Generative AI: Unreal Realities with Ilke Demir
KIMBERLY NEVALA: Welcome to Pondering AI. My name is Kimberly Nevala. And I want to thank you for joining us as we ponder the reality of AI, for better and for worse, with a diverse group of innovators, advocates, and data professionals.
In this episode I'm so pleased to bring you Ilke Demir. Ilke is a senior staff research scientist at Intel, where she focuses on the overlap of computer vision and machine learning. We're going to be discussing the fast-evolving world of generative AI, deepfake detection and more. So thank you for joining us, Ilke.
ILKE DEMIR: Thank you for the invitation. Happy to be here.
KIMBERLY NEVALA: Tell us a little bit about your background for folks that aren't familiar with you. And what does it mean when you say you're working on digitizing the real world?
ILKE DEMIR: Of course. So I did my PhD in computer science. And my specialization was in proceduralization, which is a big word. So proceduralization is taking any 3D data-- that can be buildings, manufacturing data, humans, whatever digital data we have-- and trying to extract an interpretable and controllable representation from that digital 3D input. And so that was my PhD. And it involves computer vision, machine learning, computer graphics, and how we can do both. But mostly from a machine learning - traditional machine learning standpoint - how we can actually understand 3D data.
Then I worked at Meta and developed more generative models and more deep learning models for understanding people in virtual reality, understanding satellite data, understanding 3D reconstruction at scale, so many different projects. Then I had a brief startup experience where we were also looking at deep learning models for compute efficient structures. And then I started at Intel as the research director of Intel Studios, which was the huge volumetric reconstruction space that we were shooting 3D movies.
KIMBERLY NEVALA: Wow! I actually didn't realize you were shooting 3D movies. So I learned something in the first 2 minutes of this episode, which is fantastic.
Now, these days you cannot literally turn around without bumping into a conversation about things like ChatGPT. Can you level set our understanding of what generative AI is? And more specifically, what is the range of capabilities we get with generative AI, broadly?
ILKE DEMIR: Absolutely. So I know that ChatGPT is giving us outputs that are not exactly the inputs. But I would hesitate to call it generative AI in the sense that it is actually a conversational AI based on a large language model. It is not actually generative in the sense of traditional generative models. Still, I know from the public view, it is a generative AI, so definitely we can discuss.
So the current capabilities of generative AI are limited in the sense of we should not expect them to create very novel things that they haven't seen before. And since the training data and all the data sets that are used for such models, maybe ChatGPT, maybe Dall-E, maybe Stable Diffusion, are not really understood, and moderated, and analyzed in a way that it is correct.
So the output of these models may also not be correct. And sometimes it's not just from the data set, but the way that they are built are not preserving structure. Or they are not preserving the actual information content. And these are all things that is currently out of (the scope of current) generative AI capabilities.
If you look at it from the control standpoint, that is also a downside of current generative AI: that there's no control. If there is six-fingers generated by Stable Diffusion, you cannot go and fix it; oh, I would like to make five fingers. So I think it's very important for the future of generative AI to find an intersection of traditional generative models versus AI-based generative models. And how we can take the control element from one to apply to the other.
KIMBERLY NEVALA: OK. So really simply then for folks who may not have the deeper background, when we think about generative AI, we're talking about systems that can, I don't if compose - that sounds very human to me, but can generate things like images, videos, maybe voice, speech, music. Is that right? That’s a good mental model for what we're talking about is the outputs of generative models or generative AI?
ILKE DEMIR: Yes. And there are different models and different input-output pairs. So generate images from text versus just generating abstract images. Or generating 3D imagines from text, et cetera. All of these pairings for generative AI has been developed for some time.
KIMBERLY NEVALA: OK. And is there a simple example that you can give that provides a contrast or helps us understand the differentiation you're making between a traditional generative model and generative AI?
ILKE DEMIR: Generative models go all the way back to shape grammars, which was introduced by Stiny in 1972. So if you look at those generative models, they are grammars - which are like language - but for shapes.
So these are called L-Systems. And the very basic version is that imagine you have a turtle and the turtle has three commands. It can turn left. It can go straight. And it can go right. So by just these three commands - go left, go straight, go right - you can actually create so many shapes. And it won't be, of course, a very photorealistic image, et cetera. But for a shape world, it is actually the first way that a generative model was introduced. So in that sense, you have some set of rules, which are grammars, to create shapes, create 3D models, create 2D models, et cetera.
What generative AI models are doing is instead of being dependent on that grammar they are actually having the understanding of the generative model directly from the data.
Now, if we contrast these two, traditional models are interpretable. So you know that this shape was created by left, right, straight commands, in the very basic sense. But for generative AI, there is no such primitives. There is no such operations. There is no such control elements that are understandable by humans.
How we generate things are maybe tuning some parameters, saying that we want to increase the gender, or walk in the latent vector of age, et cetera. But it's not really giving you any shape or structural command that you can apply in a generative model. So in that contrast, I want to take all of these very nicely interpretable models and somehow extract those controllable grammars or controllable way of representing the generative model from generative AI.
KIMBERLY NEVALA: And so, again, for those of us who are not quite as adept in the detail of the technical language, essentially, if we want to then generate, for instance, images - maybe it's of people. Or we want to generate language, video, music. We're giving lots and lots of examples of pictures of people, for instance, and feeding that into these models. And then asking them to generate a person or people, or so on and so forth. And it's in that training set that all the goodness and the badness of generative AI - or AI in general - come about.
I want to talk a little bit about that. I know you do work at Intel both in generative AI and also in detecting what has been generated. So what's real from - well, I will say real from fake - but I'm not sure I mean fake in the traditional sense.
Before we go there, though, one other term: how do we differentiate or how do you think about what is a deepfake? Is anything generated - any picture or any video generated by AI - considered a deepfake? Or what is the characteristic that makes something a deepfake? Is it intent? What is it?
ILKE DEMIR: Relatively, it's a new term. So people have different associations with deepfake term, but basically, deepfakes are mostly portrait videos, face videos, or voice, or images where the actor or the action of the actor is not real. And the differentiating factor that it's deep is because it is created by a deep neural network or a complex algorithmic approach. And that it is very photorealistic. It is very hard to distinguish it from the real ones.
Now, if you look at deepfakes from the intent point. If it is with good intent and a little bit less convincing, or in a different domain like 3D, it may be called synthetic data. Because the synthetic data is also generated faces that are the same approaches. But if it is used for more evil purposes, for misinformation, for impersonation, et cetera, then it may be more connected with deepfakes term.
KIMBERLY NEVALA: So in a lot of cases, is it right that we shouldn't necessarily hear deepfake and go immediately to a negative connotation? Although, I think from the popular perspective, that's where a lot of the conversation is: the use of these to generate a fake image to show somebody saying something they didn't say. Usually to influence, usually negatively, someone's perception to defraud, to create forgeries, et cetera, et cetera.
You've argued that those are all certainly concerns. And all of those negative applications are not a reason for us to throw - and I hate this term, but I'm going to use it anyway - throw the baby out with the bathwater - when it comes to generative AI. Because there are, in fact, constructive uses of deepfakes. Again, using that as a more neutral term.
ILKE DEMIR: Absolutely.
KIMBERLY NEVALA: So can you tell us about what some of those constructive uses might be?
ILKE DEMIR: Yeah, absolutely. I may be giving the same example over and over, but I really like that example. So I will go from one example to the generalization of the deepfakes for good use cases.
So there's an example documentary that one director shot to explain the oppression that certain minorities in a country are going through. And how the government is actually treating them very differently because they are LGBTQ communities, et cetera.
Now, if the director really wanted to share their emotions, expressions, facial attributes, but he cannot do that without actually revealing their ID. So what he did? He actually used deepfakes. So he kept all the expressions, micro-expressions, the emotion. But instead of masking, or blurring, or making it a non-human thing that is talking with just a voice, he used deepfakes to just mask the identity and keep the video to keep the documentary integrity as much as possible.
So this is a deepfakes work because the subjects are actually consenting. They want to share their experiences, but they don't want their ID to be shown. And that is a very powerful way of actually using that. So, deepfakes for good if they are done with consent, if they are done with different design priors. So that they are not impersonating, or they are actually helping people for anonymization, helping people for privacy preservation, then they can actually be good.
In that case, we also have a new project called My Face My Choice that’s also another example for deepfakes for good. So we have thousands of, maybe hundreds - I don't know, everyone's different - photos that are online, right? Either taken with our consent or without our consent. Or our friends are very innocently putting them on social media but we don't want to be in them. In this case, we actually designed this social photo sharing platform where there are access models based on whether you appear in that photo or not. As opposed to whether you want to be associated with that photo or not.
So association with photo is basically those tagging options: someone tags you. Oh, my name is there. I'm in that photo, yes. What happens if I am tagged? My face keeps living there, but I don't want my face to keep living there. Maybe you're not in the platform and don't want to be associated with that, right?
So in My Face My Choice, everyone that you don't want them to see you, you are swapped with a deepfake. And where does that deepfake come from? Completely synthetic images. So we are not switching you with someone else. But we are switching you with a not existing person. And in the embedding space, these are the furthest faces: which means the most dissimilar face to your face. So it is actually quantifiably guaranteed that you won't be recognized in that photo later on. But someone else looking at a photo will see a nice person smiling if you were smiling or drinking if you were drinking. But it's not you anymore.
One thing we also did on top of that is we asked seven different facial recognition algorithms. Face recognition is also a very controversial area: should it be done, should not be done, who should be doing it, et cetera. So we asked these seven different facial recognition algorithms and on the average we break down by 65%. So 65% of the time, automatic facial recognition algorithms cannot detect that it was you after My Face My Choice is applied.
KIMBERLY NEVALA: Interesting, because that's still a fairly large percentage of the time that it can identify it as you.
ILKE DEMIR: Right. But the motivation is to actually create these so much that we actually explode their search space. Before, let's say they were looking at the similarity of 2 billion faces, because that's how many faces that they stored, right? But the more that we create those, the more we are actually exporting that 2 billion to 200 billion, or even more. And in that case, they will be very, very unsure. Saying that, oh, I'm not sure that this is Nevala, but I don't know. This Nevala, this Nevala, and this Nevala…they are all very similar. So I'm confused. What will happen? So the motivation is that now it can be 65% but the more we explode the search space of those facial recognition algorithms it will decrease and decrease and decrease.
KIMBERLY NEVALA: OK. Interesting. And facial recognition, that is an interesting place, because there's a lot of ethical issues that have come up. They are not as accurate for certain populations if you're not, in fact, represented in the data set. And there's a lot of discussion about when and where it's even appropriate to use it.
So there are situations where you could say, well, it's probably better that you're not recognizable. Except if, in fact, against our better judgment perhaps, socially or individually, we're using it in cases like law enforcement. Or this is determining whether you get into Madison Square Garden or not, these silly things. You could see a flip side. To some extent, you're decreasing the accuracy of facial recognition and it's not either good or bad. It's very situational whether the impact there is pro or con.
ILKE DEMIR: I mean, as I said, this project is towards social media. And I don't think in social media, you need to be recognized in images or videos that you don't want to be recognized. For other purposes, yes, maybe. But for social media, My Face My Choice.
KIMBERLY NEVALA: Yeah, I like that. So there has been a spate of articles recently about the fact that people perceive that generated pics - pictures of people, their faces - assuming they don't have six fingers or three hands - that faces in particular look, quote, unquote, "more real than pictures of real people." And I'm not quite sure what to make of that. It's a little disconcerting on a couple of levels. So I'd be interested in your thoughts.
One is from that human perspective. You talked about social media that there's quite the escalation of issues. And we a lot of times talk about it - I think in terms of girls or women, but it happens with boys and me - I think it's pretty gender neutral in terms of body issues or mental health issues coming around. Because we are constantly bombarded by these visions of reality, this airbrushed perfection and beautiful lives that are just continuous moments and streams of joy that doesn't reflect reality.
And I wonder if as we begin to generate more images and people see those images, are we simultaneously also over time deviating from projecting images that are, in fact, in any way realistic or achievable for people? What's the impact on the human scale for that?
ILKE DEMIR: That's a really great question. And there are so many perspectives. I will try to order my thoughts.
So I want to go back to the procedural world to give an example. There was a very nice user study from Peter Wonka's group - he's an old computer graphics researcher. They wanted to evaluate the perception on the output of traditional generative models. And in that case, let's say buildings.
And these generative models are creating beautiful buildings: all the windows are crystal clear, all the walls very clean. If there is trees, trees are in the perfect shape, et cetera. And then they actually twisted that output a little bit. They manually added some dirt on the walls, some windows open, some windows closed. That looks like all those imperfections, you know? And they did a user study saying, oh, which one is more real? Which one do you think is a photograph versus a generated image, et cetera? And of course, the hand-tuned, imperfected outputs were found to be more real than the perfect outputs.
Now we are seeing the reverse trend, maybe. That the more that we see those perfect images, we are actually pushing our reality to be more towards that unreal reality - pun to the episode. Which is maybe harmful in the sense that we are trying to bias our perspective based on those perfect perspectives. But what makes us human and what makes us unique is actually those - I don't know - my hair not being perfect or that missing earring. All of these things that may be outliers in the data set is actually what is making us us. And in that sense, maybe generative will get so real that at some point it will also capture the imperfect beautifulness that we have in humans. I just hope that the perception of the humanity will not be shaped so much by that time that we will forget us and go back to our reality.
KIMBERLY NEVALA: We're imperfectly perfect as humans.
So I've also wondered, because AI systems, machine learning, they learn on the data that is provided to learn from. As the amount of generated content grows, potentially exponentially, we could see that generated, whether generated faces, or videos - I mean, as you said, it could be buildings. It could really be anything, not just the human form. It has the potential to grow at a scale and quickly outpace organically generated images, if you will. I'll use organic here to mean that we didn't use an AI model to generate them.
Is there a risk that our systems then increasingly are only effective or efficient and their accuracy is only good against images and patterns that they themselves have generated?
ILKE DEMIR: Well, first of all, what is the value of generated imagery versus, let's say, organic imagery?
In case of photographs, which are captured imagery, those photographs are mostly associated with experiences and memories. So their lifespan will be much longer than a generated image. Because no one looks at a generated image and says, oh, remember those good times? That never happened, you know? So I think even the quantity of all those images or generated imagery may increase. Still, the quality and lifespan of the captured images will be around to actually have value.
If you go in the middle ground, which is generated, let's say, created imagery, which is art. But not generated by AI, that also has a value in the sense that all those artists are spending hours, days, and all that time to create that. And that makes them even more valuable. Because it is actually encapsulating the time, and effort, and energy, and wisdom, and style, and artistic flavor of that person. And in that sense that, again, quantity versus quality. They may be in future less than AI-generated imagery, but their value is still there.
Now, if we look at the core reason that we have generative AI, it's to mimic those two categories. Why does it become so beautiful or so valuable that it has the same lifespan with the original data? That, I don't know. And I hope not in close short-term. But I think provenance approaches will catch up at that time. Now there are all those controversies around generative AI stealing, in quotation marks, "stealing" artists' styles, et cetera.
At that time, I hope that provenance approaches will be so out there and so accessible that we'll be saying, oh, this is an image created in the style of this artist. Or this image is created based on this, the reference works of this. Or, when we actually created them, we captured a photo or an art piece that was created. There will be provenance approaches that shows the authenticity of that, which is tied to the value. So I'm hopeful that provenance will catch up by the time that it is actually that dystopian future, where all images are valueless.
KIMBERLY NEVALA: I'm giving myself a reputation here as a grumpy something or other. Which I'm not, but these are interesting rabbit holes, these flywheels. It's easy to go down when you start thinking about what the outside influences of this might be.
So you mentioned the concept of provenance. Let's talk a little bit about our ability today to understand or to identify when something has been, in fact, generated.
Now, you work in the field, and may have a heightened sense or awareness of what to look for. You mentioned earlier six fingers. We know that generated images of people, they don't do very well with hands. Because hands don't tend to be featured in a lot of the pictures and things that are out there in the corpus. Taking the point about ChatGPT not being generative per se in the strictest sense, there tends to be a cadence and a tenor to the text to that something like ChatGPT pushes out. And if you are conscious and aware of these things, you are likely to catch it.
But I would argue that those of us who are already well aware of the field or familiar with AI are better positioned today to detect those than folks that do not. I often pick on a cousin of mine who is still horrified that she can Google her name and all of the names of her siblings come up. She thinks this is horrific. And I can't decide if I'm a good or a bad cousin because I don't have the heart to tell her how much more is known about her that has very little to do with just the names of her siblings. And how that's being used in every aspect of her life, what she gets marketed to, et cetera, et cetera.
So there is sometimes, I think, a bit of a naivete in the broader public. So what is the sort of state of the art today in our ability to detect, to consistently label and make people aware of what is and is not generated?
ILKE DEMIR: Right. I will start with the subset of deepfakes, and then go up from there.
KIMBERLY NEVALA: Great.
ILKE DEMIR: So for deepfakes, people have been looking at boundary artifacts or symmetry artifacts: having that feeling, "Oh, something is wrong with that video, but what?" But generative models, generative AI, have got even better than that. So now we tend to demand some AI-based approaches to guide user understanding. And for that, my collaborators and my team at Intel developed the first real-time deepfake detection platform, which gives real-time information about something is fake or not.
So imagine just like we have this audio with video. And there's a little label saying that "Oh, we think by 99% that this is a fake video. This is a real video." And the way that we are doing it is pretty cool for now.
KIMBERLY NEVALA: Tell us more. Tell us more.
ILKE DEMIR: Yeah, I know. We are actually looking at the heart rate.
So when your heart pumps blood, it goes through your veins, and the veins' oxygen content changes. Because of that change, the color changes. And of course, when I look at a video, I cannot see that color change, but that color change is computationally visible. And that is called photoplethysmography: PPG for short.
So we take those PPG signals from many places on the face. And we look whether your left cheek and right cheek have the same PPG signals. Or if their timing and periodicity is the same, et cetera. So we take all those spatial temporal correlations of those PPG signals. And we train a deep neural network on top of that to classify each video to real and fake videos.
And this gives us 96% accuracy on the face forensics data set. We also evaluate it on many different data sets: in the wild settings, and cross correlations, and cross model experiments, et cetera. They can look for FakeCatcher, and the FakeCatcher is the algorithm that I just explained. So the FakeCatcher paper explains the whole experiment.
But what I want to mention is that this is also a direction towards informing the user about more signals and what we are doing. So, FakeCatcher is not the only detection algorithm that we have. We, for example, have an eye gaze-based approach. Where we are looking whether they are looking at the point or they have googly eyes.
So we want to inform everyone, saying that, oh, according to the heart rate, it is, let's say, 96% fake. According to the eye gaze, it is 92% fake. But if you look at the motion, for example - motion is another detector - if you look at the motion, it is 100% fake, et cetera. So the more reasons that we give about the detection, the more percentages that we are transparent about, the more that we make those models interpretable, the more we are opening the eyes of people for those generative AI models.
Now, this is in the realm of deepfakes. If we go into a little superset of the generative AI outputs, of course, there is text, there's 3D, there's images, et cetera. So in all of those, we want to develop detection approaches that are looking at priors. Structurally, a hand is an example, right? If we detect hands on all imagery and if they have five fingers, maybe we say, yes, this is real imagery. I mean, it's not that easy. But having all of these detection models fine-tuned, per domain fine-tuned, per modality, et cetera, is very important.
There's also one more thing that is important. So you cannot just develop a detector and put it out with unclear indications. So when we say, 90%, that is based on the model accuracy and on the data set that we document. But if you say, unlikely to be generated, how much unlikely? What does unlikely mean? Is it generated? Is it not generated? Because of those vague terms there is a little bit lack of transparency. I just saw there's a ChatGPT detector that detects something as written by ChatGPT. Sorry, likely to be written by ChatGPT. Not absolutely. So of course, we need to develop accurate models, but we also need to be transparent about where that result comes from, or how much that result comes from.
KIMBERLY NEVALA: I suppose this begs a couple of questions.
First is that, for my poor, unwitting cousin out there, who may not even think to ask the question: is this actually a video of Kimberly or a deepfake of Kimberly? Now, I probably have googly eyes naturally and will probably come up as fake as a result, because I flail about a lot when I speak. But it may not occur to her to even ask the question. So there's some aspect of this, which is incumbent on us as the creators of these systems to be proactively pushing out those pieces.
ILKE DEMIR: Exactly, yes.
KIMBERLY NEVALA: But you also allude to a different point. Which is most of us probably are not quite as skeptical as I am and that's probably a good thing.
But let’s say we can always watermark these things in some way. And the associated metrics are always available. You mentioned the OpenAI detector which may identify text as ‘likely’ to have been generated by an AI system. And if you see that label, you may think this is great! Now I know…
But In January, it’s true positive rate – the ability to correctly identify AI-written text - was only 26% on a challenge testing set. And it had a 9% false positive rate. While 26% doesn’t inspire immediate confidence, 9% doesn’t sound so bad. But if you are one of the 9% of college students who are accused of cheating when you are just a mediocre human author, the implications could be profound. At the potential scale of use being projected, 9% could impact a huge number of people.
And I’m not sure the general public necessarily has the statistical know-how, or literacy is probably a better word to know how, to your point, to interpret those statistics. So, do we also need to really lean into better education and training? Not just for those of us in the field but for everyone that is going to run into these things, whether they know it or not?
ILKE DEMIR: Absolutely. And I think that literacy starts with awareness, as you said, for your cousin.
Because your cousin may not even know deepfakes exist. So a part of my team is dedicated to deriving trust metrics. And those trust metrics, of course, encapsulate technical trust metrics that I mentioned before. But also completely human-based trust metrics about what is the general perception, human perception, for that kind of output. It might be deepfakes. It might be generative AI output, et cetera.
And what is more trustworthy? How do we even formulate trust? These are very hard questions that we are asking. We are running some user studies as of right now, actually, about how we can pinpoint exactly how we convey the message. How we understand their perception. How we help them with their perception, instead of guiding or enforcing our perception of something about it.
There are all these ethical AI principles, like fairness, transparency, accountability, et cetera. And we are trying to use these pillars, both for detection and how we communicate detection, and how we do responsible generation over all the projects within the responsible AI framework.
KIMBERLY NEVALA: Earlier in the conversation you also mentioned the evolution of things like data provenance. What other types of proactive mechanisms do you think we, either as an industry or as companies pushing these solutions, should be looking at? If we start to look out, what is in the works now? And what can we expect in the future?
ILKE DEMIR: So we are very lucky that we are actually in a very responsible tech industry. There is already a coalition called C2PA, which is Coalition for Content Protection Authentication. This is a coalition from many industry leaders like Intel, and Microsoft, and Adobe, and Truepic, et cetera. These technical leaders are putting all their brains together about exactly how to solve what you just asked. How can we create open technical standards? I want to emphasize open, by the way. Anyway, open technical standards about provenance approaches. And how we can bring those provenance approaches onto all the media creation devices that we have? All the way from cameras to generative AI to traditional editing software, et cetera. And how we can do that in a secure way such that some camera-based watermarking solution is not replicated by generative AI, for example?
So you can open and read C2PA documentations and technical standards. My team is also supporting how we can actually implement those standards, especially for generative AI. How can we merge authentication and generation in one output? How can we make it more secure? In the sense that instead of those old certification and client-server relations, can we actually embed things into the media so that we just read the provenance information from the media itself?
I keep saying media, but again, it can be images, videos, 3D models, voice, et cetera. So that's why I'm saying media, or content, or data. So I'm very hopeful that all the provenance approaches, technical standards, and all the implementations with these very diverse set of companies will actually bring the provenance up to par with certain models.
KIMBERLY NEVALA: And that gets us out of this self-propelling flywheel, potentially, because we're not there yet. These methods don't necessarily exist, but it's really good to hear that folks are actively working on them and looking for them. And I'm sure regulation will come. Although, it will come behind, as it always does.
Because as you were speaking about -- I think you've called the fake detection the watermark of being human, right? All these minute imperfections, or the fact that my heart rate's going up and I’m getting flushed as I ask questions and get excited about what you're saying. But again, as that happens, we then have systems who know. We now know that you're looking for this differential in the flush in my cheeks or looking for the way my eyes move. And you can start now to use that to generate better images that replicate that or no?
ILKE DEMIR: Not necessarily, no.
KIMBERLY NEVALA: That's not a slippery slope I should be worried about?
ILKE DEMIR: For some of the approaches, you are right. Because they are doing, in layman's terms, simple things.
But, for example, for heart rate, for PPG signals, the way that we are extracting PPG signals is not differentiable. So you cannot just plug it into a generative AI. You cannot plug it into a GAN and try to learn that PPG or learn to generate that PPG signal.
If you don't want to use the exact extraction of PPG signal, but you want to approximate it somehow. Well, then you need a huge data set of ground truth PPG signals, which doesn't exist yet. And even if tomorrow comes and a hospital says that, OK, we are opening our thousands of people's PPG signals. Then even in that case, we can turn the PPG signal extraction locations to a probabilistic one all over all the face. Instead of just those known locations. In that case, the generative model needs to create consistent in time, consistent in frequency, and consistent in spatial locations constant PPG signals all over the face, instead of just those places.
And this is a very hard problem because PPG signals are very subtle, very under the skin - no pun intended. So to create very consistent PPG signals all over the face is a very hard problem. I cannot say it is impossible, of course. But for now, it remains as a very hard problem that people will create deepfakes with nice, good quality PPG signals.
It may not be the case for other things. For example, if you are just looking at gaze, gaze is just two vectors, two rays, light rays that you are shooting from your eyes.
KIMBERLY NEVALA: I'm not looking at her meanly, by the way.
[LAUGHING]
ILKE DEMIR: Hi, Cyclops.
KIMBERLY NEVALA: Sorry. Anyway, you were saying…
ILKE DEMIR: So when you shoot rays from your eyes, they actually converge on a point. And it's just the intersection of these two vectors. So if you are just formulating gaze, actually that formulation is differentiable. So you can plug it into a loss function. And I think a company who is leading the generative AI space already did that for Zoom meetings. So that you actually have consistent eyes that are not looking at the screen, but to the camera. So that is something that can be learned.
But for PPG, it's not yet that easily done. And for our other upcoming detectors, too. For motion for example. Motion is a very complex thing. In all of the generative model outputs that we looked at motion is not preserved as much as real motion in most of the cases.
So we are bringing up all those different detectors together so that they are complementary to each other. They are giving more information to the user and they are looking at all the different signals that are possible.
KIMBERLY NEVALA: Wow. I find myself highly reassured by that. And I had to chuckle when you said about the laser eyes there because you're right. The demos I've seen of that are still a little, they're a little unsettling. I might not know why until I know I'm looking at it, but they still don't move the same way eyes do: even though they're looking directly at the camera. I guess none of us really look that directly at a camera for that long.
ILKE DEMIR: Exactly.
KIMBERLY NEVALA: So this maybe goes back to that, you've talked about this, the watermark of being human. It's all those beautiful imperfections that make us perfect. But, also, ultimately, even if I visually can't look at something and know for sure; I might get a feeling in the pit of my stomach that says, that looks a little off. But we can use the same machines that generated these things to actually synthesize all of those signals, and then help us.
But it does sound like this is ultimately going to be all about bringing a variety of techniques and approaches. Both manual and offline, our own literacy and awareness. And getting to the point where we're actually pushing out and requiring things like watermarks and disclosure, when these tools are being used.
You are an inveterate optimist and I love that about you. So what are some of the types of applications or capabilities that you're most excited about and that you most anticipate in this field as you look forward?
ILKE DEMIR: Technically speaking - I think everyone may say that in the generative space - but Stable Diffusion and all those diffusion models that are creating more modalities. And how it is going super-fast is what I like.
So if we go a couple of years back, when deep learning was becoming deep learning. Like, oh, neural networks. I mean, if you go really back, it started very early 1980s maybe. I don't know. But when we see ImageNet, et cetera, most of those analysis techniques for recognition, classification, et cetera, got a little bit more stuck onto the modality. So everything was about images. All deep learning approaches were about images. And to extend it to maybe 3D, or maybe voice, and other modalities was a little bit lacking.
Now, with the current way that the generative models are going - generative AI are going - sorry, because generative models have been there for many years. But for generative AI, it's going in just under maybe one month, maybe two months, we saw 3D generation, text to 3D. And I think one month after that - I think it was in January - I saw that text to 3D video is already out there. So 3D plus 1. Maybe it's 4D. Maybe it is open to discussion. But 3D plus temporal dimension, which is 3D videos, are already there for text to 3D videos.
This pace is what I'm really amazed by because that was not the case. And that shows that it's not just niche population of researchers working on this topic, because all of these tools are very democratized. Everyone is actually working in that topic. You open Reddit, and, oh, see today, there is that very new diffusion model that is trained on that very specific data set.
I heard, for example, some colleagues are working on tattoo generation as a generative AI. They don't want to design tattoos anymore. They just want to use generative AI to design tattoos. And they can then tattoo people. All those niche domains that are not just coming from us researchers but from the public is actually making me very excited.
KIMBERLY NEVALA: Do you think we're spending the requisite equal amount of time on ensuring that we are putting in place the right guardrails? Your team does, you guys work on techniques for using this responsibly or helping to ensure other people are. But in general, as industry, there's certainly a lot of talk about responsible AI, responsible tech, responsible innovation. Are we spending enough time on this side of it? Or is it getting away from us?
ILKE DEMIR: Unfortunately, I don't think we are talking enough about that. I know that there are absolutely respectable researchers that have been talking about this for years now. Emily, Meredith, Timnit: all of them have been yelling about these a lot. But it cannot be just on five people's shoulders.
We all need to be open about talking responsible AI approaches, ethical AI approaches. And not only through the generation but also for consequences. Just generating something, putting it out in the world, and anyone can use it….No, you need to be accountable. Where was it used? Who is using it? What is it used for? I cannot just say, oh, I have a human body generator, a realistic human body generator, and OK, I open source it and everyone can use it. You cannot do that. People will use it for something else.
So yeah, I think we need to spend more time and more energy talking about all of these aspects about consequences, about accountability, about transparency. And it should not be an afterthought. It should be where you start the conversation, but it should not be, OK, I have this very beautiful thing. How can I make it responsible? Nuh-uh, that's not how we go. At least in my team, that's not how we go. We actually, first, see the problem, see where it can…what does the evilness come from? And then solve it by design. Solve it by how we enforce the system to go through that obstacle.
Because those pillars, those ethical and responsible AI pillars are not something to clean up. They are actually the obstacles that you need to go through. They are a requirement of the system. They are not good to have. So if we can make that perspective everyone's perspective, then we wouldn't be having this conversation. So maybe I'm glad, though. That was a joke. Sorry.
KIMBERLY NEVALA: Wow. As I've demonstrated, I can always find other weird things to worry about and have you right back on so you can balance my - I don't want to say pessimism, but sometimes it probably comes off like that - with your optimism.
I think that's a great call to arms for all of us and for the industry that we work in to leave things with.
ILKE DEMIR: By the way, I wouldn't call it pessimism. I would call it realism, because the questions you ask are the questions that we should ask. They are not pessimistic questions. They are realistic questions.
KIMBERLY NEVALA: I'm going to take that clip and carry it with me when people call me a pessimist. So thank you. I appreciate it. I appreciate that validation as well.
I love the way you're able to help us understand the depths of generative AI. I'm both equally reassured and concerned, more reassured and more concerned after the discussion. And in somewhat equal measure, but a little bit more reassured, that folks like you and your team are working on these problems. And that seems about right for the current state of affairs.
So thank you so much, Ilke. I've really appreciated it.
ILKE DEMIR: Thank you. It was a very nice conversation. and I think it's not normally as organic. So I'm very happy that you provide this friendly, warm, safe environment with right questions to ask.
KIMBERLY NEVALA: Oh, excellent! I hope that means we'll be able to entice you back some time here in the future.
ILKE DEMIR: Sure.
KIMBERLY NEVALA: All right. Next up, Reid Blackman, who is the author of Ethical Machines, is going to join us to debunk common myths about ethical AI. And we're going to talk about the line we need to draw between advocacy and fanaticism when we put AI ethics into practice. Subscribe now so you don't miss it.
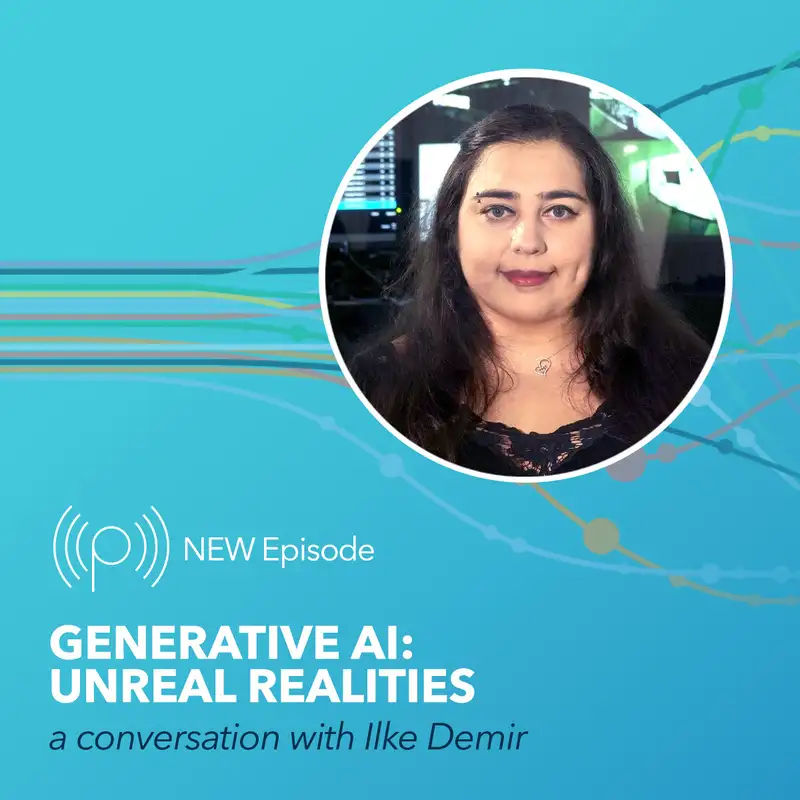