And I often make the analogy, just because I believe in an FDA, a Food and Drug Administration here in the US doesn't mean that I don't like food.
It means that I believe in a certain standard of safety and a standard that protects the health of all people. And what I believe is that in the realm of algorithms, machine learning, artificial intelligence, that we are in a wild, wild West, an unregulated sector of society unlike any other.
And I would liken it to maybe the automotive industry before there were car seat laws and seatbelt laws and a car seat for your baby. It's like having pharmaceutical products with no counter indications, no usage labels on them.
I think that is the kind of unregulated sector that we are seeing with artificial intelligence. So it's not that I believe that these systems couldn't have positive applications. It's more my concern that there are no laws in place that would give us guidelines for the industry to commit to that as the center of their business practice.
KIMBERLY NEVALA: So certainly we've seen a profusion of AI ethics, principles, and frameworks. And we're now starting to see, I think, the leading edge perhaps of more solid AI regulation, even in the context of, for instance, facial recognition where it's been banned in certain municipalities and countries.
And it's an ongoing debate. In the time since you've made that film, have you seen progress in other areas, glimmers of hope for us to be able to do this? Because certainly just as, I think, whatever we may feel, cars are on the road and weren't taken off.
Unfortunately, we used to do data governance strategies. And I would tell companies, and I hated to say this, that sometimes companies are ready and willing to get in front of it. And sometimes they have to feel a little pain. And maybe that sounds a little cynical.
But sometimes you have to feel that pain. And I think we've seen a lot of examples now. But certainly, I don't think AI is going away. Are we making progress? Are there some glimmers of hope in terms of getting-- being more mindful in developing both mindfulness and regulation? Because I'm not sure that one or the other does it by itself.
SHALINI KANTAYYA: Absolutely. I think I make documentaries because they remind me that everyday people make a difference and that not all the heroes among us are wearing superhero capes.
And I've really seen that in the making of Coded Bias. Since the film released, I've seen sea change that I never thought was possible. Three of the largest technology companies in the world changed their policy of selling facial recognition to law enforcement.
IBM disrupted their entire business model and got out of the facial recognition game. They're done. They won't research it, deploy it at all.
Microsoft stopped selling facial recognition to law enforcement. And Amazon took a one year pause for which we have a few weeks left until June 2020 when their moratorium is up.
But I think that sea change became possible because of three things that give us a recipe about how we can move the dial. One is we need brave scientists unencumbered by corporate interest.
I have seen a pattern where big technology companies often attack, discredit, and dismiss independent science that seeks to shine a light on AI and unbias in AI and towards-- bring us towards greater ethics before they are believed.
And so we need people like Dr. Timnit Gebru, like Joy Buolamwini in the room where these decisions are being made. And also, I think it's significant that three Black women, all of whom were graduate students at the time, shine a light on bias in AI that somehow Microsoft, IBM, and Amazon missed.
And that speaks to the power of inclusion. I think one of the things that I've grown in compassion for is that bias is not just in some bad people. But it's an inherent human condition that we all have, and many times is unconscious to us.
And so that means that inclusion is not just something that's good for the pictures. But it's absolutely essential when we are building innovative technologies that are deployed on the world when we need to control for the inherent human condition of bias.
And when you have an industry that is less than 14% women, and I couldn't even find statistics on people of color, frankly, half the genius is missing from the room. And half our imagination has been missing from the room.
And so I think part of the issue is that Silicon Valley has an inclusion crisis. And that should be a-- I hope translates into a major campaign, investment campaign to women and people of color in the technologies that will define our future.
So we need those scientists. The other thing is that we need massive AI literacy. And I'm so grateful to programs like that, this one, that seek to educate the public about these issues. It's one of the reasons that I make films.
And I think when it comes to AI, all of the knowledge has been in the hands of the few. So all of the power has been in the hands of the few. And it's my belief that when a 10-year-old starts using a phone, a smartphone, that's the very age that we should start talking about data collection, why he's getting that ad on Google, and someone else is getting some other ad.
And start talking about basic AI literacy from a young age so that we can start to challenge these systems by first understanding how they work. And I know that in my own process of making Coded Bias, I'm so grateful to the brilliant cast of my film for schooling me.
And now I can start to ask really critical questions and discern what might be legitimate uses of data science from what is bogus, bologna pseudoscience being sold by corporations that just want to make a dollar.
And then the third thing is that the change of these companies changing their policy, three multinational tech companies changing their policy happened in June 2020. And that timing is really important because Joy's research had been out for two years. My film had been out for six months.
And I think that timing is really important, because that's when you had the largest movement for civil rights and equality here in the States and all over the world where people of all colors were in the streets, locking arms, and standing up against racial bias.
And I think those three things coming together meant that people were making the link between racially biased, invasive surveillance technology in the hands of law enforcement with no one we elected giving any oversight, with no one that represents we, the people, giving any guidance and the communities that could be most harmed and brutalized by these type of technologies.
And I think that gives us a recipe of how we can move the dial. We need brave scientists. We need a massive inclusion campaign. We need massive science communication around AI, not just for PhDs, but for all of us, for your grandmother and your 12-year-old.
And we need to be engaged people of a democracy that push our policymakers to keep pace with technology.
KIMBERLY NEVALA: And it's interesting. We had the chance to talk with Teemu Roos, who runs a course called Elements of AI, and is very involved. He's a machine learning researcher and very involved in the Finnish National Education program.
And his comment was that his course was based essentially or targeted at everybody that wasn't interested in AI and everybody that wasn't in technology. And also had the opportunity to speak with folks like Tess Posner who is the CEO of AI4ALL for all about developing the next generation not just of data scientists but of leaders and of thinkers and of analysts.
I'm interested in your own experience in that journey of discovery you went on, what most surprised or challenged your thinking as you learned about artificial intelligence? And what can we learn from your experience and perhaps from your experience as a storyteller as we work towards making this topic accessible and creating broader public literacy and citizen engagement and advocacy?
SHALINI KANTAYYA: I think I had often heard these issues framed around privacy. And that's a word I have almost no emotional sort of attachment to. And I think the more accurate term is invasive surveillance.
And I did not realize the extent to which these companies can create almost a complete psychological profile about us. I mean, you have companies that scraped the internet and looked at people's dating profiles and then created an algorithm that said it could judge whether you are likely to be gay or straight only on that binary.
And with accuracy they could judge whether you were, with 91% accuracy in men and I think 82% accuracy in women, your sexual orientation. So you can see the way in which these systems could be a danger to vulnerable communities.
We often talk about the Cambridge Analytica scandal here in the US where you had a company that essentially created the psychological profile of a swing voter and targeted the 100,000 people that it predicted had the psychological profile of a swing voter and just pushed misinformation at them.
And so you can see how that can be a threat to democracy, how it could be a threat to mental health if it knows the thing that you're vulnerable to at the moment you're vulnerable.
You could be diabetic. And it is picking up on your erratic search patterns and knows you have low blood sugar and is just pushing that soda and those potato chips in front of you.
And so it's that kind of thing that makes us all vulnerable to these technological systems that are opaque to us where all of the power is on one side. So that was one thing that was startling to me.
And the other thing is that I could not-- I was so shocked when I came to understand how these systems work and how we trust them to do big magic that they're not capable of doing.
So for instance, predictive policing. It's saying, trust us. These softwares by Palantir saying, we know where crime is going to happen. And I can now look at that and say, well, wait a minute. I live 10 minutes from Wall Street. And I can tell you that we don't have crime data. We have arrest data.
And that's really different. And if you're using these algorithms to look at where prior arrests have taken place, are we just sending police back to those same communities?
Daniel Santos, a teacher in my film-- you can stand 10 feet away from this man and feel his commitment and passion for teaching and what a great teacher he is-- was misjudged falsely as being a bad teacher by an algorithm and had to defend himself.
It was always like being guilty before he even had a trial. He was denied his due process, which is why the court, when he challenged it, overturned it. Said, if you are going to fire me, you have to tell me why.
And it becomes a violation of due process. And so many times, we're getting these invisible-- now they're pushing these algorithms in higher education.
And I was on a call where a teacher said, I just got this star next to a student's name. And the software told me that the student was under danger of being disen-- unengaged, inattentive.
And the teacher said, no, this student is blowing up my text message. This student is very engaged. How do I override this?
But in algorithms are just putting these-- it's this gentle hand-- not even gentle, invisible hand of power in our lives. And that is what is so astounding to me, is that we've not examined this invisible hand of power nudging us along paths that are designed by power.
And I think that is what was most alarming to me in the film.
KIMBERLY NEVALA: Yeah. And there's an aspect there that-- as you said, you had earlier talked about we tend to put this in sort of a bloodless sense, it's data privacy, which doesn't necessarily resonate with people.
And yeah, we get it. But we don't. But when I talk with friends and family, they're still horrified that they can Google themselves and some of the information they can see.
And I've said previously on numerous occasions that I'm not sure if I'm a good or bad friend, because I don't know if I should disavow them of their sense of privacy. Although, I do. I'm not sure they always believe me.
But we also, I think, need to look things very holistically then. So we talk about data literacy or technology literacy or AI literacy. It's not just about understanding information and data, but bringing those outside voices in to say-- that really represent the situation and help us figure out how to interpret the data.
Because data in and of itself is not subject to just one interpretation. And as I think you're alluding to, you need to understand the context around that, whether it's the historical context or sort of the conditions on the ground, if you will, especially if you're going to play this in a human context.
Now, in the film, there's a woman who really welcomes algorithmic social scoring. And one has to assume she firmly believes her own score would be high. And therefore, such a system would be non-punitive to her or others that she knows.
But that aside, how important is it for us to include contrarian views, or at least views that are contrary to our own perspectives and experience in any or all discussions of AI?
SHALINI KANTAYYA: It's really important. I mean, I think that that episode in China is sort of a Black Mirror episode inside of the documentary. But also, I meant it as a mirror to hold off that's really a reality closer than we often think.
And I think there's part of us that are like, wow, I could buy a candy bar with my face. That would be really cool, without thinking what we're giving up in this race to efficiency.
And I think it was important to include that view. Because I think that she's pretty indicative of, I think, where the next generation is around privacy. I spent a lot of time with Gen Z kids.
And they've been blowing my mind. Because they think more like that citizen of China than like me around data rights. And I think that this new generation of digital natives have a really different view of technology.
And they don't have this preciousness around certain things being private, certain things being personal. And I think that was really important to include.
And as a filmmaker, I really seek to tell nuanced views. Coded Bias is not a gotcha film. It does not seek to look at Big Tech as this absolute nemesis of humankind.
I know that there are very many brilliant, well-meaning people that work inside these companies. And instead, I think the film seeks to ask questions and engage those questions from a number of perspectives and allow the viewer the space to make their own decision.
And that's very much what I seek to do as a filmmaker. Because I think in art is a place where we can cross boundaries and engage with people who think differently than us. And I think that's the beauty of film. And I don't want to lose that dialogue with people who think differently than me.
KIMBERLY NEVALA: And I thought that was-- it re-emphasized that idea that my values aren't your values. And they don't need to be. And we don't necessarily need to agree. But we should probably have the discussion, but also the importance of being open to sharing those broadly and putting those out there, regardless of your own point of view.
But those can be very sensitive and sometimes difficult conversations even to open yourself up. Are there tricks or tactics you can pull from your hat as a documentarian or as a storyteller that we can use to make these conversations more palatable and more productive?
Because we need to have more of them moving forward. And you're a truth teller and a storyteller. And I'm wondering if there are some best practices for helping to better engage folks in these conversations.
SHALINI KANTAYYA: Oh, I so appreciate the question. Because I think that oftentimes in social media, we're losing that nuance of speaking to people who think differently. And we never grow as people unless we engage with people who think differently than we do.
To me, as a storyteller, I always-- although all of my films, every single one of them, could probably be called political in nature, I never ever start a film with politics. I always start with a person, a character who goes on a journey.
And I try to tell even the story of technology and really complicated politics from personal stories about human beings. And I think in Coded Bias, you may not care about bias in AI.
But you watch the film, and you care about Joy. And you go on a journey with her. And I think it's that engagement, that experience of empathy for someone who is radically different than us, that feeling of identifying with their experience that is the spark of social change.
And I think the more that we can do that, to tell stories that are about people and to pose questions around their journey. And I think I do try to present evidence. But I also try to ask more questions.
And oftentimes, when I am on panels with people, and they're asking me all the answers, I'm like, my job as a filmmaker is really just to ask the questions.
And I really see that there's a beauty in that. Because we have some hard problems to solve. And we need to see into the gradients of gray. It's not black and white.
And the more that we can see into those gradients, the more that we have a chance of solving these complicated problems.
KIMBERLY NEVALA: I think that's such a great take away and probably a great place to start as well. I think for even those of us working in the corporate world, just remembering that, the humanity at the heart of all of it and bringing it back to that.
Because at the end of the day, the technology is intended to be used and serve humanity in one way or another. So thank you, Shalini. I really enjoyed the conversation.
I think your-- honestly, your journey into the innards of AI algorithms and more important, I think, the centering that on the human experience is so enlightening and important for all of us.
So thank you for bringing that to the masses. And if folks haven't seen Coded Bias yet, I would encourage you to check it out now.
SHALINI KANTAYYA: Thanks so much for having me. It was an honor.
KIMBERLY NEVALA: Thank you. Now, next up, you can join us for an illuminating conversation with the Director of Intel's Human and AI Systems Research lab, Lama Nachman. Lama is passionate about the use of AI as a collaborator, not a competitor.
And she is doing some of the most impactful work in applied artificial intelligence today, including giving people back something most of us take for granted, which is our voice. So make sure you don't miss her by subscribing now to Pondering AI in your favorite pod catcher.
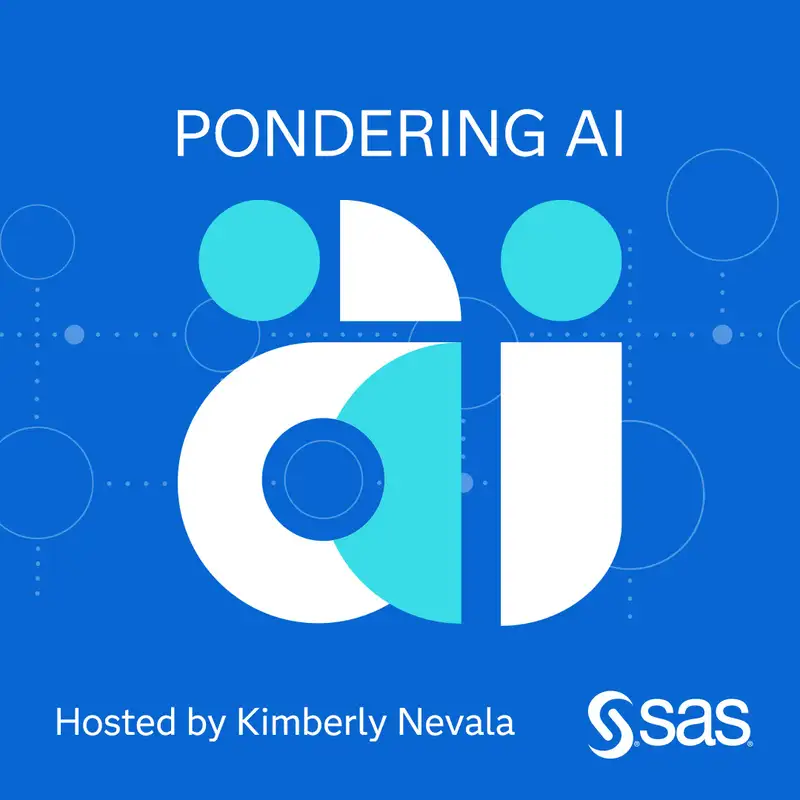