Working with AI with Matthew Scherer
KIMBERLY NEVALA: Welcome to Pondering AI. I'm your host, Kimberly Nevala.
In this episode, I'm so pleased to bring you Matt Scherer. Matt is a senior policy counsel at the Center for Democracy and Technology, also known as CDT. He focuses on data and privacy with a concentration on worker and labor issues.
Matt, we have so many questions. We're going to be talking about where AI should and should not be applied in the workplace, how we can better engage workers themselves in shaping the future of work, and ongoing efforts, including legislation, to safeguard worker wellbeing in the age of AI. So thank you for joining us, Matt.
MATT SCHERER: Great to be here.
KIMBERLY NEVALA: So we're going to start right at the top. Tell us a little bit about your path to CDT and your current role.
MATT SCHERER: Oh, wow. Well, I'll try to make this as short as possible. So I spent the first several years of my legal career in the public sector, working for judges in a prosecutor's office. After I transitioned out of that, I was trying to figure out what I wanted to do next and I decided that I wanted to practice employment law. But at the same time, I started getting interested in artificial intelligence and I started writing and speaking on artificial intelligence, pretty much as a hobby.
Eventually, I got a job offer from a law firm that allowed me to merge those two and work on tech-related issues in the workplace, Littler Mendelson, which is actually the world's largest management-side labor and employment law firm. But after a few years of doing that, I kind of felt the itch to do something more socially beneficial than working for a large law firm that represents large companies. And I had the opportunity to move over to CDT. And I leapt at that opportunity. And I've been there for about 3 and 1/2 years now.
KIMBERLY NEVALA: It's always nice when your hobbies become your career and you enjoy the work.
[LAUGHS]
MATT SCHERER: Does not happen often, yes. It was an unexpected and somewhat serendipitous turn of events that led me here.
KIMBERLY NEVALA: That's awesome. Well, we're glad it did. Now, there is no shortage of concerns and issues about the use of AI in the workplace. But before we dive into those deep and dark waters I'm wondering, are there areas of application that you find particularly interesting and for which AI is well suited within the workplace?
MATT SCHERER: My general philosophy on that is that workers should - there should be a bottom-up approach, essentially, to the adoption of AI in the workplace. AI is going to be most effective when it is adopted by workers who find it useful to their jobs. Rather than something that management adopts in an effort to more efficiently manage the workplace or to try and force workers to take up as part of their jobs if the workers don't have -- are not buying into it.
So examples of that, for office workers, using generative AI or even non-generative AI, things like Grammarly, that's actually a form of artificial intelligence. Grammarly, if you're not familiar with it, is an app that runs on your computer that will check for grammar, style, redundancy, and all these other things while you're writing. It's a form of AI. I use it. I find it helpful. I reject a lot of its suggestions. But that is an example of a bottom-up use of AI.
That's something that helps the individual worker do their job more effectively and gives them control over whether or not to accept the suggestions, analysis, or output of the AI system. To me, that should be the model. That should be the way that companies should think about AI: whether or not they are giving their workers that level of autonomy to figure out how useful it is to them and gives them a voice in how it's used and when it's used.
KIMBERLY NEVALA: Mm-hmm. There's an interesting gray area that can develop, even under that mandate.
We see it a lot, perhaps with more surveillance-oriented or, depending on how you are marketing it, safety-oriented applications, that purport to watch, perhaps, how someone is motoring around the workplace. If they're using safe body or are they applying themselves to the things and the activities at a level and pace that management wants. Very often, those are sold as this is good for you because it helps us improve, make you more safe, improve the quality of your work. Whereas, to the worker, that may seem very paternalistic, if not downright negative. And eventually, be parlayed into places like performance in ways that weren't initially expected.
So what is it that employers and workers need to do to ensure that those interests are, in fact, aligned?
MATT SCHERER: That is a somewhat tough nut to crack.
I think that there definitely is a role for AI in ensuring workplace safety, in certain settings and when done correctly. The example that I think that a lot of folks might be familiar with is, in factories or in warehouses. Those are two settings where the injury rate is a bit higher that the average workplace. It's higher certainly than office work. And those settings are frequently surveilled anyway through traditional methods, like having video cameras in the workplace that monitor the factory floor or warehouse floor.
There are functionalities, AI-powered functionalities, out there that can identify hazards that are popping up on the factory floor or the warehouse floor and can alert either the workers who are near the hazard or supervisors so that the hazard can be cleared. That, to me, is a useful additive application of AI to enhance safety.
But the problem is that, beyond those concrete and relatively narrow examples where the AI is really looking for objects and for hazards that are out in the open, a lot of things that can be couched as, well, we're doing this in order to ensure safety or security, can be taken too far and done in ways that can threaten the privacy and legal rights of workers.
If you include safety, for example, as saying, well, we want to prevent harassment and abuse from happening in the workplace. That could be used as an excuse to use AI to monitor all of the emails and communications. Or maybe you even turn on the microphones on those cameras and you have them record the conversations of every worker. And you run them through an algorithm to determine what workers are saying. If all of that information is made available to the employer, quite frankly, I don't think most advocates or workers have the level of trust that employers are actually only going to use it in order to ensure safety. And more to the point, there's probably less intrusive ways of preventing workplace harassment and discrimination and other security and safety threats than that really intrusive algorithmic form of monitoring.
So, yes, it's a balance. But to me - and a lot of advocates have taken this position - the right way to think about it is, OK, does the employer have a legitimate reason to use the algorithmic system? If so, the use of the algorithmic system should be narrowly tailored to that purpose. They shouldn't be using it to collect information or make decisions on workers beyond the purpose for which that is being done. And that general framework, which is - the technical term, when you're talking about surveillance and data collection - the technical term is data minimization. That is a fundamental principle that a lot of advocates are pushing for.
KIMBERLY NEVALA: There's another aspect to this. I've noticed this even in meetings that I participate in. Is when people feel like they are being watched or there's, today, the ability to record every meeting and have notes generated. Folks are a lot less open and forward with both their concerns or just around open brainstorming and ideating for fear of what may come out. So we've had some success and have started to move towards having sections of the meeting that are always unrecorded, sort of off, quote, unquote, "off the record". We hope they're off the record and we think they're off the record - maybe they are, maybe they're not - to allow that space. So in some cases, where we're trying to quantify and datafy these bits in the interest of automation we may be undermining, actually what's required - the space and the creativity and the incentive - for innovation. It's an interesting conundrum.
MATT SCHERER: It is. And I think that when people hop onto a Zoom meeting and they see that recording "this meeting is being recorded" alert, they probably do act differently than when that alert doesn't come up.
And beyond that, I think that this is actually something that the general counsel for the National Labor Relations Board made a point of a couple of years ago. Workers may be chilled in exercising their legal rights if they know that they're being watched. So that's a major concern for a lot of advocates.
If they know that their communications are being monitored, even if it's supposedly only for purposes of ensuring safety and legal compliance are they going to…is that going to discourage people from trying to form a union? And is that sometimes maybe the underlying objective of employers in adopting these algorithmic monitoring systems? It's interesting that, anecdotally, a lot of employers start to, there have been lots of cases, where advocates have noticed employers adopt those sorts of electronic monitoring systems in relatively close proximity to union-organizing campaigns. And it doesn't seem coincidental.
So there's the unintended, the truly unintended consequences of it chills people's creativity and it chills people's exercise of their rights. But there's also the question of whether, in some circumstances, employers might be doing it for the purpose of chilling some speech or some rights.
KIMBERLY NEVALA: Complicated, important areas.
Now, AI or no AI, I think we can safely argue that any process or application that has to do with whether someone gets considered, hired, fired, or how they're measured in their job is, almost by definition, high stakes. At least for the individual always. So when it comes to AI in particular, are there particular popular or emerging areas of application or types of applications where you have just seen that the expectation or the presumption of the machine's capabilities just flat out exceeds the reality of the situation?
MATT SCHERER: Oh, many, many, many.
The reality is, AI is generally no better and is often worse than humans at decision making in employment. And there's a reason for that, which is that, as a society, we have not gotten very good at measuring what makes a good employee. And without objective, quantifiable metrics that define what a good employee is in a given job, you can't automate the process of looking for good workers in that job.
An example that I often use as a comparator is sports. If you look at my favorite sport, basketball, there are drafts every single year for players in the NBA and the WNBA. Those players are being drafted to do something that they've been doing for many years, where they have been watched and quantified, basically performing the exact same job in a new setting that they have been performing for many years in other settings, admittedly less challenging settings.
Despite the fact that you have those long track records and that they're also brought in for private interviews and workouts and combines, where they are poked and prodded and their physical abilities are measured down to the n-th degree, there are still more misses than hits in the drafts for the NBA and the WNBA. Usually, teams do not select the best player available, the person who will go on to have the best career, who would have been the best fit for them, in the draft. And that is in a setting where, again, the similarity of what people have done before to what they're doing now is about as high as it can be. And the amount of objective metrics that are in place are about as many as can be.
But the reason is that there are so many things that you can't quantify that matter in basketball. There are so many things that have to do with the interaction of how a player plays with their team, with their motivation to continue improving over time, with all of these other things that you can't measure in a training camp or you can't observe from their past playing against lower-quality players. If that's true in basketball, it's true to a hugely greater degree in the vast majority of other jobs.
In the vast majority of jobs, you can't even explain the things that make somebody a good employee in a good position. Certainly, you can't predict how they are going to perform alongside new colleagues that they've never worked with before. And if those colleagues themselves change over time and that changes their performance over time, you're certainly not going to be able to measure and predict that.
So, just fundamentally, there is a challenge in trying to automate any sort of assessment or hiring process. Until we figure out how to better measure employees in most jobs, it's just not going-- all you're going to be doing is quantifying and automating a flawed process of employee selection to begin with.
And the risk with that is that, with AI…With a human who is selecting individual people for a job, there's only so many resumes they can go through. There's only so many interviews they conduct. If you have an AI system… and if that person is bad at their job, I should say, if that HR recruiter is bad at their job or they're biased, there's, therefore, a limited amount of damage that they can do. AI systems can screen out as many people as you feed into it in as short a period of time as you can imagine. So the scale and the potential for harm from an AI system that is bad at decision making is much greater than it is for a human that's bad at decision making.
KIMBERLY NEVALA: Well, so as you were speaking before, I was thinking about that old statement that all-star teams aren't composed of all stars. That it's really down to human engagement and performance and the dynamic interaction is really, really messy. And maybe that messiness is just, it's messy enough to defy true quantification and any sort of automated selection.
But all of that being said, there is an inherent assumption that, by virtue of giving these applications that can look at lots and lots of data - a scope of data and data points that a human would not be able to assess - and by virtue of the speed and their ability to look at patterns, they are just somewhat inherently more efficient. That they are going to be maybe more fair, although folks will go back and forth on that.
Are there just basic assumptions and beliefs then in some of the processes here that we have to debunk or more critically analyze before we can decide when and where to apply AI fairly and appropriately?
MATT SCHERER: I certainly think so. One thing is that, by their nature, the vast majority, if not all of the AI systems that I've seen that assess and try to make decisions about workers, they basically apply a one-size-fits-all approach. They have a set of factors that they are trained to look for, as it were, in candidates. And to really dumb it down, they score candidates based on those factors and their presence and absence in the candidate.
So for a resume screener, that means that it's looking maybe for keywords or patterns of keywords. In a video interview analyzer, it's looking for particular phrases that the person speaks. In a personality test, it's looking for particular responses to particular questions.
The issue is that that's a one-size-fits-all approach. And if there are people that are underrepresented in the data that is used to train it, it's not just a matter of it'll be maybe biased against them. It fundamentally won't know what to do with them. It won't have enough information in order to make an accurate assessment of that person's ability and potential. And an AI system just simply does not have the life experience or the common sense of a human recruiter that, when somebody comes up with a truly unique experience it's never seen before and a human recruiter says, wow, that could really actually make - it's something that I wouldn't have thought of - but it could actually make them a good fit for this job or bring us something that we've always lacked, an AI system just has no capability of doing that.
So the issue of bias, right now, given the state of the art of where AI is, in my view, is fundamentally not resolvable. AI can't even make inferences or best guesses as to things that are not in its database that it doesn't know. And the variety of factors that go into, again, any job, the number of potential causal relationships between what could make somebody good or bad at their job, is far beyond what can be captured in data and trained in an AI system. A human might be able to pick up on it. Often, they won't.
Humans are certainly prone to having their own presuppositions and overlooking things that they're not familiar with, or even being scared or rejecting things that they're not familiar with. But at least they have a shot. At least they have this background of common sense and the understanding of cause and effect to try and be able to make inferences about people that have unique characteristics that have not popped up in the past.
KIMBERLY NEVALA: Now, that being said, you previously mentioned, we're not actually that good, even today, even outside of AI, at really performance and 100% positive recruiting. So there is a tendency to go, "what about?" There's a whataboutism, which says, OK, that's fair, Matt, but humans are biased and so the machine's a little biased. But hey, it can still look at more people and maybe we can just sort of adjust it.
I saw this report, actually just yesterday, and it was very optimistic about AI-enabled recruiting. And essentially, the conclusion was an AI recruiting tool will increase recruitment quality, it will increase efficiency, and it will decrease - this was interesting because they said transactional work and I'm not sure what that meant. With a little underscore that says, but algorithmic bias of every flavor - they had every ism in there, ageism, sexism, racism, all of it - can cause discrimination. And the solution proposed was an unbiased data framework and data sets and good governance. What's your reaction to that?
MATT SCHERER: There's no such thing as an unbiased data set. It does not exist.
KIMBERLY NEVALA: [LAUGHS]
MATT SCHERER: I can get into the more technical details of why de-biasing an AI system is essentially impossible. But it requires a bit of explanation. But before we turn to that, I think that the argument that, well, humans are bad at something, therefore, what's the harm in having a more efficient machine do the same thing, is just fundamentally almost-- not almost-- it's ridiculous to me. If we're not good at measuring something and quantifying it, we are not ready to automate it.
Let me give you a story of something that happened. I was giving a talk to a relatively small but prominent group of people who work on AI policy issues. It was a mix of mainly people in academia and industry. And I made the point that we are not allowing self-driving cars out on the road to just go and do their thing without any human supervision right now because, even though they might be good at 98% of driving tasks, they're not 100% of the way there. And that last 2% can lead to a lot of problems.
And as I was saying that a lobbyist for a big HR tech vendor cut me off and said, well, you're just fear mongering right now. People die when a self-driving car malfunctions. Nobody's dying as a result of an AI system that is doing HR tasks. And I said, no, people are just losing their jobs and missing out on career opportunities. No big deal, right?
That kind of attitude of well, either humans are bad therefore, we can just automate it and let the machines do all the work that humans are doing right now. Or that just because people aren't dying or because losing a job is not as big a thing as some of the other more catastrophic and life-threatening and society-threatening and matrix reality threatening forms of AI harm that get talked about, doesn't mean that it doesn't matter. When somebody loses out on a job opportunity, that is a very big deal to that person. When somebody gets fired based on a flawed analysis of an AI system, that is a very big deal to that person. So I think that you have to be very careful about automating that process.
And unfortunately, no matter how many times vendors say, oh, well, our systems don't make automated decisions in public and that they're supposed to be used under human supervision. I've been in so many pitches and I've been at so many conferences where they say the exact opposite. When vendors are selling these tools to companies, they are telling them, you can have fewer staff, you can delegate decisions to these machines. Yeah, we say that in public, but we have to say that in public. In reality, here's a cost-cutting measure that you can adopt that will automate many aspects of this process.
So to me, I think that a lot of the well it improves efficiency and because humans are flawed decision makers anyway, you might as well go with that efficiency, again, it fundamentally, number one, ignores the impact of bad automated decision making. It ignores what I said before: that an AI system that is flawed can cause a lot more harm than a flawed human decision maker can. And just fundamentally, again, until we do a better job of defining what it is that makes a good employee in a specific job, you're not ready to automate looking for candidates for that job.
KIMBERLY NEVALA: And hopefully, the tendency there won't be to start breaking down jobs and tasks into minute, quantifiable bits. How many things you pick up and put down and how many times you tap the keys. That seems to be a bit of a trend.
MATT SCHERER: Exactly, it is. And unfortunately… A lot of people make the point of, so are we ever going to get to that point in most jobs? Frankly, the answer is probably not. And that should be OK. It should be OK that we're never going to get to the point that we're going to be able to automate looking for people in most jobs.
We have been able to automate for a long time, more or less, the process of screening out people for certain jobs. A classic example, which is ironically becoming dated because of the rise of AI, is transcription services. They're the only things that really matter are how accurate and quickly you can transcribe what somebody is saying. So a typist whose job it is to do that, you can essentially automate the process of looking for them by having them take a typing test that measures their speed and accuracy.
With the exception of that and maybe some sort of background check to make sure that they're not an ax murderer, you can automate the process of looking for that. But there aren't that many jobs like that. There aren't that many jobs where the things that matter are so few and so discrete that you're going to be able to measure them.
Back when I was a prosecutor, there was only one metric, really, that they tracked in order to evaluate you. And that was the number of trials that you won, where you got a conviction. That is - and essentially, so I should say there's two - there's how many trials you won and there's how many trials you lost. Your winning percentage, essentially. And that is a horrible way of evaluating prosecutors.
It's a horrible way of evaluating any lawyer who goes to trial because it's going to punish the people who take on the hardest and most complex cases. Those people are going to have fewer trials that they go to because they're taking on the harder cases and they're going to lose those trials more often. But that's not because they're worse lawyers. It's because they are doing a harder job.
So maybe in 150 years, the job of being a prosecutor will be so well defined that you are able to quantify the things that matter and measure them. My guess is that you never will. And I think that that should be OK. I think that we should be OK with the idea that we are never going to be able to quantify a job to the degree that is necessary to make automation of looking for good people for that job, and automation of the task of firing people who are bad at it, something that we should feel comfortable doing.
KIMBERLY NEVALA: Yeah, there's an - I don't know if it's an adage or a principle - when we talk about decision intelligence in general. Which is that we should really be evaluating the quality of the decision-making process and not measuring just the outcomes of those processes. Because how you got to the decision, there's just a lot of factors outside of your control in almost any sort of decision (outcome). Whether it's just luck or circumstance or we are just fabulously, fabulously messy creatures, all of us. That can work against you. But those that have a good process or method for how they make decisions is where you want to go. So that's an interesting bit.
I've also been thinking, specifically in the context of the workplace, about that old apologue about the frog and the frying pan. Where, as the story goes, you throw a frog into a hot frying pan he jumps right out. You put a frog in a cold frying pan, heat it up, and he just becomes acclimated to it incrementally over time. And as the fable goes, he'll just sit there while he burns. Apologue aside, I'm wondering, when you look at where we are automating or starting to apply these types of systems - AI and perhaps even other in the workplace are there places where we're starting to set precedents that might go unobserved? Or seem very non-germane, but that ultimately build into something harmful because maybe they erode expectations for how one should be treated or analyzed or what have you?
MATT SCHERER: That's a great question. I think surveillance is probably the best example of that and automated forms of data collection and surveillance of workers. There is a growing tendency of employers to, well, number one, employers have been, in a lot of cases, collecting a lot of information about their workers just because they can, for years. There are really no guardrails that are in place right now legally that prevent. That say that lawyers or, sorry, that say that employers cannot collect information or engage in surveillance of workers while they are on the job. And really while they're off the job too, as long as they agree to it.
The result is that, for a long time, employers have not been very restrained in monitoring workers' computer activity, in setting up cameras in the workplace. But now that AI is coming along, there is a lot more detailed and privacy-threatening information that can be collected and analyzed through those data collection and surveillance methods. And what might initially start as a limited, oh, we're just doing this to monitor health and safety. Or the classic example that people are familiar with is when you call into a customer service line it says this call may be monitored or recorded for quality assurance and training purposes.
Well, initially, those calls were just recorded and potentially played back later by a supervisor to listen and see if there are teachable moments or if there's a complaint to hear what happened on the call. But increasingly, what is behind that warning is not just that sort of passive recording that may be actively listened to later. But active AI systems that monitor in real time what the customer, what you, are saying and what the customer service representative is saying. And providing feedback or alerts to the customer service representative while they are talking. There are AI systems that have a - that is their function - that they give that real-time feedback to workers. And by feedback, I mean it can say things like, you're not showing enough empathy, show more empathy to this caller. And it's very creepy.
So I think that there is that tendency. You can have surveillance that is theoretically in place for this limited purpose, like "training” quote, unquote, but the use cases of it kind of creep in over time where it becomes more and more all-encompassing. And it is more and more eroding of the worker's privacy and dignity.
So to me, that is the prime example is surveillance and data collection. You might say, or even the employer might fully intend to use it, for a limited purpose at the beginning. But then when a vendor comes along and says, hey, I have the ability to sell you this tool that will allow you to do a lot more analysis and make a lot more decisions using data that you already have access to or you're already collecting, the ability for those uses to creep into the creepier territory proliferate quickly.
KIMBERLY NEVALA: Well, as a consumer or a customer, I don't like those either. And in fact, again, this is that interesting point where it may work again against an agent who follows something that says, she's pissed off, be empathetic. Nothing irritates me more.
MATT SCHERER: [LAUGHS]
KIMBERLY NEVALA: I don't want you to feel bad. We don't need to empathize. I just want you to tell me what to do to fix this. And I've been known to call them and say I'm sorry if I use my mad voice. I'm not intending to. I know it's not you, but if I call, I'm already mad, right? You don't need a system to tell you that. So it is very interesting.
But this is probably a nice segue then into the evolving - I'm going to call it a patchwork - landscape of regulation and guidelines that are coming about. Now, when I was doing some of the research and looking into some of your work previously, I was really interested to note that there was a privacy bill in California - I think it was one of the earliest ones - that you had specifically noted was one of the first to not carve out workers or to exempt workers from its protections. Which then fundamentally just begs the question: why have workers - workers in particular, by and large - been taken out of the scope of what are already fairly limited data and privacy protections here in the US or otherwise?
MATT SCHERER: Yeah, so California's privacy law is actually still the only law in the country - the only data privacy law in the country - that doesn't carve out workers. That covers workers and provides them with some level of data privacy rights. I think that the reason that that happened in California but it hasn't happened elsewhere is the way that the California Privacy Rights Act, which is basically the version of the law that is now in effect there, came into being. That is, it came into being through a ballot initiative. As a result, you didn't have the same ability of, frankly, lobbyists to come in and shout down the efforts to ensure that employees get covered by it.
Now, that being said, even in the California Privacy Rights Act, it's really a consumer-focused bill. And if you want to talk about the real fundamental reason that workers are often left out of this, it's number one that we start from - when we're talking about data privacy - we start usually from let's write a bill that protects consumers' privacy. And workers are an afterthought, if anything. And that certainly was the case in California's privacy law.
The reason that I think that data privacy laws in other states continue to carve out employees is that those haven't been adopted through ballot initiatives. They've been adopted through legislation. And legislators face immense pushback from business and industry groups that do not want to see any regulation of their ability to collect information and use automated systems to track workers.
There is just a culture, a policy culture, if you want to talk about it - and in the United States not just a policy culture, but a societal culture - where we have come to expect that once a worker steps foot in the workplace and is on the clock that the employer has an exceptionally high degree of discretion to watch them, to monitor them, and to manage them. And that there's really, unless you are actually committing a crime, there's almost no guardrails on the management's prerogative to collect information and take steps that outside the workplace would seem like a really gross violation of someone's privacy.
Until that narrative starts to change, and I think that we're starting to see that enough people are creeped out by the different things that AI can do to spy on you, that maybe we're starting to see a little bit of a shift in that. But until that really starts to change substantially, I think there's going to continue to be much stronger pushback from businesses against adopting rules that protect workers' privacy, protect workers' data rights. And there's going to then, on the other hand, there's going to be a push from workers and their advocates to put those protections in place. Right now, workers are not nearly as activated to get those protections as employers are to stop them. I think that that's the fundamental problem.
KIMBERLY NEVALA: We'll see if that changes here. So there are a couple other bills. I think it's in Colorado - SB20 something or other - that related to or addressed some of these types of issues. It was interesting. You had an analysis published through CDT addressing there was a lot of criticisms of that particular bill. That it was perhaps overreached, overreaching, or just unmanageable. And your take was really the exact opposite, that, in fact, not only did it not overreach, it, in fact, had some weak points that needed to be further shored up.
When you look at a bill like this or the very optimistically titled, I like this just as a tagline, the No Robot Bosses Act or something like that in New York. What are some of the maybe common misperceptions around these types of protections and regulations that either hold them back or are used to keep them from becoming real, substantive pieces of regulation and legislation?
MATT SCHERER: Yeah, I don't know the degree to which I'd call it a misconception.
Right now, there is just so much hype surrounding AI and every state wants a piece of what seems like a continually growing pie of startups and businesses that are using AI, that they are just terrified of adopting any legislation that the tech industry or business lobbyists come up and say, this will hinder innovation, this will slow it down. And I think that there's two answers to that.
Number one, we should not view innovation as a good thing in and of itself. Innovation is a good thing when it benefits workers and consumers. It is not a good thing when it exploits them and increases power gaps between businesses and workers and consumers. I often say, the Ford Pinto was one of the more innovative cars that has ever been invented in American history.
KIMBERLY NEVALA: Fighting words, Matt. [LAUGHS]
MATT SCHERER: Yes. There's also this inventor, who was in the news relatively recently, who railed against any efforts. Who basically ignored the regulations on his industry, railed against the efforts to rein in what he viewed as a very innovative method of doing things in his industry. And it led to disaster because those regulations, as it turned out, were in place for a reason.
I'm thinking of the guy who invented and ran the business that did the Titanic submersible. And if you read his emails beforehand you frankly see a lot of the same arguments that tech lobbyists use. Which is like, regulations just hinder innovation. People are wetting their beds over the potential for harm with these technologies, but what we should really be doing is focusing on making sure that innovative practices are not stifled. Again, yeah, people aren't going to die as a result of having bad AI systems. But they are going to lose out on job opportunities and they're going to get fired from their jobs.
So I think that misconception or problem number one is just that, simply because something might slow down innovation of some sort does not mean that it's a bad bill. What you should be thinking about is, is this bill going to stifle good innovation or is it only going to stifle innovation that is harmful to workers and consumers? If it's only going to stifle innovation that is harmful to workers and consumers, to me, that's not an argument against the bill. And in my view, none of the legislation that has been proposed anywhere in the country would come anywhere close to stifling good innovation. ‘
The information, for example, in the Colorado bill, that it would require companies to provide to consumers is information that the developers and deployers of AI systems already have in their possession, routinely communicate to each other. And all we're asking for, as advocates, for the advocates who want to see those sorts of protections in place, all we want to do is see that information also provided to the consumers and workers who are the subjects of those decisions.
If you read, for example, the impact assessment provision of the Colorado bill, all that does is require companies to document, for the most part, things that are already, again, in their possession and that they already are doing it. It's just asking them to write down this is what we do to test for relevance and validity. And it does require, the one kind of affirmative obligation that it puts on companies, is to check and make sure that it's not discriminating against people. Which is something that federal law already requires them to do anyway in most circumstances.
So again, to me, the question, there's two answers to that. One is, the question isn't whether it stifles innovation, it's whether it stifles good innovation. And to me, the answer is no. And two, is it asking companies to do anything that is actually a big lift if they're doing what they're legally already supposed to be doing? And the answer, again, is no on that.
So those would be my answers to those things. And as you said, in my view, actually much more is needed to protect workers and consumers from AI. I think we're in the midst of a massive AI hype cycle, where the abilities of these systems are being dramatically overplayed and oversold. And once people, whenever you actually explain to a person how these AI systems work, they come away much less impressed than they were after the vendor pitches, the developer pitches about the hype around these systems. So I think that there needs to be a more sober assessment of, OK, what is the real, good innovation that these systems are actually bringing to the table? And the answer is, very frequently, a lot less than people think there is.
KIMBERLY NEVALA: Mm-hmm. Now, you alluded, in talking about notice, to this concept of transparency, which is a big pillar in a lot of emerging AI-specific regulation. Whether that's the EU AI Act or some of the again various patchwork of things that are across the US or even mentioned in the executive order. And transparency can cut both ways in that telling somebody that you're using a system is not necessarily the same as providing meaningful information or consent or allowing them to take action in a meaningful way. What, in your mind, is required for organizations to be meaningfully transparent about systems being applied in the workplace?
MATT SCHERER: The formulation that I usually use is, number one, you can distinguish between the collection of information by automated systems and the use of that information to make decisions.
When you're talking about the collection of information, the main thing is you need to…my preference would be to have the model that they have in Europe. Where you can collect the information but there has to be meaningful consent. And in general, what they say in Europe is that meaningful voluntary consent is pretty much impossible to get in the workplace unless you're talking about a union member. Because of the power imbalance between employers and workers. And I think that that's right.
So I think that, for automated data collection, there should be hard guardrails like you can't collect information that reveals people's disability, that monitors them for their potential to join a union. You should place hard limits on these particular uses of data collection. And beyond that, again, what I said earlier, there's that principle of data minimization. You should have a legitimate reason for doing so and your data collection should be limited to what's necessary to do that. That's the information collection side.
On the decision-making side, I think that actually notice and consent goes a lot further. It provides a lot more protection than it does in the context of data collection. The reason being that we already have a lot of laws in place that protect people from discriminatory decisions. And really, what is missing to me is that most people don't know when AI systems are even being used to make decisions about them.
So in that setting, transparency - whereas, when you're talking about data collection and surveillance, transparency alone I don't think goes far enough to provide meaningful protection - in the AI context, it can allow existing laws against discrimination, existing laws against unfair trade practices, to do their job, essentially. In order for them to do their job, I think that what's needed in that context is workers need to be told that an AI system is being used to make a decision about them, that this is the decision that it is being used to make, this is the information that it's using to make that decision, this is how it uses that information in order to make that decision, and this is the output and the role that it plays in the decision-making process.
That, to me, is the core of what needs to be revealed. And it needs to be backed, importantly, by strong enough enforcement mechanisms that companies feel that there's a greater risk in ignoring the law and withholding the information than there is in providing it and potentially subjecting themselves to a discrimination or unfair trade practices lawsuit. So to me, that level of transparency, it's essential in order for even the laws that theoretically protect workers and consumers from unfair trade and discriminatory practices from working correctly. You need that transparency in order for those laws to work correctly.
And then, if we decide after that that even greater protection is needed of some kind, maybe we've gotten to the point as a society where it's not enough that we simply want to stop discriminatory decisions. Maybe we want to make sure that decisions are actually accurate and not arbitrary, which is actually not a legal right right now. Right now, if a company wants to, for example, adopt a system where the way that they choose who gets hired is to simply pull people's names out of a hat, that's perfectly legal. Maybe we would get to a point in our society where we want that not to be legal, where we want companies to actually use a fair and accurate method of selecting employees. But to me, it's not necessarily like … that should be a right regardless of whether you're using AI. [LAUGHS]
So again, from my perspective, I think transparency goes a long way in providing people the protections that we as a society have decided that workers and consumers ordinarily should get.
KIMBERLY NEVALA: Yeah, that's great. Now, I know we're coming up on time here too.
You surfaced an article that was really interesting recently by some work by Dr. Wilneida Negrón. I'm hoping I'm pronouncing her name correctly. She really makes a cogent case that, when it comes to workers and expressing the rights and the needs of workers, that we can't rely on experts. You have, I think, in a separate but related context - but you can correct me if I'm wrong there - also said that given the pace of the technology, given the scale of the change, given the strength of the tech and industry cohorts, that we may need to think a little bit differently about how we can best regulate and approach this on an ongoing basis. Specifically talking about this idea of co-enforcement. Can you give us just a quick snapshot of what that is and why that might be important moving forward?
MATT SCHERER: Yeah. I think that the fundamental issue is that we, for better and for worse, live in a society where there's a capitalist system. And in a capitalist system, the people who have more resources and businesses who have more resources are going to be at an advantage in both policy arguments and in labor market dynamics. So that means that, enforcement, there's always going to be pushback from companies against strong enforcement mechanisms.
And there is consistently an issue that, without a private right of action, which is the usual way… So a private right of action is when an individual worker or consumer has the right to go and individually file a lawsuit against a company that has harmed them in some way. That is the classic bottom-up leveling the playing field way of ensuring that people can vindicate their rights. If you don't have that, then you're relying on government agencies to enforce laws. And they typically don't have the resources and are often purposefully starved of the resources that are necessary for them to do their jobs effectively.
So in order to level the playing field, there's this emerging idea of what's called co-enforcement. Where we don't rely just on agencies and the experts that staff them to enforce laws. Those agencies work with, basically they use, individual workers and consumers and their advocates as their eyes and ears on the ground to look for companies that are potentially violating the law and bringing them to the agency's attention. In order for that to work correctly, though, it has to be very purposeful. And right now, those ideas of co-enforcement are still very much, I don't want to say in their infancy, but they are not consistently applied.
KIMBERLY NEVALA: It's still nascent, so more to watch there. So final question. What is it that you're keeping a close eye on and interested to see what evolves over the next six to 12 months in this space?
MATT SCHERER: Yeah, kind of uniquely, I'm probably not looking at the presidential election on that front.
KIMBERLY NEVALA: [LAUGHS] What? Good for you.
MATT SCHERER: Yeah. At least in terms of my job, I think that the main action on these things is going to be in the states. We saw this past year different versions of this industry-backed bill on AI systems proposed in, I think, 11 states. And additional AI decision-making bills passed in several more beyond those. I don't expect that pace to slow down in the next year.
I think that what is going to change is that, unlike this past year, where virtually all of the AI-related bills that were introduced in the states came from industry groups, I think that that's going to not be the case going forward. I think that worker and consumer advocates are activated and are looking to be more proactive in policy on this stuff in the next year or two.
KIMBERLY NEVALA: Awesome. Well, we will definitely track that with you and perhaps get you back early next year to let us know if that came true. Just fantastic insights, I appreciate your time and providing us a bit of a look behind the curtain, if you will, of AI in the workplace. Certainly more to come in what seems to be a dynamically evolving space, so thanks again.
MATT SCHERER: Thank you for having me.
[MUSIC PLAYING]
KIMBERLY NEVALA: Thank you all for joining us as well. If you want to continue learning from thinkers and advocates and doers like Matt, subscribe to Pondering AI now. You can find us on your favorite podcatcher or on YouTube.
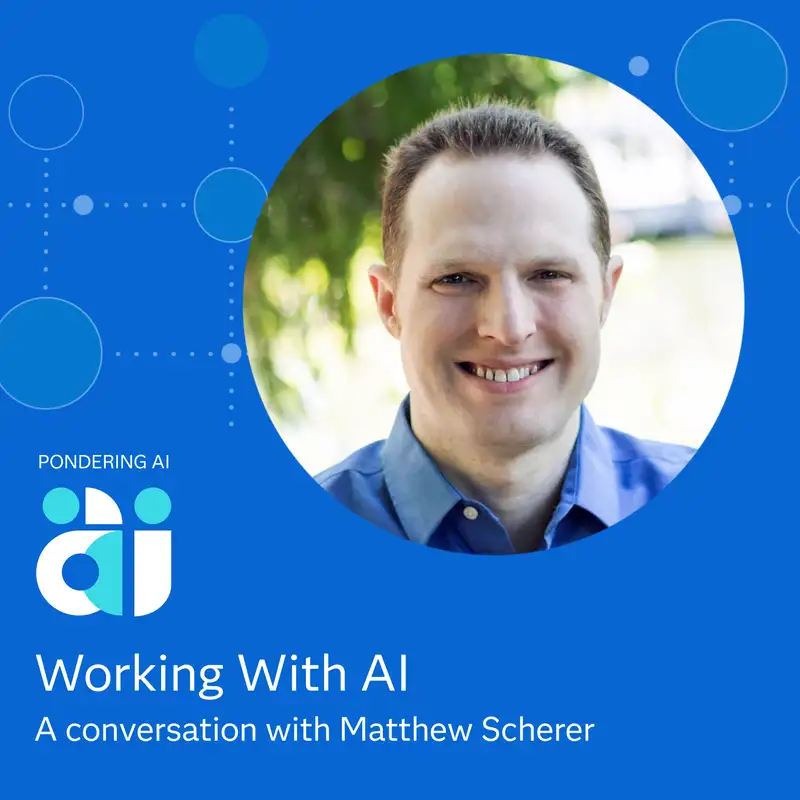