Chief Data Concerns with Heidi Lanford
KIMBERLY NEVALA: Welcome to Pondering AI. I'm your host, Kimberly Nevala. In this episode, I'm so pleased to be joined by Heidi Lanford. Heidi is a seasoned analytics practitioner and executive who has been named by CDO Magazine as one of the top global data power women for three years running.
Heidi has led enterprise and worldwide analytics efforts at companies as diverse as Red Hat and Fitch Group. She is also an avid entrepreneur who runs a successful data and analytics consultancy and has recently co-founded two new AI startups focused on AI readiness and culture. And, more specifically, what is required for senior leadership in the age of AI.
Amongst other things, we'll be talking about how AI has put the spotlight back on data and whether that spotlight is driving the required investments in data infrastructure, from culture to technology. Welcome to the show, Heidi.
HEIDI LANFORD: Thanks for having me, Kimberly. Really excited to be here.
KIMBERLY NEVALA: Excellent. So let's start by talking about what it was that originally enticed you into the data and analytics space and what keeps you engaged still today?
HEIDI LANFORD: Wow, I, like you mentioned, I've been in this field for a long time, several decades.
Interestingly, it's the career path that I chose when I graduated from university. I really liked math and I liked statistics a lot. I happened to land into a consulting group with PwC that was focused on analytics. And I was doing projects for Fortune 500 companies that were really invested in, I'd say, more of the technology and infrastructure, such as like ERP systems and CRM systems.
But our group was used a lot of times on the front end and on the back end to help sell those big projects, multi-million dollar projects. To develop the business case by doing some analytics and finding out some opportunistic areas. Then, on the back end, to ensure that they were going to achieve the ROI that they needed.
It's interesting because, as I think back on it, the need for this skill set and this type of work, extracting value out of data, it's advanced a lot in the technology. But we're also still seeing the same kinds of struggles in terms of adoption, momentum, areas of focus that we saw 20 or 30 years ago. It's still hard to do.
KIMBERLY NEVALA: Yeah. That's very interesting because, on the one hand, AI and the discussions of AI, seem to be shining the light again on the importance of data. On the other hand, there are certainly numerous anecdotes that I've heard, numerous headlines that bemoan the precarious sands, if you will, that folks such as Chief Data Officers or CDOs continue to find themselves. And it does seem an odd conundrum. Why, if data is hot again, are CDOs continuing to find themselves then on the perpetual, proverbial hot seat?
HEIDI LANFORD: Well, using data, whether it be in operational practices of a company, using data and the value that data and information bring to make better decisions about how to run a business, or infusing data and AI into products if you develop data-oriented products, it's a bit of change management, new capability.
We're asking people to think about things differently, change business processes, change habits, change the way that they do work. And any time you introduce change, it's a lot about storytelling and changing the hearts and minds and getting people on board. CDOs are not always equipped with those skills. It takes a lot of learning and development because you're figuring out -- you're an expert on the technical side. You're an expert in either the technology that's being used or you're an expert in the data or you're a data scientist. But then, you also have to be incredibly persuasive and sales oriented. And that takes a whole other different set of skills.
I think another dimension to this is the willingness to change and finding where those pockets of forward momentum and likely adoption are going to be. And - maybe this is another topic we could talk a lot about - I hate to say the proverbial, "Don't boil the ocean." Figure out some things to get started, it's really true. It’s not only true to demonstrate progress and forward momentum but it's also just physically impossible to solve all of the data woes that a company may have. To make data perfect and have everything defined and cataloged and seamlessly integrated and removing duplication. And then having everyone operate in this nirvana-type environment where the data is pristine. You're never going to get done with that project. So breaking this down into areas that are going to have the most impact and value and aligning with the folks that you think can carry things forward, that's probably the best bet.
That also takes time. So if you're new in an organization, as a CDO, you've got to figure out who those folks are, what those departments are, and what are the biggest important issues that a company has that you can help make the biggest impact. So I think those are some of the contributing factors. There's another one that's probably a separate topic, and that's aligned incentive structures.
KIMBERLY NEVALA: Now, is it true that, when you come into an organization to lead the analytics and data efforts, one of the first hires you look to is a communications person?
HEIDI LANFORD: It's my go-to first hire. I've written about this a lot. I did this in my past two roles. I hired a comms expert. They weren't necessarily experts in data and analytics communications but they were experts in communicating and articulating value, and crafting messages, and tweaking those messages that resonated with different audiences.
They also had responsibility for helping me to report out on progress. And again, what we, our teams, would think would be great - like how much data do we get into a data lake and how many people were using it, didn't really matter to our stakeholders. So getting my leadership team to pivot and think about, what does this person over on the product management team really care about? Or what does this person in the finance organization or sales or marketing?
It's funny. I recall vivid meetings where me and my communications lead were just kind of pounding on the leaders in my team about no, they're not going to care about that statement. Nope, they're not going to care about that one. Say it to me like you're at a cocktail party and I have no idea what you do. So explain it to me so that I don't want to walk away and go get another drink. Keep me engaged. That was really, really important. You can never underestimate the power of communications and messaging and storytelling.
KIMBERLY NEVALA: You mentioned right up front that the more things change, the more things stay the same in some aspects. Do we still, as both analytics, even AI and data practitioners, overestimate how much senior leadership, decision-makers, even the C-suite, appreciate or understand the amount of foundational work that is required to deliver on these higher-level capabilities that they just want? Everyone wants AI. Everyone wants Gen AI. Are we still somewhat too optimistic about the level of understanding they have.
HEIDI LANFORD: I think so. I will say, one of the things that I did in a previous role. We were building out an executive dashboard so that we had kind of like a one-stop shop for our C-suite to be able to see all of the key leading and lagging indicators for where the business was headed.
It was really important that we got several opportunities to sit down, one on one with our CEO and go through the intricacies of the dashboard. But not only did we just click on filters and look at graphs and things like that. We also talked about some of the things that we had to overcome to prepare that data, to make this dashboard look great. And there was a ‘oh, my gosh moment’ of I didn't realize that there were five different definitions of a customer that you had to overcome. How did you overcome that? And why was that a problem? What was happening to the data when it left the central repository of all of the good data? How did it come have all of these amalgamations and start to look different? To be able to share that, I think it was kind of eye-opening for some of our executives.
Even for people like myself who have been removed for many years from the actual programming aspect. I feel like I can relate to people on my team and how much work it takes. But it's been a while since I've written code and had to integrate data sets together. And it's always helpful to have a refresher. Of getting down into the weeds and understanding a few tidbits about what is actually happening because then I appreciate what's going on. If it's possible, I can help influence ways to make that easier or stop some of the duplication work or figure out, why do people feel a need to change data? It's not meeting their needs so what can we do to help automate that for them? or change the way we're defining things or get new sources of data so that they can feel that they're more effective in their jobs. I
t's important as leaders in this field to be able to dive deep, bring your head back up, and then figure out a path forward, and do that frequently.
KIMBERLY NEVALA: And people listening would, especially those that have been around for more than a year or two, would be forgiven if they thought that conversation - about the difficulty or the fact that five different definitions of a customer - must have happened 10 years ago. That's not happening today, right?
HEIDI LANFORD: It's happening today.
KIMBERLY NEVALA: But it's happening today. Now, well, let's talk then a little bit about expectations versus reality. We know that, broadly, when we're talking to organizations, the current focus on the latest and the greatest - so maybe that's generative AI, large language models - does run the risk of diverting organizations' focus or attention from the myriad of non-bleeding-edge analytics and even less exciting AI applications and the opportunities they afford the business. There's a real opportunity cost and risk there. It's something that Sarah Gibbons eloquently expressed on a previous episode when we were talking about the AI user experience.
But I'm wondering if there's also a gap in expectations versus reality relative to the data. That's drawing attention or misleading folks about the level of investment that really is required to build the stable data foundation. For instance, I get very nervous when I hear folks talking about pointing a large language model at poorly organized, noisy, disordered, dirty data with hopes of extracting value it seems almost magically from those dirty data sets. It's not garbage-in, garbage-out. In this case, it's going to be garbage-in, gospel-out.
But that does align with a lot of the PR. AI is just going to make it easy. Are you seeing that as well? And how do we address that misconception, if it is a misconception, within our organizations?
HEIDI LANFORD: Woo, there's a lot packed into that question, Kimberly…
KIMBERLY NEVALA: I know, I'm sorry.
[LAUGHING]
HEIDI LANFORD: No, it's OK. I'm going to try to address some of it. And then, cut me off if I'm going down a different path than where you wanted to go.
So let's take the case of an organization that's got some good foundational roots in place on the data side. But they have the - we'll call it the need for speed. They want to go fast. They want to start employing large language models, so that they hopefully can leapfrog and either build better insights or build better products that they want to start using. That's admirable. I love an experimental, let's throw 100 things in and if only 10 survive, that's great. I like that kind of culture. I thrive in that product innovation culture as opposed to a more heavily regulated, tamper down what you can do.
The issues are, like you said, the dirty data. We don't know what's in a publicly available LLM. Private LLMs that companies are doing in conjunction with some of the large, the bigger LLM companies… So there are instances where companies are partnering with some of the big firms and building their own private LLM. They're expensive, but they do allow you to take advantage of that technology.
However, you don't get whatever the group hive data sets that might be there. You have no idea what the quality of those data sets are. And we've spent decades talking about data certification and data governance and being able to trace data, data lineage, and all of these things. That doesn't come with, at least today, doesn't come with data that you have access to in a publicly available LLM. So it's buyer beware. There's risks out there.
Could you experiment with some of those things and then pull it in-house? Then do some more rigorous testing and try to understand what is influencing that LLM through those data sources. Then maybe try to procure them, bring them into your trusted environment and do something with that? That is an option. And that's something that companies should be considering and thinking about.
I'll pause there because there's a couple of other thoughts that I have on companies that maybe aren't quite at that point where they can start using them. But they think they want to use them because it's the talk of the town. So we could certainly talk about are we over-investing in our thinking that we can use LLMs when really we just need basic, good, old-fashioned analytics.
KIMBERLY NEVALA: Well, let's talk about that because, as listeners of the pod here will know, I have been skeptical about the tendency to take whatever is the latest and greatest and start to apply that as if it's a Swiss army knife. Not recognizing - in fact, I've gone so far to say as, you should never be talking about AI strategy except for at the top line on a big, pretty PowerPoint because AI is a portfolio of capabilities - there's a whole bunch of stuff under there, including the broader analytics portfolio.
So yeah, please expound a little bit on readiness and awareness and how folks should be thinking about this if they're not already highly advanced.
HEIDI LANFORD: So one of the ways that I like to start…many consulting clients that I have had and have worked with will come to me and say, hey, we're thinking of launching a bunch of pilots with these AI vendors. These AI vendors have called us. They've offered to do a POC. And we're really excited but we could use some help structuring out that. Or, could you help review like a statement of work or something?
In many of these cases, I will go back and ask the client, so what is the nagging business question that you wish you knew that you don't have the answer to? And in a lot of those cases, they fall into a very good, solid, rudimentary question of who are my best customers? What are they going to buy next? What products are most important and am I making the best margins on? What is an ideal buyer's journey look like? What does a good product roadmap look like?
Those types of questions can be best answered by the company's point of sale, ERP data, CRM data. Maybe throw in some demographics or some firmographics to round that out. If you've got any customer care, customer service, interaction data, layering all of that together and figuring out those ideal customer profiles or product roadmaps or things like that, that is a wonderful place to start.
It also focuses on some of the most important data that an organization needs to have accessible. High quality, clean, ready, consumable for lots of other decision-makers who are not data scientists or dashboard visualization experts.
Then you can start talking about maybe you get that ideal customer profile or segmentation or journey mapped out. That can take several months. That could take almost a year to figure out. Then you can start talking about, now I want to anticipate what customer X is going to buy next. And if I see that they haven't done something in 15 days after they received delivery of this product, what should we do next? Now you start talking about predictive analytics and you can start bringing in those elements of AI.
See, it's a natural progression. It also puts, I think, the right pressure on the data that needs to be cultivated and built for prime time use for AI applications going forward. It's so easy to get caught up in the AI marketing and hoopla that's going on because it's all we talk about every single day.
Everybody's talking about it, and we've been talking about it for two years now. But sometimes the good, old-fashioned basic stuff is going to get you really, really far and further than if you go down that, you know, trying to do something when you're not really ready for that yet.
KIMBERLY NEVALA: It's reminiscent of some of what we saw in the early days of Agile way, way back. Where a lot of the organizations who really wanted to adopt Agile were trying to solve a problem Agile couldn't solve. Which essentially was they had really poor communication and they were out of alignment with their business partners. They weren't able to understand, prioritize, manage expectations. And the thought was, Agile will allow us to just develop and deliver faster. Therefore, it will improve our relationship.
What we actually found was the organizations that were prepared to use Agile well were those that already had the existing rigor. They knew what the process was. They had good alignment. They actually had positive, collaborative relationships with their business partners that allowed them then to execute in an agile way. So the thing that organizations that were less mature or were struggling with that they thought Agile would solve for them was actually the foundational capability that was required in order to use Agile.
And again, it feels - maybe it's not a perfect analogy - but it feels a little bit like what we're seeing with a lot of organizations struggling to figure out where to start to deploy even advanced analytics, including different aspects of AI, today.
HEIDI LANFORD: I think it's a perfect analogy. And until you mentioned it when we were talking about it, I hadn't really drawn that parallel. But I actually think it's a perfect analogy.
I have worked in companies that have deployed Agile. Some have had that good infrastructure, good practices for deployment, good QC and testing, good data infrastructure to be able to build things, good software that you can use to enable the business to consume that data. And I've also seen organizations where they had creaky, old technology. And the business was very frustrated with stuff not getting done and waiting on the technology organization to get a myriad of projects done. And if you weren't the loudest voice, your team or organization didn't get stuff built.
So Agile, in a way, just takes all of those resources, puts them into different product groups, and gives them to the business. And the business then, just, it's essentially cramming more stuff into the top of the funnel. It's not actually, if not done well like you said, it's not actually solving the real problem. Which is maybe creating great products that mean something to our customers and the market that are advanced and are easy to use and state of the art. That will help gain more market share, snuff out some of the competition, all of those things that you desire, whether they're internal or external.
Creating more stuff just for the sake of creating more stuff so that the product owners are happy, that doesn't move that needle, like you said. So data is in the same way if you shift to a we'll call it a mass data-enablement capability versus a deliberate and focused one. And I'm not talking about data organizations controlling the flow of all of the data and how data is used. I'm talking about a focused effort that is deliberate and purposeful aligned to business objectives. Where you work hard on getting the right data that's going to move the biggest needles in the organization. And you make that data as available, as federated as possible so that people can consume it. Because the goal at the end of the day is to get people to use data to change how they do their day job. That is the goal.
That segues into a data literacy topic. Which is you need to be data literate enough to be able to consume and use that data. We're not talking about everyone being data scientists. We're talking about using data to either make business decisions or using data to build products to make customers happy and capture more of the market share. So focused effort, great foundation, and sometimes Agile can work well in that environment if that foundational - let's call it the horizontal - aspect isn’t forgotten with the vertical aspect of Agile.
KIMBERLY NEVALA: So I want to come back to the topic of data literacy. Before we do, though, I want to hearken back to the start. You mentioned the need to ensure that incentives are aligned, back when we were talking about the CDO role and just the data organization, in general.
You've also said or written that, something like the CDO or a data organization, that role is disruptive or it should be disruptive by nature. So can you talk about why this should be a disruptive role? And then how or why aligning incentives isn't happening today and why that's important.
HEIDI LANFORD: So, in the vast majority of organizations, the role is disruptive because CDOs are coming in and there are a lot of first time CDOs, like first time in the organization, CDOs. The CDO role as a C-level role is relatively new, past 10 to 15 years.
Companies bring somebody in to fill that role because something's not happening at the level that they want it to happen. So I wouldn't say a problem or an issue. There's an opportunity to use data and analytics more effectively. Either in running of your business, business operations, or incorporating more data into products. The fact that there is somebody that needs to come in and shepherd, build the strategy, and lead that for an organization, it's indicative that some changes need to happen. So you're already entering into a situation where the way things have been has not been as effective as it could be.
And so your job is to come in and make that more effective and more successful.
So by nature, to me, that means you're asking people to be thinking about change because you're going to be changing some things. Like, we're going to use this data over here to measure customer value - whether you're a new customer, how does that affect sales, compensation, et cetera - that's changing some things. Sales team, you can no longer bring a spreadsheet with your forecast for what deals are going to close in the quarter. You're going to use this data set that we have curated with your input to help inform that. And by the way, that's going to be a feed into how your commissions get paid out. You might be incented to acquire new customers a little bit more than renewing existing customers. And this data set is going to be the data set that informs the finance organization as to whether or not you, as a sales person, get that bump or not. So when we start tying that into compensation and incentives one of the incentives needs to be, in that little example, the sales leadership team needs to use that data to inform sales compensation.
Similarly, if we agree that we want to be data driven…and I'm just making this up. But if one of our areas is our product roadmap needs to be more aligned to what customers are submitting tickets about, that are issues with our product. Then we have to agree, as an organization, that we're going to use that data to be one of the major influencers of the product roadmap. If we say no, we're going to go with what the head of products feels is the most important thing in the market and ignore that, then we've made an investment in the data organization to get that product data great and usable. But we don't have an incentive around the product organization to utilize that data. So that's what I mean about aligned incentives.
I've seen this a lot where you're asking a data organization to invest in a certain area. But then, on the back end, the adoption metrics they're in PowerPoint. But they're not in the other people's annual development plans, their compensation criteria, and things like that. I believe strongly that, until that happens, this shared data strategy - which, it really does need to be a shared data strategy in an organization - is going to struggle to be shared. It's going to continue to be a thing that the CDO has to lead with maybe only a few supporters. And that makes it hard for the company to really realize the incredible value that companies can get from their data.
KIMBERLY NEVALA: Does that mean, then that - whether you're an official chief data officer or another analytics or data leadership role up to and including the C-suite - that your objectives and incentives should include, not be only, but include the same business objectives as your key customers? And that the objectives of your key customers have to also, their incentives need to include the data incentives or data-related incentives, as well? Really, it's not business objectives, incentives here over this delivery and technical objectives and deliverables over here for this incentive. But both of those components need to be (present) in every C-suite or business leaders and technical leaders.
HEIDI LANFORD: I think so. I almost think the objectives for a data leader should be about what the same ones that they are for the sales team and the product team and things like that. It's how I help the company achieve that objective is going to be a little nuanced for me. For example, I might have to acquire some data or build some data infrastructure or have some predictive models that are in place and are disseminated to the teams that can use them.
But then, similarly, I want to see adoption of those predictive models and use of those predictive models. If I'm asked to automate something, data collection, for example, then I also need to see that, if there are resources that are doing that kind of work that I'm being asked to automate, those resources need to either be reallocated or deemed redundant. Those are the kinds of things that I think make this stuff sticky and has a higher likelihood of being achievable. But CDO objectives need to be around the big-picture things, not around the how you're going to get there.
KIMBERLY NEVALA: Now let's go back to data literacy because, certainly, data literacy, similarly AI literacy is something that needs to be established within an organization both broadly and within specific contexts. But too often, this still seems to veer or dive very quickly into a mechanistic discussion of people understanding data quality or whatever that may be. That stuff's all important. But it seems to me that is perhaps missing the forest for the trees sometimes.
In your mind, what is the objective or outcome of a good data literacy program? And what should a data literate individual at any level of the organization be able to do?
HEIDI LANFORD: Yeah, great question.
So I see data literacy as the interpretation and ability to apply data to a decision-making process with confidence. That's sort of my kind of go-to definition. It does not mean that the person looking at data that we want to make a decision with it needs to have a PhD in statistics or have been a data scientist or anything that's super technical. It's knowing enough to feel confident to let that influence your decision.
So those measures of confidence could be in things like, say, if I'm in a marketing role and I'm looking at some research on a study about customers' opinions on a certain product and I'm thinking of enhancing my product that way. I, as a marketer, should know, I should be able to at least ask questions and find answers to this study such as, how big was the sample size? If they talked to 20 people, that's not enough. If it was only in one geographic area or say, if you're in a B2B-type company they were only talking to large enterprises and our product is useful for mid-market and SMBs as well. I would want to certainly be hesitant about using that data.
Similarly, if I'm the CFO and I'm trying to make decisions about changing our pricing and I'm looking at margin, I don't want to look at it one-dimensionally. If somebody gives me a spreadsheet or a dashboard and it just shows margin by customer or margin by partner and it doesn't include things like over time or across multiple products or including products and services or things like that.
Data literacy, to me, means we want those people to feel good enough about interrogating and asking questions about the data before they then use it. But then, we also want them to use that as a major component to making a decision.
KIMBERLY NEVALA: So does this, for organizations that do this well, require them to spend some time and effort in rethinking the collaboration and communication between business users and their data and analytics team?
i.e., business users need to be taught and encouraged to not only identify potential opportunities and to raise those opportunities where data might be useful. But also to ask the questions, ask really critical questions, about what was the data? Does this data really make sense? Where may this be falling short? What might we have missed? And for the data and analytics teams to be open to those questions and, in fact, encourage and solicit them as part of that discussion. Because, this is, again, one of those things that we've talked about to some extent for a long time. But I don't know that we've trained folks to behave in that way. Where you're a collective team. It's not an ask-and-answer engagement model.
HEIDI LANFORD: Well, so the good news on this story is I actually think that the shift has been happening for some time.
So when, for example, curriculum, even at high school levels and now college levels is becoming much more data infused even into subjects like humanities. I mean, of course, we would expect it in business schools and even undergrad business programs. As an example, the data science minor is the most popular minor at the University of Virginia. It used to be economics or something. It is the most popular minor. We're starting to see some universities put some data literacy and basic data-visualization-type courses as part of required curriculum. And they're also being offered as part of the - most schools have a mass science requirement - like a couple of courses in the undergraduate. But we're also seeing this starting to get infused into high-school level into classes like history and psychology and things like that. So that's the good news part.
Now, our workforce today, obviously we have people ranging from in their 20s to in their 60s and 70s. It is important for companies to employ some form of a data literacy program, in education. And it's not just a one-week training course or a 45-minute end-of-the-year thing you're doing on Christmas Eve or the 31st or whatever when you're trying to get all of those required online courses done. It needs to be built into part of the organization's cultural fabric.
Then there's the partnership aspect. One hospital network in the Midwest actually does a standing weekly office hours drop-in thing for all of the faculty and staff to come in and ask data questions of the data analytics team. No judging. You can ask something that you think might be a dumb question. There are no dumb questions. This organization has made this a standing thing, and people take advantage of it. So you've got research professors coming in. You've got doctors coming in. You've got nurses coming in. You've got PAs coming in asking about anything regarding data that that team has made available.
Those are the kinds of things that I think can tilt the culture and increase people's level of confidence about data-driven decision-making and also help educate so that we can have a data-driven populace. I mean, that's the goal. I hope this happens, though, even with my parents looking at election polling. Very sensitive topic, but we want the average citizen to be able to look at an election poll that they're seeing in the newspaper or on a news channel and be able to scrutinize it and ask the right questions about it. That's, to me, the goal of data literacy. If we can elevate that, we've made progress.
KIMBERLY NEVALA: Wouldn't it be nice if we had open, honest, public office hours for pollsters and report generators?
HEIDI LANFORD: Yeah.
KIMBERLY NEVALA: I love this idea, though, because, at the risk of - I always say, at the risk of - when I'm about to say something that it's not really a risk because I'm going to do it. That trite saying about, "A rising tide lifts all boats." But it would appear then, in having that office hours structure, not only are the business or the end users, they're the consumers of data and information, getting educated about the potential, the possibilities, and understanding the information and data itself. But the analytics and data folks that are staffing those hours are learning a lot about the business and the concerns and what the needs are by virtue of the questions that are being brought to them. So it's a really nice way to ..
HEIDI LANFORD: It's a win-win.
KIMBERLY NEVALA: …raise literacy on both sides. Now, I saw something recently. I'd be interested in your take on this. There was a post talking about data literacy. They stated - I wrote it down because I thought it was striking - that the goal was to make everyone data proficient, even if that was by means of or required giving everyone access to generative AI or an LLM. And it went on to state that, furthermore, data literacy is about adopting a culture that eliminates subject matter expertise. What is your reaction to that?
HEIDI LANFORD: [CHUCKLE] Well, that's a tough one. I guess you can never say never. But I don't think everybody wants to be a data scientist or have my background. And I don't want to be an expert in…
Frankly, you could say the same thing and use LLMs to eliminate the need for super creative marketers and CMOs who develop incredible brand campaigns and come up with fantastic logos and visualizations. I've tried to use of those visual tools. It's the same thing for image creation versus writing text. And they give you a good thing to start with but it's not something that I would want to use as the logo for my company going forward. I want a professional that thinks about a lot of different things to help shape and influence that. Maybe they could use an LLM to get some things going.
Anyway, my point is, I don't think that that world is going to take over creating, taking photography, or doing original works of art. Similarly, I don't think it will eliminate the need for the kind of work that we do, because it's still needed to help interpret. Can it be helpful and get us there faster and challenge us on things that we're thinking about or data sources that we want to include or exclude? Absolutely. It's awesome for that. But I don't think that that's the point. I think it's a tool in the toolkit. And that's how we need to think about it.
KIMBERLY NEVALA: So all of that being said, you've been doing this work and remain very passionate about it. I know you have two early-stage AI startups here looking at different aspects. I believe one is looking at AI readiness and the other one - I loved this, the tagline was cracking the culture code for AI - focused on enablement of senior executives.
As you look forward, given where we've been and where we're going, what most excites you about what's coming and the opportunities being afforded to us today?
HEIDI LANFORD: I love that AI has, I feel like, shed a little bit of a spotlight on the need for great data to be supporting it. If that can help influence investments that companies are making in that area, that is a win.
I am also really passionate that the whole world is seeing how useful data can be in informing anything. We've seen it in various pieces of our personal life with Amazon and with Netflix and self-driving cars and all of that. But that wasn't tangible for everyone. It was sort of real, but it wasn't real. It was removed.
What the LLM, ChatGPT, all of that surge has done is it has made it accessible and tangible. When you have people that can touch things and feel things, like hands on keys, they then see a little bit more. They have an additional window behind what's actually going on. I think that that increases the level of understanding and importance of those of us that are in the data and analytics field.
So that's what's exciting to me: the personalization and accessibility that it has given. So, while there are things to be a little nervous about or concerned, we've got to walk a tight line between the opportunities and also some of the risks, I think it's only positive moving forward. So I think it's a great time to be in this profession.
KIMBERLY NEVALA: That's awesome. We look forward to seeing the work that you continue to do and to support more broadly. Thank you so much for your time and insights today. This was really, really helpful.
HEIDI LANFORD: Fun conversation, Kimberly.
KIMBERLY NEVALA: Awesome. Well, thanks again. Hopefully, you'll come back and join us and we can talk about where we've made progress and where we might need to spend a little more time.
HEIDI LANFORD: Absolutely.
KIMBERLY NEVALA: All right, well, thank you all for joining us as well. If you'd like to continue learning from leaders and doers such as Heidi, please subscribe to Pondering AI now. You can catch us on any of your favorite podcatchers and, as of this month, also find us on YouTube.
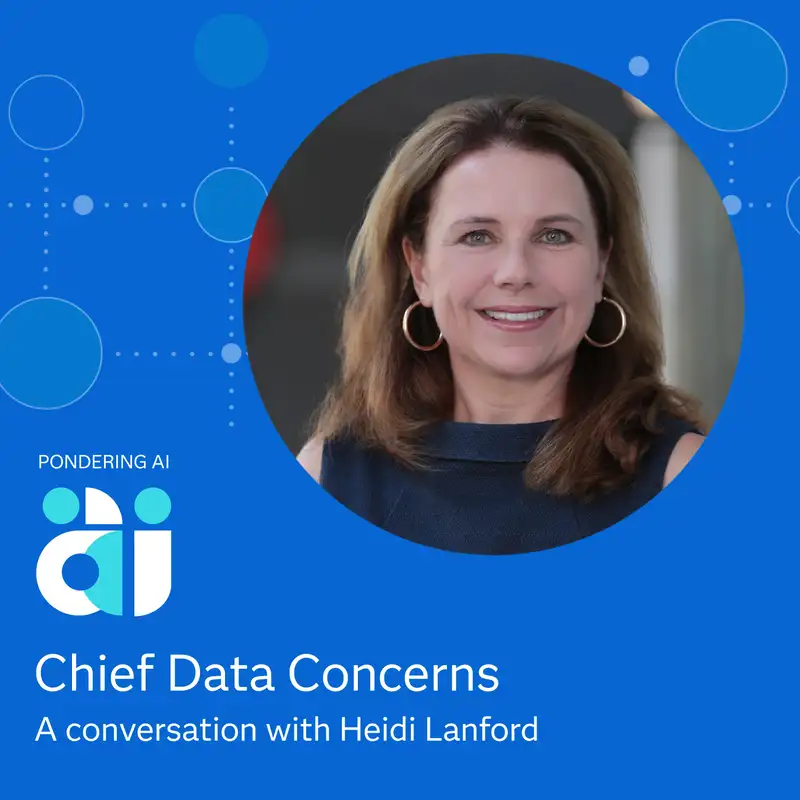