GAI Detection and Protection w/ Ilke Demir
KIMBERLY NEVALA: Welcome to Day 5 of Insights and Intuitions with Pondering AI. In this installment, Ilke Demir reflects on generative AI detection and protection.
Hi, Ilke. Thank you for coming back and joining us again.
ILKE DEMIR: Hi, Kimberly. It's a pleasure to be back.
KIMBERLY NEVALA: Well, I think it's fair to say that the furor, the excitement, and potentially the fear over generative AI and LLMs has not died down since the last time we spoke.
You are in the center of the storm working on how do we not only leverage these productively but also safely. How do we safeguard their use, both in terms of our individual rights and the collective best interest. What have you seen as the most consequential developments in the generative AI space over the last six months or so?
ILKE DEMIR: Good question. So starting from my own bubble, we actually demonstrated defect detection and defect detection as a service last week in Intel Innovation. And we are working towards Trusted Media development to build this online trusted feature.
One latest addition to our suite of tools is called My Art My Choice. We are bringing this choice to all the content owners, face owners, style owners, artists, content scene owners about protecting their content from being used in diffusion models.
KIMBERLY NEVALA: So how does that work?
ILKE DEMIR: So that's actually an adversarial attack on diffusion models. We are trying to protect an image. We learn to generate a super similar version of the image perceptually but adversarially it has these very little perturbations that makes diffusion models break. The output is looking nothing like the style, nothing like the image, et cetera. We also tried it on faces and other domains and it works pretty well.
So if anyone wants to protect their work from being used in diffusion models without their consent, without granting their copyright, et cetera, they can say, My Art My Choice, and hopefully it will be available to them. So that's within our bubble. [LAUGHS]
KIMBERLY NEVALA: That's excellent. Obviously, this will work for folks on a move forward basis. But if you've got art that's already out there - these things have been out vacuuming up any content, digital content available on the web -- have there been any developments or research on how folks who may want to pull that out of a previous training set can do so?
ILKE DEMIR: Excellent question. So there is some research around that and how to make models forget. Or, how to even understand how much one sample is contributing to the whole latent space of one model, all learned space of one model.
I love that research, but I haven't seen anything that is coming out from there. Some industries, some companies should be building them internally if they are promising to compensate artists' work for their contribution in generative models. Any company that is promising that should be building that internal model about understanding how much one sample contributes to the whole diffusion model. And hopefully, that will happen at some point, but I haven't seen any technological solution there yet.
KIMBERLY NEVALA: Sounds like an area of active research. It'll be interesting to see if those companies pursue that in the interest of trying to minimize the payout to somebody. To say this really doesn't have that much of an impact versus trying to optimize value for those creators. We'll hope that that comes out on the side of good.
What are you keeping an eye on outside of your bubble that you think is potentially most interesting or impactful moving forward?
ILKE DEMIR: Right. We mentioned this a little bit in our previous chat, but the provenance integration. That is what I'm keeping my eye on. That's also what we are also working on.
There are different types of provenance. You can say that this model belongs to someone, or this sample belongs to someone, or this data set belongs to someone, et cetera. How do we integrate all of this information within the whole generative creation process?
So my face or my art. I want it to be known that the origin was me throughout - wherever it goes - without being manipulated without my consent, without being deleted without my consent. And that will make the answer to the previous question much easier. If it is known throughout the process, then we don't need to decide how much my sample contributes to a diffusion model because it will be known at the output of the diffusion model that it belongs to me.
So how can we have provenance integration and provenance preservation throughout the synthetic data generation process? That is one big question to answer a lot of our problems right now.
KIMBERLY NEVALA: That may have prefaced the answer to the next question I'm going to ask you. As we look out across the next year, what are you most excited to see?
ILKE DEMIR: I don't want to be biased by our own work, but I believe in our mission. I believe in our mission to be generalized across my research colleagues and that we need to bring humans to the center of all of this. Not forget about our research - Trusted Media research - but for everyone. This is not just some black box that is giving a sentence, getting an image. We need humans to be in the middle of that.
For humans to be in the middle of that, the results should be explainable. We should know why that model says so. We should know how we can control them. How can we do controllable generation beyond ControlNets? ControlNet is a good thing. But we can give some guidance that is not really controlling. There are research outputs that are doing drag and drops or some copy paste, et cetera. So these are some nice things around that control.
How we can bring the ownership and transparency into that process to make it more human-centric? It's great that all of the beautiful minds are thinking about all these technological advancements. But we should take a step back, put humans back into that equation and see how we interact with them, how we understand them, how we interpret them.
KIMBERLY NEVALA: As that work goes on, there are understandably a lot of humans who are very excited. They may or may not understand the underpinnings of the technology underneath all of these tools. And god knows they have exploded, the number of tools out there these days.
Is there any piece of advice you would give in this time where we don't necessarily have these controls, we don't have the transparency? There may not even be the literacy. Is there a single piece of advice for those who want to engage with these tools, are excited about it, but really do want to do that in a way that is ethical and responsible?
ILKE DEMIR: Whichever tool they are using, they should find out the source. Or what are the RAI - the Responsible or ethical AI pillars - that it was built on. If it's a company tool, it may be a little bit more structured such that it actually goes through that (checklist). Sometimes companies don't give the model cards or which data set it was built on.
So I know this is asking too much before you use a tool: go check the source. But that makes all of us aware. That makes all of us a conscious part of this ecosystem. Instead of just blindly downloading a tool from GitHub, or using a company's super newest offering, or clicking through that 10,000 liked Twitter thread on five ways to use this tool. Is that a good tool to start with? I would say check the sources. Check how it was done. And do not believe the output. That is it.
We were checking with some friends what one of the latest AI chatbots is saying about me. It seems I am from three different universities which I never studied at.
KIMBERLY NEVALA: [LAUGHS]
ILKE DEMIR: I had so many collaborators that I never worked with. I had so many papers I was working on in health care or something. I have no idea. And that was just a chat conversation in one of the messaging tools, you know? And I'm like, wow. That's me? OK, good to know.
And I don't even know what to say because people may be asking to really get that information. I'm asking because I’m toying with the tool. But if someone is really interested about my academic background and ask these questions. I wasn't in those universities, so… [LAUGHS].
So yeah, just check the source. And checking the source is not asking another chatbot. Checking the source means credible scientific papers or credible websites, et cetera.
KIMBERLY NEVALA: [LAUGHS] And for the record, Ilke's background is quite impressive all on its own.
ILKE DEMIR: Thank you. [LAUGHS]
KIMBERLY NEVALA: Perhaps the fact that it fabricates in that way is an indication of exactly how pervasive your influence is, even if you never studied at all those places.
ILKE DEMIR: Sure. In a parallel universe, in a different timeline. [LAUGHTER]
KIMBERLY NEVALA: Awesome. Great advice and great insights, Ilke. Thank you for joining us again.
ILKE DEMIR: Of course. Thank you.
[MUSIC PLAYING]
KIMBERLY NEVALA: 12 Days of Pondering AI continues tomorrow. Subscribe now for more insights into what's happening now and what to expect next in the ever-fascinating world of AI.
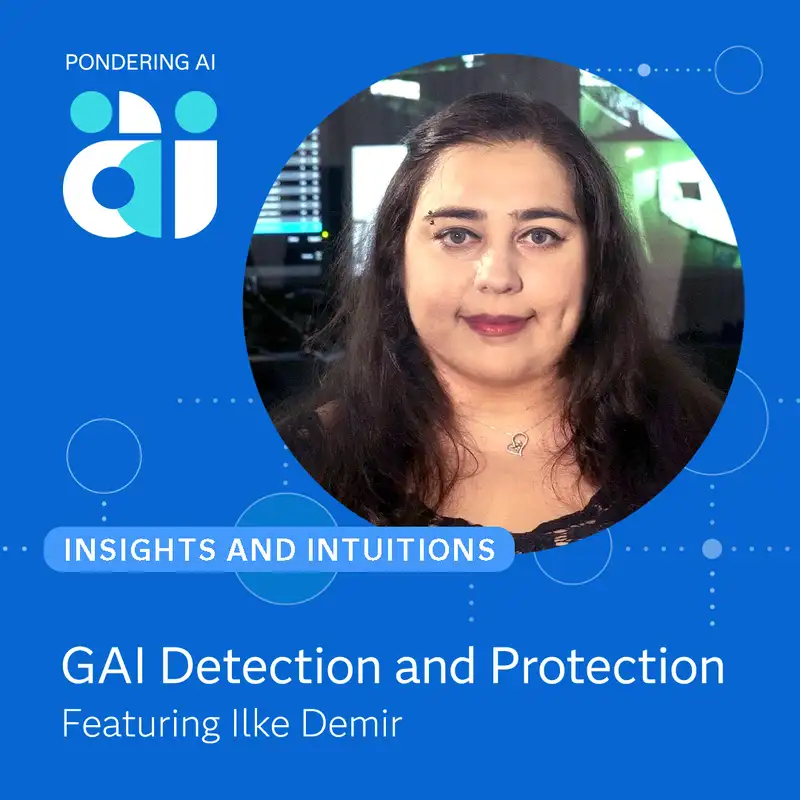