Artificial Empathy with Ben Bland
KIMBERLY NEVALA: Welcome to Pondering AI. I'm your host, Kimberly Nevala.
Today, I am so pleased to bring you Ben Bland. Ben is the co-founder and partner at Loopers where he spearheads digital strategy, communications, operations, and ethics. He is also the creator and instructor at a fantastic AI ethics for leaders course. And perhaps, most importantly for the conversation today, he is the chair of IEEE's Standards Working Group for Emulated Empathy in Automated and Autonomous Systems -- I think it's Autonomous and Intelligent Systems. It's a mouthful.
Ben joins us today to discuss the premise and the promise of empathetic or emotive AI and to balance that against the realities of this tech today. And as I've said to you Ben, and this audience is well aware, I have some feelings about this topic. So I'm absolutely chuffed, if you were, to have you with us today. Thanks for joining us.
BEN BLAND: Thank you. It's a pleasure.
KIMBERLY NEVALA: So to kick things off, can you tell us how you came initially to have an interest in the topic or study of emotion in human machine interaction?
BEN BLAND: Sure. I mean, I've been interested in technology my whole life and been working in the area for the 20 years. I've been working and always pursuing the more interesting and meaningful tech that could have a major influence on our future.
But I ended up at a startup here in Belfast that was trying to build a comprehensive machine, an engine for measuring and estimating people's mood based on various biometric inputs, and thankfully, working with the founders who actually wanted to do something good with it. We were faced with a lot of interesting challenges as we had clients that were big commercial entities and whatnot. So we started looking for how we can get ahead of this industry before it really becomes something global and that led us into working on ethics and so on. And even though that company didn't make it through COVID, I've continued to work in the area of standards and ethics and so on in this space.
KIMBERLY NEVALA: Now, before we talk about emulating empathy in machines or the use of emotion in AI systems, I'd like to touch base on the current state of research into human emotion itself. There's a lot of discussion about whether these types of applications are sitting on solid scientific ground or not.
So what is the current state, if you will, of our understanding of human emotion, and are there underlying issues to be aware of as we move forward in this space?
BEN BLAND: Yeah, it's really important for understanding what this technology might be capable of today and what it might be capable of in the future, and more importantly, what it might not be capable of.
The whole field of emotion science, it's a major part of psychology and machine learning and neuroscience and all these kind of fields. But there's still a lot of contention over what are the best models for the things that we feel. Are emotions even really a thing, or are they just a sort of composite of physiological phenomena? What is the difference between, say, the expression that we give around our emotions, a facial expression or a hand gesture or whatever, as compared to something that we actually feel? And even those feelings themselves, I mean, how do they actually manifest beyond being just a sort of cluster of nerves, for instance?
So it's very much an open debate. And so any technology that is being built on top of it has to be built on that kind of rocky foundation, shall we say.
KIMBERLY NEVALA: And so with that in mind, more philosophically, what does it mean if we say that in a machine or an AI system is empathetic? I keep saying empathic, which I know is not the right word. But can a machine, in your estimation, really have feelings?
BEN BLAND: Yeah, right. Well, actually, on the wording thing, we get stuck in this all the time. I've been pushing for the empathic one, actually, not for any particularly good reason other than that it's more succinct. But they seem to be interchangeable for some people. But then when you talk about empathic, sometimes people think about telepathic and superhumans and stuff. But anyway, that was not the intention.
Yeah, no, the easy quick answer to whether or not machines can feel or empathize - and this is where a lot of people in the community that I sit in who are trying to develop standards and regulations and so on in this space - is, no, they can't. It's different. Humans and other organisms, potentially, empathize in different ways, but machines are different.
But the more complex answer is that yeah, well, sort of. Because, I mean, long-term, who knows? It's hard to say at what point this so-called intelligence of modern machine systems will demonstrate capability of understanding or responding to or even potentially emoting in some way; feeling something that we couldn't really differentiate from our own or in some way we need to recognize.
But even in the short and immediate term, there are systems that, whether designed for this purpose or not, will respond in some meaningful way to something like a felt state in a person. And as such, or even just as a way of understanding them, even if you take away the emotions out of it, that you could fit into a broad definition of empathy. And so, as such, empathy is a part of the way intelligence systems behave today.
KIMBERLY NEVALA: And when you think about the range of applications and patterns…or maybe it's use cases that you're considering. When you're thinking about empathetic/empathic/emotive machine applications, what does that look like? I tend to oversimplify and say these are applications or elements that either try to detect or to project an emotion, a desire, a need, whatever that may be.
But when you think about the spectrum of applications we're considering here, what does that look like? How do you define it?
BEN BLAND: The obvious one to start with is mental health and wellbeing because there's a clear case to be made to at least attempt to use technology to help people to better understand their emotions, to develop a more stable and comfortable and healthy psychological and physiological well-being. Although, of course, there are questions around the capabilities and whether it's OK to replace humans at such jobs and things like that.
But there are loads of other use cases, either currently in deployment or late stages of development, that include education. There have been trials of systems to try and work out if kids are paying attention or not while they're learning. There are deployments in connected cars, in mobility systems that look for things like sensible things like fatigue. But also more kind of nuanced things like stress, which can affect your capability to concentrate and so on. And whether you include something like fatigue or stress as an emotion is all part of the debate, right? It's a felt state in some form, but that's a different question.
There's use in employment along lines of worker performance, but also things like customer service. Like is the tone of your voice correct for the way that you're treating this customer, or indeed, is the customer getting angry in the way that you're treating them, and so on.
And I'll give you one more example. There are other elements of performance that might be less, let's say, ethically questionable around things like athletic performance. The emotions are linked in some complex but very meaningful way to how we perform in the field. And therefore, if I was an athlete and I had somebody - my coach is going to be working with me on an emotional level as well as a physiological level - I mean, they're all interconnected. So there's a use case there to do things like attempting to measure what state you're in before you perform.
KIMBERLY NEVALA: I want to somewhat stipulate that that we might be able to simulate emotion or perhaps detect…and maybe we need to talk about those two elements differently. The ability to try to detect an emotive state or an emotional state and then do something with it. And maybe that is just detection to influence, to either influence behavior or to relate to somebody. Then there's the issue of projecting emotion, which has a lot of ethical stickiness, I think, across the board.
But when you're thinking about the use of systems to emulate emotion or to detect emotion, there's clearly some potential big wins, there are a lot of potential big underlying troubles and we need to balance those two things out. So maybe we can talk about each of those. What are the potential big wins and the big value or benefits of pursuing this type of technology?
BEN BLAND: Yeah, there's a sort of spectrum, to use the word again, between the good and the bad cases that often overlap with each other. The same thing could be seen in different ways.
But as I see it, most of the systems developers that are pursuing this kind of technology seem to be thinking about more naturalistic and more human interaction with machines. So machine systems that can be more personalized to our particular behaviors and our particular needs and so on. They can be more naturalistic in the way that they converse with us, which we see with large language models and things like that. There are empathic elements from understanding and from feeling that sit within a better communications between people. So there's that kind of naturalistic and human and personalization case.
But I think the really big one is even today, if a system can, let's say, guess at some state of stress in you from a change in heart rate. It already has a capability that could fit under this umbrella of empathy in a broad sense that is superhuman. You can't do it yourself even on yourself, really, let alone other people detected externally. And it can, then, respond in some useful way, like to attach it to, let's say, an event in your calendar that says, right, well, once a week you go and visit that person and you seem to be getting stressed about it. Let's work on that. We have the potential then for something that acts in a way that a very good friend does or a very good counselor or somebody who is developing an understanding of that even you can't pick up on. Then, with a two-way interaction with that system, especially if you can actually give critical feedback and say, no, you're wrong, this is not the case and have your own subjective viewpoint on that. Then we could get into this evolving emotional intelligence capability between us and the systems we interact with that I think could be really powerful in moving forward.
KIMBERLY NEVALA: Does that also preference or bias a certain type of, I don't know if it's human performance. With other aspects of machine learning, we talk about this progression towards the average or towards the mean. And for a long time, in the workplace even, we've got this long-standing debate, are extroverts or introverts better leaders? Well, it's not that they're better or worse leaders, they're just fundamentally different.
So how do we manage…I mean, is that even an issue? Is that something that we need to be really careful of here? You've got all kinds of folks that are neurodiverse and we all just operate in slightly different ways. So how do you balance that natural diversity, if you will?
BEN BLAND: Yeah, I think this is an enormous issue. I think it's a subset of a broad problem around how these systems could potentially be very powerful and in very subtle and subconscious ways. We talked earlier about the questionable foundation on which effective modeling, should we say, is built in the first place, and that we haven't figured out the science of it yet.
But then, there's actually how the system then behaves. And for instance, people deal with things in their own ways. People, to some extent, may need to go through negative experiences to learn about how better to deal with them or even just for a more balanced experience of the world.
If you constantly push towards what our quote unquote "desirable states" - like if you have a system that's actually capable of making you feel happy or 1% happier all the time - then theoretically, you could become more desensitized to that more positive state. And I use ‘positive’, again, inverted commas, because it depends on what's appropriate. It's not a bad thing that we do have negative feelings.
So yeah, there's an underlying vacuum of model on which you would base that kind of counseling and psychological support type behavior that is quite dangerous. And leaving it up to engineers, essentially, to summarize, to take that control, is pretty sketchy.
KIMBERLY NEVALA: So you've been working, building, investigating these systems since at least probably around, I think, 2016, is that correct?
BEN BLAND: Yeah.
KIMBERLY NEVALA: What is the current state of the art of the technologies, and what are the boundaries right now that we need to carefully mind or the gaps we need to carefully mind versus what's the PR and the hype about what we can do?
BEN BLAND: Well, I think it is still kind of in that hype stage. Even back in 2016 to 2021 when I was directly involved in building these systems, we were surprised to see that the market still hadn't lifted off. We were kind of early, which was odd because around that time there were some big purchases by some of the big tech companies, for instance, of similar organizations. And then, things went rather quiet.
And my best guess is twofold. One is the slight fear of negative sentiment towards this, to use the term. People might get freaked out by their phone suddenly saying, as of today, I will try to guess your mood. But also, then not really being that kind of killer application yet that's driven it forward.
But there are subtle ways in which you see it start to drift in. Even as far back as when we started to get the kind of emotive expressions that you can add over your face. There's facial detection going on there that could be used for coding based on facial expressions like smiling and frowning and stuff like that, which is a pretty basic way of doing mood estimation. And there are challenges around that too, but I'll not get into that just now.
Then, in recent years, we've seen increasing capability for sensors to be able to detect more subtle metrics in the body. We had poor quality heart rate sensors on our smartwatch before. Then they got better and better. And now they can pull out things like heart rate variability with reasonable accuracy, which is much more closely connected to things like stress and whatnot.
But it's worth noting that while we're now 2024, it still feels like this industry hasn't really taken off. And maybe there's some fundamental reason for that but I suspect it's more just the confluence of things standing in the way. But we have seen something that feels like a qualitatively different situation with this new generation of let's call them general purpose AI, so the large language models like ChatGPT and the multi-modal models, Gemini, GPT-4o, and whatever. Where you see an example of a system that can behave in a way that may look a lot like empathy, right?
Whether it's expressing emotion; like it says, I know how you feel or that's upsetting, you shouldn't say things like that to me. This is the system talking. Or responding to our own expressions of them. And it could be something really subtle, like a choice of word that we are completely unaware of. And in such a way, systems like that probably weren't deliberately designed to do that. The developers probably can quite fairly say, well, that was never our plan. But it is inherent in an implicit empathy within the system, which we think-- some of us think is changing the game and we really need to start to spread the word about the controlling and managing how we deal with these systems in the world.
KIMBERLY NEVALA: Even the system responding with I or I think you feel or perhaps you should, that basic use of language, depending on how you look at this, can be seen as just natural and trying to enable a more interactive, intuitive experience for the user. Or it could be seen as coercive because it is purposely, to some extent and by design, influencing how we perceive the entity on the other end.
So I don't know if you have -- where you come out on that spectrum. Is it just the nature of the beast in your mind?
BEN BLAND: I mean, recently when OpenAI announced GPT-4o, what was it? A day before Google did their Gemini announcement. No coincidence, I'm sure. It was a perfect example, I think, of what you're talking about there where you could see demonstrations of two systems released more or less simultaneously.
And there was this big debacle around the fact that the GPT-4o system sounded like Scarlett Johansson's voice in Her, the movie. But put that aside for a second. It showed these elements of anthropomorphism referred to itself as I and the voice tone changed and it really…because it's quite emotive. And the Gemini 1 was slightly flatter, less emotive, more passive.
So you can (hear) this spectrum between really gregarious and emotive to neutral and flat. I think those have their own places. Personally, just for my own perspective, I think I prefer a system to just shut up and get on with the job and not pretend that it has feelings because I find it draining. And there is an issue of empathy and emotional exhaustion, fatigue, and whatnot.
But a lot of this lends itself towards a problem that I keep going on about it, but I haven't actually got much to say and exactly how we solve it. I call it sophisticated agency. It's this idea that we as agents, as users of the system, are better protected and there's less problem if we really understand what's going on in some meaningful way and have some control over it. But it's easy to say that and very hard to do in practice.
And I sympathize with anyone who's producing these kind of things. Just look at the cookie consent forms that we have to fill out. We just ignore them and so on. So if you try to stop every time you encounter a system like this and tell the user this is what it's going to do, are you OK with it, and so on. That's very hard to do. But it's also really important because people need to not be duped. We fool ourselves very easily, even unintentionally. Some would even argue that you could build in some kind of variation in how emotive the system is and allow that as a user option, which is a lovely idea. Again, in practice, it just adds complexity to the design, though. So not necessarily easy to do.
KIMBERLY NEVALA: In your prior remark, you made the comment that folks working in the field - like yourselves, the teams that you work with and you do research with, a very broad swath of folks - felt that this latest iteration of the multi-modal type LLMs were a qualitative sea change. What is it about these or what is that change, I guess, is a better way to ask.
BEN BLAND: Well, it partially relates to the fact that before a lot of what, at least what I understood, to be this kind of empathic realm of autonomous and intelligent systems, AI, right? It was quite a specific thing where there was a metric or a signal from, say, your body breathing rate, whatever. Or from some other input such as the text that you input into the computer. So you can use things like sentiment analysis.
Then, there was this deliberate design to put that into a model that mimicked some kind of psychological model about, let's say, valence and arousal is a big one. Valence being positive, negative, and arousal how highly or low aroused you are in a particular state. You can pretty much map the emotions onto it. That's the theory.
Now we have this case where that doesn't seem to be necessary because we see this emergent - let's call it intelligence - that comes from throwing machine learning at a big enough corpus of language or at enough images to some labeling and things like that. So one can easily imagine - and it's already happening - people are interacting with these systems and developing relationships with them. Developing an emotional capital there that could be badly affected by, let's say, somebody taking them off the system. Shutting the system down. And that's happened before. People have complained when systems have been shut down.
But also, it can have these poorly explored outcomes in your social and psychological life that there is some evidence for but we still don't really understand that well, which is desensitization. You might find a dependency on a system that is constant, that has no infinite patience and is always nice to you compared to real humans who might not be so. And that's an important fact of the human world that we really need to get our heads around.
KIMBERLY NEVALA: Are there any red lines today, just given the state of the understanding of the field at large machines aside and where the technology is today, are there red lines you think we should just not be crossing in terms of whether that's hypothetical, logical, or specific application categories?
BEN BLAND: Yeah, but the trouble, I think, is that as soon as you start to define them and enumerate them, you create potential get outs.
From my very limited reading of the EU legislation that came out recently, which does draw red lines at a legal level, where they've tried to define what they mean by these systems. They've talked about emotion recognition. So just as we've talked about this more implicit version of empathy that you might get from a system like an LLM. That doesn't need to be actually saying I have detected 40% anger in any kind of model. But it might, nevertheless, say that this person is using more negative words, or their voice pitch has changed. Then, so the employer and the workplace where it's banned or the teacher in the educational institution where it's banned might respond according to that. They've taken on themselves to say like, well, I think you're getting angry here and you shouldn't be or you're not paying attention or whatever. So it is very difficult to draw those lines.
I mean, if you take my earlier description of what's the really good use case here. This idea of having empathic companions that live with us all the time, as I say, have infinite patience and can look out and look at the context around our psychophysiological states and say, I see it. Not only can I associate it with particular events in your life but I can also go out and look for remedies and things like that. But equally, that serves, as I see it, the ultimate product. And I think this is how commercial corporations and consumer organizations are seeing this.
Ultimately, sorry, I'm going off on a bit of a tangent here, but I think it should come back around to our point. If you think about business in general, ultimately, business is about guessing how somebody feels or guessing about what they want or when they want it or whatever. Getting inside their head, wearing their shoes, wearing their glasses, walking a mile in their shoes, whatever. And then, giving them something that is pitched in the right way that they will then buy it from you, essentially. That sounds not too far away from this kind of emotive companion thing or empathic companion thing where if you attach your efforts to guess at somebody's emotion or to respond even without the direct measurement to, let's say, a sale, then the system is likely to just move towards some optimal state. Where it will present the marketing material to you in just the right way at just the right time when, let's say, you're tired, you're vulnerable, you're lonely, and then sell it to you.
So there's a sort of line at which we have to, somewhere along there, we have to stop and say this system is either deliberately designed or likely to be used in such a way that it is going to be coercive for people. And you can say the same thing about politics. We've seen this with Cambridge Analytica scandal. But that's using something that wasn't nearly as subtle and potentially manipulative as something that would get at your feelings and attach that to voting behavior.
We can look at outcomes and draw red lines around the potential outcomes of a system and we can look at their capability in their design. And as I say, it's not just what they're deliberately designed for, but what they can reasonably say they're capable of. If it's coercive or if it brings about extreme states.
I'll throw another example, which I use all the time. If you look at a video game that is designed to change some of the metrics like how difficult the game is or how much your firepower is or whatever it is. Metrics in the game that change according to some physiological state that you're in with the aim of it being more exciting or more enjoyable. That's one kind of metric. That, on the face of it, isn't necessarily a particularly challenging use case. But nevertheless, we talked about desensitization earlier. What if you enjoy it so much become addicted to the game or you become desensitized to real life enjoyable states and things like that.
There can be cases where this extreme use or overuse, where that's possible, we have to find a way to manage that, whether it's restricting the use or just restricting deployment in the first place.
KIMBERLY NEVALA: Well, and as you said, the AI EU Act does have some very specific call outs for prohibited uses of this type of technology. Although a lot of it is predicated or hung on the hook of intention and that is always somewhat difficult to prove and it can be fairly subtle. We had a really interesting conversation with Marianna Ganapini just recently about nudging and some of the intentional and unintentional and why that's so hard to detect.
I think that's a good turning point to talk to the work that you're doing with IEEE and the Working Group. Again, that's the Standards Working Group for Emulated Empathy and Autonomous and Intelligent Systems. I'm reading that straight off the page because it's long and a tongue twister. P7014. You probably have that memorized.
Now, I will say, one of the things that I thought was interesting about this is in the description of some of the work - and I don't remember if it was here or at the level of the overarching committee - there's verbiage that talks about providing guidance as we develop partnerships with AI. I thought this was interesting because that very language seems to presume an agency that doesn't exist, at least today, and that we really would not prescribe to any other technology, no matter how advanced.
So I do wonder, is the language problematic in ensuring or promoting appropriate user expectations and usage?
BEN BLAND: I think all the language is problematic here.
I mean, I've had some quite challenging conversations about even the title of the standard 7014. Which we can proudly say after five years of deliberation and design with a global community we published just a few weeks ago. I's now available and available for free download as well. If you search for IEEE 7014 you should be able to find it.
Then, this new recommended practice that we've developed, which is kind of an offshoot there with 7014.1 looks specifically at this kind of human AI partnership element. This emerging phenomenon and emerging set of systems in which we do have these quite complex interactions with highly intelligent - I keep hesitating to use the word intelligent just because it's such a hot, challenging word in itself.
Again, empathy, intelligence, these are very problematic words. But we have to find a way to describe the thing that we're talking about. At the same time, this has been a huge problem for us in trying to write standards. Especially in a world where standards tend to be quite specific and technical in the past. A specific process, a specific technical requirement as opposed to law which still needs to be very understandable and, in some cases, concrete, but is still, to some extent, interpretable.
We've been trying to find that sweet spot between them in an area that is poorly understood, is changing way faster than we can write the documents. I mean, it took us five years to write ours. That's a long time. It's like a couple of generations in digital technology, right?
So yeah, it is problematic. My best answer sometimes is to say that when people challenge these terms like empathy and empathic and AI and say, hey, careful, machines can't empathize. We say this is precisely the point we're trying to get across. Look at the standard. We explicitly don't claim that is the case.
But we also try not to be too prescriptive. We try instead to set rules around which people who develop or manage or deploy. Basically, people who have control to some extent over these systems have to be very open about what they are intended to be used for, what they're likely to be capable of, why they're doing this, why they made the choices they did, why they picked the particular technology, and so on. And it partly covers that issue of we don't have a language for this yet. We're still discovering it. And we're learning stuff in our relationship with machines that could be good or bad, but that could be enlightening. It could enlighten us as a species.
KIMBERLY NEVALA: And did you find the process of just trying to develop and work through that standard educational in and of itself? Wre there specific points of contention or maybe just questions of - I always seem to come down on the negative there - but positive questions that got raised that were somewhat unexpected to you or that caught you off guard?
BEN BLAND: Yeah, loads. I mean, I suppose I'm guilty of coming from a mostly commercial background. I'm not really a typical business person. But I have been working in industry and had no real contact with standards, very little contact with ethical and community organizations and things like that less so.
And immediately, you say something that you think is relatively easy to lock down and then there are challenges to it. It's been enlightening to have a different - people from different sectors. We've got a lot of people from academia and that covers a range of subjects from psychology to neuroscience to legal and whatnot. You've got the actual practicing legal and policy people. You've got people from civil society organizations as well as industry people like engineers and so on often coming at the same problems from different angles. But that, of course, is what we try to harness.
The danger, of course, is that you can end up watering things down. Like, for instance, making this set of rules or recommendations too broad, too vague. But also, you can then tighten things up too much. You can make them too complicated, impossible to follow, and so on.
But yeah, we had questions around, for instance, we've been writing an ethical standard. And my understanding of moral philosophy is pretty poor in the first place. But it was one thing to try to learn what the basic ethical frameworks and approaches are and get up to speed with that. But it was then to realize that there's no real-- this is not a solved problem. We don't have one ethical framework that everybody in the world is OK with and we can just apply that to technology. And I think anyone who does come from a very specific strict camp is probably being quite narrow-minded because there are many different ways of viewing things.
So again, we were immediately stuck in this problem of, right, we can't define these things. We can't be strict about which way to approach it. How the hell do we get around this? And we can talk about how we've approached it. But yeah, there are lots of really big thorny questions from day one.
KIMBERLY NEVALA: So we'll just extend on that. What was the approach you took? We’ll link to this as II highly encourage people to spend some quality time with the standard itself. So what was the approach you took and how would you like to see people using it and taking it on board?
BEN BLAND: Well firstly, I'll say, yeah, please do have a look and provide critical feedback. Because as a product guy, I see this as version 1. I mean, really, it should be version naught point something. It should be a beta product, but that's just not really the way standards are developed. And that's something that many of us think needs to change. We need to adapt.
It's the same with law as well. We need to adapt the way that we generate things like standards, guidance, regulation that is suited better to a world in which companies, for instance, are completely globalized in ways that our regulations our standards aren't, or at least our regulations, and can move much faster and so on. So yeah, please give feedback and tell us and we will work on it on version 2 down the line.
But broadly the approach, as I say, we struggled to or we realized we couldn't really define what is the right and wrong way to think about machine systems, the right ethical approach. So instead what we've done is advocated for quite a detailed approach to transparency and explainability that goes right through the system life cycle. And not just transparency into the function of the system, which is probably quite a familiar version of explainability and transparency to people. But also into the ethical choices behind it. Like why you've taken this approach. Why you think this particular user is appropriate to a system. Why not restrict it to a certain subset of people? Is it OK based on the system you've designed to release it publicly or should you actually restrict it and so on?
So the idea is to try to put the developer in a system - I'm sorry, I say developer, I mean anyone who's using this system and deploying it - is to put them in a position where they have to learn about the background issues. They have to explain why they're doing what they're doing. And we can hope then that whether they - regardless of what their initial intention is - the system can then be put out in the world. And then those people who are in a position to do so can judge based on these claims and we can start to develop this kind of auditability and accountability in face of this ecosystem that hasn't really developed yet.
We can say well, yes, you made these claims of x. It turned out that y happened, but we can see that was an unreasonable thing and it could never have been predicted. So culpability might be lower. Or the opposite, of course, you could have seen this coming. You made all these claims. These are not true. You didn't do your homework. And that's the broad approach that we've taken to summarize a whole load of specific recommendations.
KIMBERLY NEVALA: Yeah. But it does sound like this idea of continuous assessment, continuous critical evaluation. So it's not just we may say to you, yes, this was reasonably unintentionally or reasonably unpredictable. But now that you have the information, you should be able to go back and then react accordingly. Of course, there's a lot of commercial logic here that says and certainly, a lot of forces that would say get it out there as then it's very hard to take something back later. But hopefully, we're getting - it's not just in this area, but across the board - we'll start to get into a place where that is not only appropriate and recommended but rewarded. We are nowhere near that yet today.
So this is just, it's a fascinating, rapidly evolving, as you said, complicated area. And we can't even begin to - we've just hopped on the top of every one of these topics. It's like going from lily pad to lily pad here.
So are there any key questions or elements that folks like myself just aren't asking or that we haven't touched on that you think is really important to highlight for the audience?
BEN BLAND: Well, if any of this sounds new to people, then that's something they should go and try to educate themselves on. This idea of empathy in AI, we talked earlier about, do we even call it empathic or empathetic? There are problems with the names. We don't really have a name for this yet. There's emotion scientists and machine learning people might call it affective computing. Others might call it emotion AI or there's emotion recognition systems. And there's all this sort of stuff overlapping each other. So it's quite hard to even educate yourself on these things.
But I would like people to be able to walk away with this kind of thing in the back of their mind. That when they're interacting with a suitably capable machine system to challenge any effort it seems to be making to guess how they're feeling or simulate emotion or thought or anything like that. Just in the same way as we need to learn to recognize the dumber elements of what we're currently calling AI. Where actually sometimes it's just predicting the next word. I mean, clearly, the result of that can be quite profound. But we need to be able to educate ourselves in some appropriate way to those issues.
And also to watch out for this implicit empathy that I've talked about before, this idea that it may not be an overt thing. You might have a smartwatch you bought and it says, I'm going to predict your mood throughout the course of the day. And even then, it's very easy without the right level of understanding to think that it actually knows what it's talking about or that it matters. You get angry on Thursday afternoons. That's not necessarily a bad thing. So yeah, there's a certain level of awareness, I think, I would like people to go into this with as we wait and see what the actual applications will be.
KIMBERLY NEVALA: And again, that individual response is so different. I was on stage once with my Garmin watch and it beeped at me and it told me I was detraining. And you know what? It tells me I'm detraining quite often. And it may not even be wrong, but it's not particularly helpful. I don't find it inspiring.
A lot of times my response is, how is that helpful? So it is interesting: when is it helpful, when is it not?
In any case, zooming back out, what is it that most worries you and then what most excites you as we look at the near to medium future here?
BEN BLAND: Well, I'm always in danger of being a bit of a techno utopian, I think, in that I'm fascinated by what our technology can do. I think that we are overwhelmed with negative stories in the media and whatnot, and it's quite hard. You actually have to go looking for the positive stuff, which is mad.
While innovation in general, if we just broaden it out a bit, may not be the cure for all of our ailments. But I do think that we have so far been on a pretty positive path in most ways and are entering a new age of human machine existence. But I think that there are all the usual problems underlying that around global social cohesiveness. We're still arguing with each other over which groups we belong to and stuff like that.
There is still immense power in commercially-driven behavior in companies and whatnot. Whereby the objective function of these systems, in the end, is mostly going to be about making money or getting people to vote in a particular way. As I say, whether it's deliberately designed or not, that's just where it's at.
All those things worry me whenever they're applied to any powerful technology. And I think this is just that. It's just another potentially powerful technology. But where we want to be really aware and keep our eyes open is in this subtle, subconscious element of interacting with us in around mood and emotion where we really still don't agree on what that is, how it works.
And we can relate empathy. Empathy is a very broad term. I think it's a superpower in a way. But we can relate it to things like charisma and persuasion. Emotion scientists I work closely with over here who talks about how jazz performers and standup comedians are highly empathic. And I think it's just a case that most people don't necessarily think of. But it helps to analogize this for the machine system where a good jazz performer can read the room and then respond in some way that then draws the room towards them. And we find that very attractive. We find it very entertaining. We also will listen to people who are able to do that, to pick up on our subtle signals and then demonstrate that I'm able to read your mind here. I know what I'm doing. And it's a very powerful thing. And unfortunately, giving that power to machines will be OK only if we're aware of what they're doing and we're also acutely aware of what their objective function is.
KIMBERLY NEVALA: Yeah, absolutely. This has been a fascinating toe dip into the very large and evolving pond or ecosystem of emotive AI, empathic, or empathetic applications. We'll see where we come out on that one in the end. I so appreciate your time. I will look forward to continuing to follow the research and the really important work that you are doing. Thank you again for joining us and sharing all the knowledge, hard fought and otherwise, that you've come by over the last many years.
BEN BLAND: Thank you. It's been emotional.
[LAUGHS]
KIMBERLY NEVALA: Yeah, I won't tell you how I'm feeling. I'll let the system read it.
BEN BLAND: I have to guess. Yeah.
KIMBERLY NEVALA: No, all kidding aside, this has been fantastic. Thank you so much.
BEN BLAND: Thank you.
KIMBERLY NEVALA: And thank you all for joining us as well. To continue learning and hearing from leaders, thinkers, and doers such as Ben, please subscribe to Pondering AI. You can find us wherever you listen to your podcasts or now on YouTube.
Creators and Guests
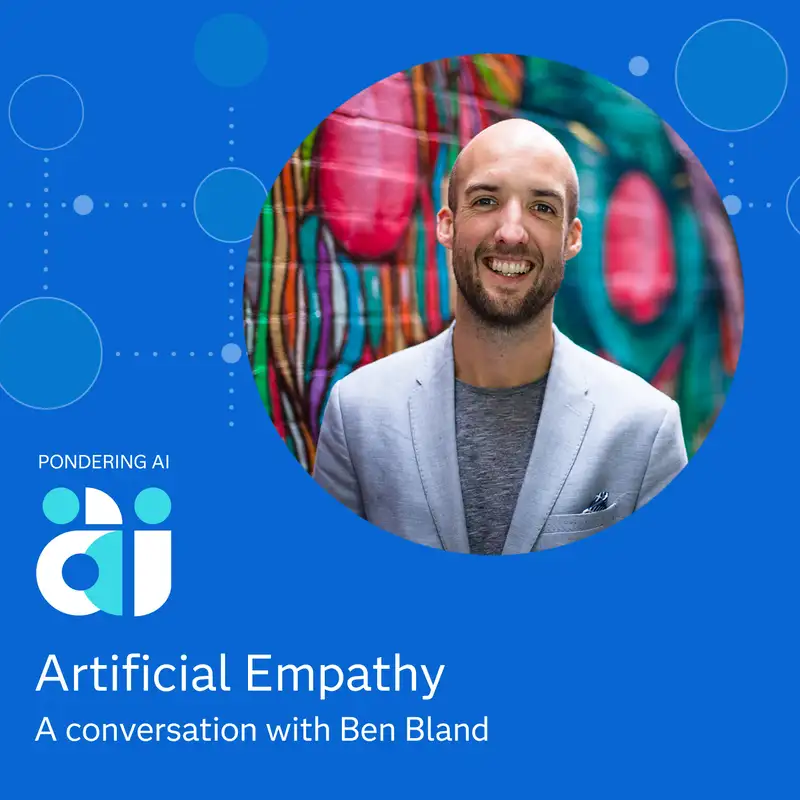