GAI in the Enterprise w/ Ganes Kesari
KIMBERLY NEVALA: Welcome to day 10 of our Insights and Intuition series here at Pondering AI. In this installment, Ganes Kesari reflects on generative AI in the enterprise. Welcome back, Ganes.
GANES KESARI: Thanks for having me back, Kimberly. Great talking to you again.
KIMBERLY NEVALA: In the time since we last talked, progress has continued apace. There has been no shortage or slowdown in both the hype and the excitement, and some of the reservations around the adoption of AI. What have been the most promising or striking developments you've seen relative to how companies are thinking about and/or adopting advanced analytics or AI?
GANES KESARI: Sure. That we've been seeing progress in the last few months is actually an understatement. We've been seeing a lot more than that in the months since we spoke. If I recall our conversation, we were talking about how ChatGPT was relatively new coming into the public domain and applications and the hype around it.
In the last few months, I have been pleasantly surprised with the pace of progress in the space. It’s gone way beyond education and we have several implementations in place. I'm seeing across the industry, organizations have implemented LLMs (large language models), broadly generative AI applications in production, which is fabulous. And, at the same time, really surprising compared to the earlier waves of AI we have seen just a few years ago.
So to explain, to give some additional context, compared to even the early stages of deep learning. People started talking about it, in the enterprise context, it was ~2014 or '15 where a lot of conversations were happening and some frameworks were available. And it took about four or five years or so before seeing some real-world applications and increase in the level of awareness. Compare that with generative AI: all of this has happened in months, which is incredible.
KIMBERLY NEVALA: So what do you think are the key enablers for organizations that have been able to embrace and, in fact, deploy this in a production setting given the relative newness and some of the very real limitations of the systems themselves?
GANES KESARI: Two things have really worked for organizations that have been able to progress.
One, picking the right use case, where are you applying it. We can talk about some areas of application I'm seeing generative AI being used most commonly in enterprises. So picking the right use case is number one. And number two is doing a quick integration and having a working version which they can have users play around with and use as a working pilot within the organization.
So these two, by the way, nothing new, same with any new technology or even with advanced analytics years ago. If you have the right pilot, the right use case, and an early prototype, that can work wonders. That continues to play out here.
I see a lot of applications. Broadly, I would say stakeholder engagement is one area: whether you are improving customer engagement or customer experience. An easy thing everyone can relate to is chatbots, how chatbots can become much smarter and actually useful after a long time. This could also be employee engagement: helping people find stuff internally and understand policies, simplify communication. So, broadly, stakeholder engagement is one area.
The second area is productivity improvement. Could be office tasks automation or technology automation, which includes coding, and other tasks that people do within organization. These two areas, I've seen these are low-hanging fruits best suited for using large language models, broadly generative AI. That has been working well for organizations that have gone in this direction.
KIMBERLY NEVALA: Have there been particular areas that customers have been interested in using these technologies where they've realized that either the technology is either not well-suited or they're just not ready to go? Have there been any that have been striking to you where somebody went out to test this and have decided not to move forward in that area, currently?
GANES KESARI: Use of these models for more predictive tasks has not really been successful yet.
For example, if it is understanding of text, understanding within quotes, and being able to answer questions and summarize, that has been area of strength. Whereas if you're trying to do very accurate, fine-grained classification of text - for example, sentiment classification or even customer journey classification at a very fine-grained level - there are areas where it works and areas where it gets it wrong. It doesn't get the context. And definitely prediction kind of use cases are still far away.
So those are areas where organizations have not been as successful. From within an enterprise standpoint, I see that the first set of tasks I mentioned is where they have been relatively more successful. For example, simplifying employment policies, or customer contracts, or the regulatory documents. All of those are areas where people have been successful using it.
KIMBERLY NEVALA: It strikes me that this might underscore a key point you made the last time we talked. Which is that these technologies are just another tool in the toolbox. So organizations that are looking to apply them sort of in a peanut butter manner, smear them all over the organization to solve anything and everything, are likely to be sadly disappointed at the very least, if not taking on major risk.
But those that are looking at them, understanding the capabilities, understanding the limitations, and applying them for the proper tasks in conjunction with a broader suite of capabilities, AI and otherwise, are more likely to be successful.
GANES KESARI: Very true. I think that's well-put. There's still a case for building your linear regression model, still a case for building your BI dashboards, nothing takes away that budget and efforts yet. Again, yet is a word which keeps coming back. But they are important. Like you mentioned, just layering it on top just because everyone else is using it, that's a recipe for failure.
KIMBERLY NEVALA: So what should we anticipate? What do you forecast happening as we make the turn here into the new year?
GANES KESARI: There are a couple of things I'm really excited about.
One thing I have been telling people to look out for throughout this year has been a multimodal AI. At the start of this year while people were excited about text chat, I was saying how it could be incredible if we cannot just text but also talk, see, and have a real conversation with an AI.
Again, conversation can sound frightening. But from an enterprise application standpoint, having a meaningful usage of these applications will be much more powerful if we are able to share pictures, and talk, and get some quick answers as opposed to having to type and structure that into a text prompt. So that is what I continue to be very excited about. Already, ChatGPT, earlier this month launched image integration as well. So you can upload pictures into ChatGPT - I think in most geographies it is already live - and it can ask questions about pictures. So that is showing a lot of promise.
Now, imagine if you're able to talk to it freely and do all of these interchangeably. For example, ask a question, and then paste a picture in a follow on. And then copy/paste a document, dump it or point to a link. And that's what I mean by a complete, meaningful conversation to accomplish and get to a decision end of the day. That, I think, we will see in the coming year mature. Again, much faster than what people are thinking. That will be a game-changer.
KIMBERLY NEVALA: An unfair question, but I'm going to ask it anyway. If there were a singular piece of advice or activity that you would recommend people focus on - what is the thing they need to do and get right - to be able to leverage these technologies most effectively and safely?
GANES KESARI: If it is just one word, I would say upskilling.
Organizations can tap into this only if they enable their teams, whether it's a technology organization or serving in a different business domain. The people across the roles, across the divisions have to not just be aware of it but also should be capable of using it. So that is an effort I see. Again, organizations which are way ahead and who already have realized that this is a game-changer, they are training their workforce in tens of thousands. That's happening, but only in a handful of organizations today.
So that would be my very strong recommendation. Take up this training and definitely go beyond education in terms of practicality where can people use it by role, by activity, and provide them with the tools. If it means getting some subscriptions, whether it is those end-user tools, or APIs, or the cloud, enable that as well along with education. That would be my recommendation.
KIMBERLY NEVALA: So engaging and enabling not just literacy but facility?
GANES KESARI: Exactly. Yes, right.
KIMBERLY NEVALA: Awesome. Well, thank you, Ganes.
GANES KESARI: This has been good. I think there's a lot happening in the space so certainly there are other aspects. But for a quick take, this was good.
KIMBERLY NEVALA: 12 days of Pondering AI continues tomorrow. Subscribe now for more insights into what's happening now and what to expect next in the ever-fascinating world of AI.
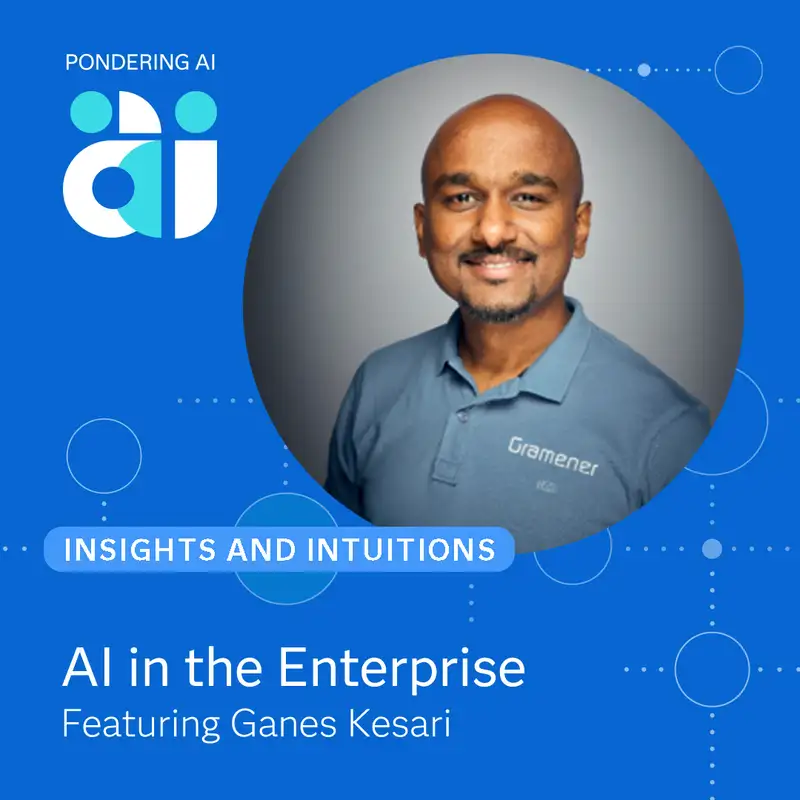