Making Model Decisions w/ Dr. Erica Thompson
KIMBERLY NEVALA: Welcome to day eight of Insights and Intuitions with Pondering AI. In this episode, Dr. Erica Thompson reflects on making model decisions. Hey, Erica. Thanks for joining us again.
ERICA THOMPSON: Hi, Kimberly. Nice to see you.
KIMBERLY NEVALA: Since the last time we talked, your amazing book, Escape from Model Land, has come out. So congratulations.
ERICA THOMPSON: Thank you. Yeah. It's super exciting.
KIMBERLY NEVALA: Since then, has our collective understanding of how to apply models correctly and jump from model land to real life improved? Why or why not?
ERICA THOMPSON: Wow. I don't know. I suppose lots of things have changed, haven't they?
We've of seen more fallout of the consequences of decision making based on mathematical models during the pandemic. We've seen all sorts of hype, and maybe the crash of the hype, and maybe different hype of AI models, large language models. So I think our understanding has moved on in a lot of ways. Maybe practice has lagged behind a bit.
KIMBERLY NEVALA: You mentioned the large language models, generative AI. How has that development contributed or stymied our ability to look at and apply models rationally?
ERICA THOMPSON: Well, it's helped because perhaps the large language models have such obvious gaping deficiencies. And I think that's actually really nice in that it helped to expose some of these flaws, so that we can have more of a conversation around them.
There's been some really exciting work. For example, about how the biases in the training set, the biases in the way that humans think then get propagated through into the way that these large language models operate. And of course, the large language models are just the ones that are fairly easy to see it in. And these biases are throughout. Anything that we do is going to encapsulate the biases that we have in our training set.
So we have to be particularly careful in how we think about that. Whether we want to propagate those biases or whether we want to try to design them out. Because if we want to design them out, then we have to do that proactively.
KIMBERLY NEVALA: With that being said, it does seem that large language models, ChatGPT and all of its ilk, are being applied to absolutely everything and anything these days. Have we done a good job of prepping the public or the broader collective with the information required to use these properly?
ERICA THOMPSON: Yeah. Well, I mean, no. No. We've done a terrible job. But as I said, it helps us to start that conversation. So we have these large language models and they're being used in many different ways. And one of the things that we have seen is how incredibly powerful and incredibly effective they can be in certain limited situations where they can do a really great job.
What perhaps we've failed to do is to think sufficiently carefully about the boundaries. Where is it that they stop being useful and start being actively misleading. And then start being genuinely dangerous. How do we distinguish those? And having that conversation about how to distinguish the good, from the bad, from the ugly is really important.
KIMBERLY NEVALA: Large language models and generative AI aside, have there been other consequential developments or activities that you'd like to draw people's attention to that may not have received their due as a result of this all-consuming fog around those elements?
ERICA THOMPSON: Yeah. I mean, apart from my book obviously, which is super exciting.
One of the things that I've been really heartened by in the response to my book is I was sort of terrified that people would hate it and come back with lots of criticism. But actually, the kind of reflections that I've had back have been from people saying, oh, this really resonates. We're doing something in some area that I know absolutely nothing about – law or transport modeling or investor state dispute settlement was one of the recent ones. And I don't know much about this at all.
But they come back and they say, your thoughts on how models are used to support decision making and the ways that can go wrong really resonates. So that's been really nice as a sort of integrative synthesis over the last year or so. To be able to try and bring together some more of those threads and learn from people who are struggling with these questions in other application areas that I'm less familiar with.
I've found that really inspiring and exciting to think that there are wider communities of people involved in these questions who we can work to bring together.
KIMBERLY NEVALA: To your earlier point, it does seem that a lot of the discussion around decision making and "data-driven” decision making is becoming both front and center and a bit more robust.
What do you anticipate or project we can expect to see in the area of decision making and how we think about making decisions with analytics in 2024?
ERICA THOMPSON: Well, I suppose on one strand there is the continued improvement of data. We are measuring more things. More data is available. There is more computing power available to harness. There are more fancy models like the large AI models to process that data, to use it, and to start pushing it towards applications.
So I think we'll see a lot more of the bad and the difficult: of people sort of rushing into doing things without perhaps having sufficiently examined why and what the consequences could be. But also we're pushing on in the other direction as well. That as these things get rolled out, there is more of the criticism. There is more of the interest in working out where the limits are and finding a balance between how we can use these powerful methods responsibly for the benefits that they do genuinely bring. Without stepping over the line into accidentally doing something that's going to be a really bad idea.
KIMBERLY NEVALA: In that regard, what advice would you give folk? Where would you encourage them to focus their energy or attention as we move forward in this, I can't say brave new world, because it's not so new these days…
ERICA THOMPSON: There's loads of exciting stuff at the edges, and in the gaps. The sort of interdisciplinary boundaries. The surfaces between domain expertise and say computer science expertise and ethics, or feminism, or postcolonial studies, or all of these sorts of things. There's a real melting pot there of people coming in with really different backgrounds. And saying, actually what's the fundamental question here?
The fundamental question here is A: about the limits to our knowledge. And B: the limits to our wisdom if you like. Our ability to make good decisions rather than just defining something to be optimal. And then writing a program that will make the computer make the optimal decision in the shortest possible time. Actually, thinking more humanistically about what it is that we're actually trying to do.
Those are hugely exciting discussions and ones that get to the core of what we are doing as a society. Why are we here? What's the whole point of it? And I think that's fun, exciting, fruitful, and really important.
KIMBERLY NEVALA: With any luck, that will come from your mouth to our collective ears and 2024 will be the year of wisdom. I love that. Thank you, Erica. I really appreciate the time.
ERICA THOMPSON: Thank you very much.
KIMBERLY NEVALA: 12 Days of Pondering AI continues tomorrow. Subscribe now for more insights into what's happening now, and what to expect next, in the always fascinating world of AI.
Creators and Guests
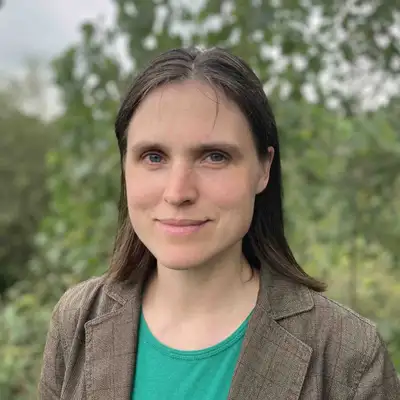
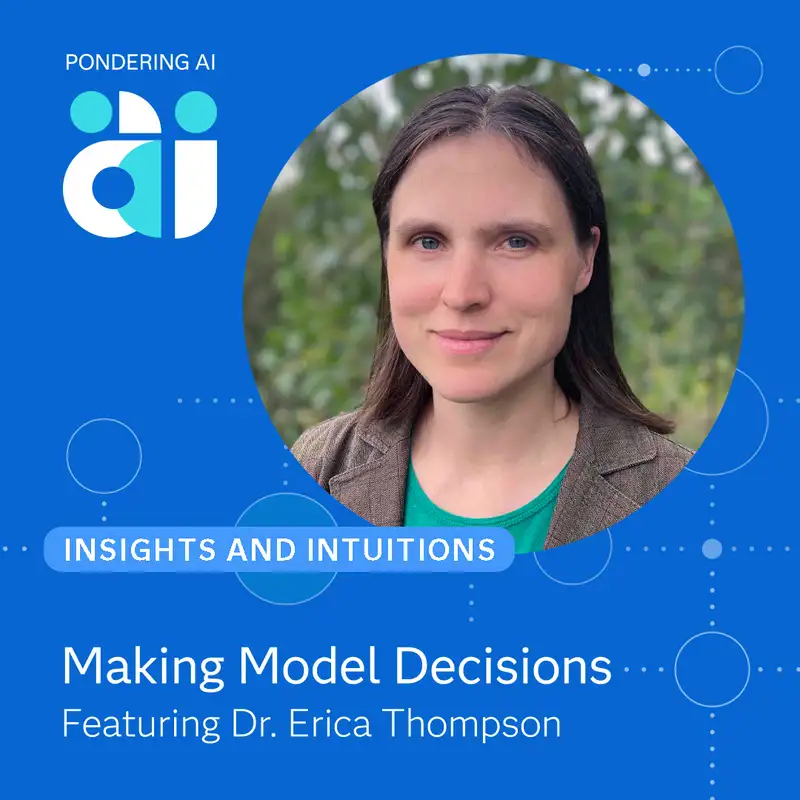