Keeping Science in Data Science with Patrick Hall
KIMBERLY NEVALA: Welcome to Pondering AI. My name is Kimberly Nevala, and I'm a strategic advisor at SAS. This season, I am joined by a diverse group of thinkers and doers to explore how we can all create meaningful human experiences and make mindful decisions in the age of AI.
Today, I'm beyond pleased to bring you Patrick Hall. Patrick is the principal scientist at BNH.ai, and he joins us to discuss science and law in the age of AI. Welcome, Patrick.
PATRICK HALL: Thanks for having me. I'm excited to be here.
KIMBERLY NEVALA: So let's start with -- you and I used to be colleagues and did a lot of work in the early days about helping folks understand the basics of artificial intelligence and machine learning. But our audience may not be familiar. So tell us a little bit about your background and the mission of BNH.ai.
PATRICK HALL: Sure. So I should start off by saying I'm not a lawyer, that should be the first thing I say.
So I'm not a lawyer. I have a technical background. My education was in math and chemistry and statistics. And I worked at SAS. It was lovely, it was a lovely four years. And what I did there was really just basic machine learning. Making machine learning tools for people to use. From there, I transitioned to a software company called H2O.ai where I was lucky enough to work with another group of very talented individuals, and we ended up bringing one of the first products for explainable machine learning and with some bias testing for machine learning systems to market there, and that was very exciting.
And one thing I learned in that whole process is that if you want to use machine learning in these high-impact areas, which is that's typically where we want to use machine learning, that's where the biggest gains are, you're going to have a lot of legal considerations. And I was so struck by that and so struck by how it seemed like almost no one was aware of this outside of a few highly regulated spaces such as in employment and in consumer finance that my business partner and I, Andrew Burt, who is an attorney, who had experienced the same phenomenon but from being the attorney that was constantly breaking data scientists' hearts …Because it's always at the very last stage of a data science project that someone thinks, hey, maybe this could have some legal problems, and they often do. And then it's often up to some poor attorney who got this draft on their desk and doesn't really understand much of what's going on to say, hey, this is clearly problematic and we have to stop.
So I've been on the data science side of that, Andrew had been on the legal side of that, and we got together and started this firm, BNH.ai.
KIMBERLY NEVALA: This is awesome. And today I want to talk to you a little bit on both sides of that divide. Both the data science and the legal side - I shouldn't really call them a divide, I don't know that that's the right analogy.
PATRICK HALL: Yeah, that's a good point.
KIMBERLY NEVALA: Shame on me, right? At the top of the discussion, no less.
I saw a recent article about AI and there was a mention which was attributed to the ethicist Giada Pistilli. And she said, “a worrisome trend is the growing willingness to make claims based on subjective impression instead of scientific proof.” Now a few months ago I reached out because you had written an article about the need to keep the scientific method alive in data science. So this comment and the subject has really struck a chord with me given the profusion of - let's call them highly suspect - capabilities being attributed to AI right now.
How does that resonate with you? And why is this a valid concern and important point of discussion?
PATRICK HALL: Well, it really resonates with me. And we've been lucky enough to work with NIST, National Institutes for Standards and Technology, in the time and the two years since we started BNH.
And one of the things that we learned both working on the bias standard and the broader AI risk management framework is the massive role that bias plays. Both systemic biases against minorities, against women, against any number of groups of people. And really, any group of people can experience discrimination, but we all know who tends to experience it the most, both in the real world and online.
Those types of systemic biases, mixed together with a really nasty set of human biases, like our tendency to anchor our thoughts to a number that we've seen before. Something called the McNamara fallacy, which I find endlessly fascinating: named after or the famous government official and businessman McNamara who put forward all these ideas about automation and algorithms, who collected data that are mostly wrong, honestly, and we've just acted like they're right for decades.
And then that combined with just statistical and modeling biases really creates this pernicious mix of both human structural and mathematical problems that lead to AI systems just not working. And so on my side, there was a recent paper coming out of FAT ML Conference (the Fairness Accountability and Transparency and Machine Learning conference) that highlighted just fundamentally how many AI systems will not and cannot work because they're poisoned by these kinds of biases and many other issues.
And so where the scientific method comes in here is we've known for centuries, really, that these types of biases are problems, and if you don't address them, they'll spoil your results. And I just see that reality woefully lacking in the practice of data science.
KIMBERLY NEVALA: And for folks that are not familiar with it - the McNamara fallacy - what are some of the precepts that folks have maybe taken for granted that have been proven wrong but still underpin a lot of the way that we approach data science and AI today?
PATRICK HALL: So basically, the McNamara fallacy says that algorithmic automated decision-making and data, whether analog data written on paper or digital data, is essentially better than other kinds of decision-making and other kinds of information. And there's just not much evidence to support that.
I mean, unfortunately, humans are not great at making decisions either. Humans are not great at being objective either. But all we're doing with computers, usually, is taking our own biases and automating them at scale. And so of course, that doesn't really address any of the problems.
And so I think the McNamara fallacy allows us to act in biased ways faster and broader scale than we used to be able to and say, look, we ate our vegetables, we took our vitamins, we did machine learning. We did data-driven decision-making when there's really not much evidence, especially in social contexts, that machine learning does any better than simple models or, sadly, even biased humans. So especially in social contexts, the evidence is really that AI and machine learning systems don't do better than simple models. And so I point to that as one primary place where we're falling back on the McNamara fallacy and not the scientific method.
KIMBERLY NEVALA: And something else you've referred to in the past, which I think is important and helps people think about this issue: there's a lot of discussion about the lack of comprehension in machine learning systems or AI systems broadly. Which can show up in systems that emulate or mimic human responses, often very well, sometimes really convincingly. But they're not aware of context. They're not behaving in an intentional way. And I thought you maybe said it best when you said machine learning learns about data.
PATRICK HALL: Yeah.
KIMBERLY NEVALA: It doesn't learn about people or their behavior. Talk a little more about that, and again, why is that so important for everyone to keep in mind relative to these systems?
PATRICK HALL: Sure. And thank you for bringing that up. And again, it ties in with the McNamara fallacy.
Machine learning systems only learn about people or companies or whatever it is to the extent that those things are accurately represented in data. And that's because machine learning learns about data.
Machine learning is not like a child. It's not like a person. It doesn't have eyes and ears and the nose and a mouth. It's not it's not kind of constantly looking around and understanding the effects of gravity or if I touch this hot thing, it hurts. It is really limited to learning from digital data sources.
And so of course, there's been this big controversy, which I had to run my mouth about on social media, where a Google engineer - and a very accomplished engineer, and an author of one of my favorite machine learning papers, actually - has become convinced that a machine learning system is conscious.
And the conventional wisdom is, this is just not possible. There's no, getting back to the scientific method, there's no concept validity in going from learning from digital to data to being conscious. We have to have a scientific hypothesis that would explain how learning from digital data would give rise to consciousness.
And we don't have that. No one can explain to me how learning to predict the next word in massive amounts of digital data could give rise to consciousness. And so that-- I don't want to go on too much here - but I think that that is a really good example of the lack of the scientific method and data science.
You have to have construct validity. You have to have a real hypothesis that is verifiable to do science, otherwise it's just magic. Anybody can say this machine feels alive, and they're free to say that. But it's not science unless there's a verifiable hypothesis that has been proven that makes sense that bridges this gap from learning from databases to becoming conscious. And so I think that kind of magical thinking is oftentimes behind a lot of what we may see successes in data science and AI today.
KIMBERLY NEVALA: Yeah, and I think the tendency, the beauty in big data, is we can find a lot of patterns. There's a lot of insights. But someone said to me recently, patterns aren't people. And what's valid - again, back to basic statistical principles - in the aggregate, cannot, then, be localized to what a single person, single human, or even sometimes what a system will do next at the level of a single action or incident.
And there's also a lot of what I'm going to call, for lack of a better term, statistical coincidences. And sometimes we see what we want to see. One area I've noticed this really coming to roost is this emerging - I don't know if I should call it a topic or an application - that people like to call affective or emotive AI.
And for folks who listen to this podcast, they know that I am on a perpetual rant about the fact that just because I tilt my head and flail around a lot, it does not mean that I'm depressed. But there has been this multitude of systems that purport to do things like determining someone's potential - work potential is - from their posture. Or if a child is engaged in the classroom and going to be successful based on their basic body language. Or what my personality is from that body language. I think one of the more recent ones was getting your mood or your emotional state from your facial expression. And in most cases, the scientific basis for this is dubious at best. I don't want to make any aspirations about people's good intent or lack thereof but this seems to be another area where we can look at a lot of things in data and find some correlations, if we look hard enough, to tell us something that really isn't telling us anything and leading us down a path and it looks magical.
So I know you did some work-- or have been involved with some work with NIST on affective computing research. Is this another area where the magic of big data might be leading us down illusionary, if not outright dangerous paths because of this lack of grounding in science, if you will?
PATRICK HALL: Yeah, yeah. I think so. And so not to say that this is never possible. And not to say that machines being conscious is never possible. And not to say that one day in 100 years we won't be able to have deep insights from people's faces or something like this. I don't think so, I think it's fundamentally dubious. But also I think it's also important to leave open the possibility that this hypothesis could one day be proven true just to be objective. And so that's why I say it that way.
But in the work with NIST, a just really exhaustive literature review shows that psychologists do agree that your face can portray your emotional state. But, that varies wildly across cultures, across ages, across demographic groups, across the same person at different times of day.
And so there's no…my understanding is psychologists might agree that your face can portray emotion, but not in some kind of way that can be learned by a computer systematically. And so we call this notion out pretty strongly in fact, in a callout box in the NIST SP 1270 Bias Guidance. And say essentially that today it is mostly pseudoscience. It is mostly pseudoscience.
And, again, this gets back to this point I was making earlier. AI, machine learning, what they're good at is understanding digital data. They're good at predicting when machines will fail based on digital signals. They're good at things that can be represented well by digital data. They are not good at making decisions about social outcomes.
And being in DC, I know that public policy professionals are becoming very aware of this. So it's more having the general public get this understanding that just because a company says, if you play a game on your phone, you'll be a good employee, it's really the McNamara fallacy that leads us to believe that that's true.
There's not much scientific evidence, there's no construct validity. There's no common sense way to go from a video on your phone today tells whether you will be a good employee in 10 years. It's just magical thinking to go from video today to good employee in the future. And AI is not magic.
I think a lot of my sentiments can be boiled down to that: it's not magic. And when there's success, it's either because people have followed the scientific method diligently for a long time or they got lucky. And I think we see both happening sometimes.
KIMBERLY NEVALA: So with that in mind, then, what does it look like if you are participating on a team being asked to or trying to develop an AI-driven system - maybe some machine learning algorithm? Are there basic steps or guidance you can provide to them to ensure the actions or the decisions they are basing on these AI system outputs are, in fact, on solid statistical and scientific ground?
PATRICK HALL: Yes.
So this sounds weird to say, but what the internet calls data science is not science as far as I can tell. So one, this idea that we take whatever data is available, whatever observational data. Statisticians have understood it for a very long time, that drawing conclusions from observational data is very, very fraught, like you brought up earlier. So this idea that we take whatever observational data is available, pour it into a black box model that we don't really understand how it works. And then, essentially in that same data set, look at some basic accuracy statistics and decide that the system works, that's just not science.
There's well-known notions with drawing conclusions on observational data like I said. The hypothesis that we're trying to form is not verifiable because of our use of a black box model. And then the test that we do is not a strong test. Like deciding that an overfit algorithm looks good in one sample of data drawn from a big data set and another sample of data drawn from a big data set. That's not really testing your hypothesis.
So we really have to shift away from this like Medium and-- there's a really funny video about a fictitious Python programmer who goes from, I just learned this on Medium to do you want to write a Medium? And so like we have to move away from this Medium concept of what data science is and go back to the scientific method. Which would say: write down a hypothesis and don't change it to make your results look better. And that's mostly what we do in machine learning as far as I can tell.
So write down a hypothesis. I think that hypothesis should be about the effect, the intended effect, of your model in the real world. Not about, is a neural network better than a random forest or something like that. So write down your hypothesis, have that hypothesis be about the intended effect of the model in the real world.
Use a transparent model, which may not be possible for some NLP-- Natural Language Processing or computer vision processes. But for structured data, there are many types of machine learning models that are very transparent that perform just as well as so-called black box models. So use a transparent model so that you can understand if the model has any construct validity.
And then test, try to do an actual test, like an A/B test of whether your model is delivering the intended effect in the real world or not.
So it's really a complete shift from dumping bad data into a black box model and pretending it works to actually practicing science. It's a very big shift but I expect that as companies and other organizations become more serious about their AI systems returning value, that we will see a shift to this.
Because getting back to this notion of systems that cannot work, it's very likely that if you just pour bad data into a black box, it can never work. It's just money and time down the drain. And so I think as companies become more and more demanding, that all this money they spend on data science actually provides return on investment, we'll see a shift to people actually trying to do science.
KIMBERLY NEVALA: Yeah. And there certainly - I mean, obviously in the press we see lots of outcry against biased models. Or just models that don't affect change in the way they were intended out in the wild in the public sphere, but also even in the more corporate sphere. Certainly we've also seen a lot of recent research that says, for all of the discussion of AI and its benefits - and here, I'm talking about it as an umbrella term (so machine learning, deep learning, NLP, natural language processing, or computer vision) that still very few companies are really deploying this for good effect.
I've started to wonder if in some cases, because of all these issues - and it can sound very overwhelming, - that AI might be simultaneously overhyped and undervalued for a lot of organizations. By which we're expecting both too much (or some magical outcomes) from these systems and we're not seeing them but we're also missing some germane opportunities to drive value with AI for what might be seen as much more mundane applications. Very back office operational problems where, as you said, the type of data and the nature of these systems actually comes together very well but it's not sexy, it's not fun.
So in your experience, are organization's leaving value on the table with AI? And how can they address these kinds of opportunities?
PATRICK HALL: Well, I think you said it correctly for the most part.
KIMBERLY NEVALA: Well, that's good [LAUGHING].
PATRICK HALL: Yeah. So I teach about these topics, too, and one thing that I use my teaching a lot: I sit on the Board of the AI Incident Database - just being transparent in case there's any conflict here. So I use these AI systems failures, which there's thousands of reports, public reports of AI system failures. And if you haven't seen the AI Incident Database, it's good reading: it's anything from hilarious to horrifying.
So a lot of times my students say, hey, you spent all semester telling us how these systems failed. How do they work? So again, getting back to this theme of there's no magic, if you're being really ambitious with AI, then you need to be really ambitious with your spending and the resources that you plan to put behind it and the time that you plan to spend on it.
Why does Google search work so well? Why do certain military applications of AI work so well? Why does AI work fairly well in consumer finance? Because all of these organizations have spent billions of dollars over years with literally the best people in the world to make these systems work.
So I think you're right, that many organizations could take a much more commonsense brass tacks approach to AI machine learning to get much better results. How can I optimize back-office data-driven procedures with machine learning? That's where machine learning really excels if you don't want to spend billions of over decades, so I think you're right about that.
And if you do want to be ambitious with AI, you have to open up your wallet and you have to wait a long time for it to bake. And that's simply because there's no magic, there's no magic. And if you look at the organizations who were ambitious about AI and successful, they spent billions over decades.
KIMBERLY NEVALA: Interesting. Which I'm not taking to mean that you're telling smaller organizations or the non-digital natives that AI is out of their wheelhouse. But again, that they need to, A, make mindful investments and be prepared to do that, but also target the technology at the areas that, in fact, they can impact.
PATRICK HALL: Yeah. And it may be important to unpack what we mean by AI. Some people mean these massive billion-dollar systems that were developed over decades. Some people mean a two-variable model that just takes the average of both variables.
And papers published in the proceedings of the National Academy of Science, what they will say is, if you're trying to make decisions based on social outcomes, about social outcomes, those two-variable models that just take the average of two variables, that's the way to go. And don't expect to be particularly right. Expect to be right about 20% of the time and maybe you'll just be a little bit less biased than we were in the past.
Machine learning also works really well on learning about data. So learning about how to clean data and things like this I think are great applications of true machine learning.
And then we have the very ambitious programs like you might see in the military or in banking and hedge funds or in Google search. Those are extremely ambitious programs that require extremely ambitious resources.
So I think there's all kinds of ways to build models. Humans have been building models for maybe millennia. Models are not guaranteed to be right, they're just a way to understand reality. There's all different ways to do it, and you should think about what kind of model will actually help your organization and not default to this internet conception of data science where we use bad data in the wrong models.
So that's how I would try to break it down. That's how I would try to break it down for smaller organizations that want to dip their toe in this. Be smart and be more clear about what you mean by AI and what you want to do with it. And try to tackle it in a way that actually makes sense and doesn't just buy into the hype around technology that essentially doesn't work.
KIMBERLY NEVALA: Yeah. And that mention of the National Academy of Sciences sparked another thought. A lot of our early AI discussions seem to be focused on people being very excited about AI as an autonomous agent or AI as the decision-maker. And even here, I'm using this language very poorly. ‘Using an AI system to make decisions’ is really what I should be saying as opposed to imbuing it with some human objectivity and agency.
But the conversation does seem to be shifting slightly away from this idea of AI systems as autonomous agents or even independent decision-makers - which I think is really positive. And is even going beyond human in the loop (who are just there to oversee the AI system) to the idea of human and AI teaming. What are we learning-- or have you seen - about how to best utilize AI as an assistive tool, as a decision-making engine, if you will? Or an input rather than an independent or autonomous agent?
PATRICK HALL: Sure. That's a great question. And I was recently invited to present at the National Academies. And while I really thought I might get struck by lightning or the building might burn down or something when I walked in, none of that happened.
One publication that the National Academies has just put out is about human-AI teaming. I really think that's where the smart money is right now. And that is very difficult. It’s incredibly difficult to make an AI system that makes better decisions than humans and that you should trust with your business: that's incredibly difficult. But it's still difficult just to make an AI system that works well with humans. But I think that is where you might get the biggest bang for your buck in terms of minimal investment, maximal payoff.
And really, this is aligned a lot with traditional analytics that companies like SAS have been working with for decades. We just want to balance out our human intuitions and our human experiences with data. Because, it turns out, neither are perfect ways to make decisions. But maybe working together, we might sort of balance each other out.
And so the National Academies did just recently put out a long paper - almost book - about human-AI teaming. And one of the biggest tricks there is how humans interpret results from computers. Which, if you talk to any psychologist or any user interaction user experience expert, that's a very tricky part of getting things right with even human-AI teaming: just all the different difficulties that humans have interpreting results from computer systems.
And there's lots of other gotchas too but just to stay positive, I would say, if I was going to invest big in, quote-unquote, "AI" right now, I would be looking more towards how do I augment my people with so-called AI systems? Versus how do I replace my people with so-called AI systems?
KIMBERLY NEVALA: Yeah, and there's some really fascinating research right now about, as you said, about how humans perceive inputs and outputs from machines. We had a chance to talk with Marisa Tschopp - she's a human-AI interaction researcher. Which was a fascinating conversation about our tendency to both distrust and overtrust machines. It's this catch-22 in all aspects. So for folks that haven't listened to it and are interested, I'd recommend giving that one a listen as well.
PATRICK HALL: Well and just really quickly, I'll say this is really just me being in DC, not of having much involvement with the military myself. But if you look at the programs in DARPA and the programs in the Air Force and the programs where they're really working on these mission-critical augmented decision-making technologies, psychologists play a huge role in those systems.
And we're talking about some of the most famous HCI (Human-Computer Interaction) psychologists in the world are playing a big role in these programs because it's just a huge part of it. A huge part of it is that human-computer interface. It's not my area of expertise, but really, really crucial to getting things right.
KIMBERLY NEVALA: So before we let you go, I'd like to flip back to the other side of this coin. You talked about being on both sides of the data science and the legal and risk management side of the house. And BNH.ai is obviously focused on helping clients really prepare and address legal and compliance issues to manage risk.
PATRICK HALL: Mm-hmm.
KIMBERLY NEVALA: What are the trends that you're seeing with companies? And are companies doing enough today to be ready for both the existential and legal risk and legal environment that's developing here?
PATRICK HALL: What I've observed is it's really only the largest organizations, both in terms of commercial organizations and government agencies, that are starting to come to terms with risk management around machine learning. And I think that just points to where we actually are in the adoption cycle.
Everybody can say they're doing machine learning. I'm sure in many cases they are. But if you really want to base your business on it and you really want to be successful with it, you're going to manage risk around it just like you do with every other important piece of technology.
And so what we see right now is I think it's really the companies with the deep pockets, with the armies of PhDs, with the long-running experience in technology, that are starting to take action on risk management around AI. We do work with some smaller companies, particularly smaller companies that are working in extremely high-impact areas. And it's great to see that as well.
And so I'd say in the end, it's kind of a mix between the usual subjects, big companies and big government organizations who have been at this for a long time, and then smaller companies who are working in really high-impact areas. These are the companies that are reaching out to us to get help with-- to get help with risk management.
But again, I'd like to emphasize the statement that if a technology is important to an organization, they do risk management around it. And so I think if you're proclaiming that AI or machine learning is important to your organization and yet you're not managing the very significant risk around such a new and dynamic technology, you might want to think about doing some risk management around it.
KIMBERLY NEVALA: Yeah, we did a global study called AI Momentum and Acceleration. And it was a comparative back to a study we did back in 2018 on AI adoption and maturity.
And one of the findings that was frankly, for me, somewhat terrifying was that I think probably 73% or something like that - so a huge majority of people - said they trusted AI-enabled systems to make decisions for them. At the same time, about 50% said that risk and concern about both ethics and just basic risk management were a constraint and that issues like trust kept them from moving forward. So there was a little bit of a dichotomy there.
But of those folks who said they trusted AI systems to make decisions, a minuscule amount - I think it was less than 30% - said that they had ever actually had to rethink redesign or change an AI system when it was in practice. And I'm not sure if that's because of the nature and size of what those, quote-unquote, "AI systems" really are. Or because we're not finding these problems because we're just not looking for them.
But in any case, knowing the nature of these systems and the fact that they're probabilistic and non-deterministic. And they change with time and they do error in rather significant ways and can go rogue in the wild so fast - not the least of which is due to factors like what you talked about before - which is not doing really good real-life verification and validation and making sure that the data you've trained something on is actually reflective of the real world, and it's always not going to be exact, but it's good enough for the situation.
It was a confounding and, as I said, slightly concerning finding. And it may be due to just we're at the really early stages of adoption and the types of use cases, but I also wonder if it's people not just being mindful enough about what the actual risks might be here.
PATRICK HALL: One thing that I like to fall back on when trying to sort through these issues is, I think-- and people, other people have said this, too. I'm not sure exactly who to give credit to here. In 99.9% of cases, machine learning is just software. And I'm sure there are instances of machine learning that are embedded in firmware and things like this, but just broadly speaking, machine learning is software.
And so, it's just mind-boggling that some kind of software that a big enterprise or big government agency was going to use to make serious decisions, that would go through years of testing and people would have all kinds of documentation and best practices and be following NIST frameworks and SANS Institute frameworks and have armies of product managers and testers.
And then it's, oh, over in the corner we have this group of data scientists, this group of brilliant data scientists, they're doing AI and machine learning. Just let them do whatever they want to do and deploy it as fast as possible.
That dichotomy is just purely driven by hype, and if you think of AI as software or spreadsheets, it's a lot easier to be sane about it. And so I think that's another: no magic, it's just software. Treat it like other mission-critical software that you use and you'll be in much better shape.
KIMBERLY NEVALA: Yeah, that's such a good point. And I guess back in the day we always thought of analytics systems, the data warehouse, it was separate from the operational systems - they were on their own little island. And I wonder how much of that historical thinking also is impacting us when we're not thinking about these AI-enabled systems as, in fact, operational business processes. So great point.
PATRICK HALL: Past is prologue.
KIMBERLY NEVALA: That's right. The work you're doing is going to be increasingly important and, as you said, you can't divorce the legal environment from the operational environment and the data science environment. Any (final) words of wisdom or lessons learned you would leave with the audience?
PATRICK HALL: Well, of course I think the legal aspect is very important.
And probably, I think the reason I was drawn to it is because if you're a big corporation or big government agency, I would say that your biggest risk is safety. You could deploy these systems, and they do kill and hurt people today. They've already killed and hurt many people.
So I would say, we need to reframe from this notion of, get bad data, train a model, draw another sample of that same bad data, and decide that the model works on that and we have a good model.
We need to change away from that mindset to a real product mindset in which is safety is most important, then probably legality, and of course, safety and legality are tied together. But as an example of them not being, when an Uber ran over someone in 2018, for reasons I can't explain, Uber had no criminal liability there. All the criminal liability was placed on what was very likely a contract employee safety driver who had very likely been told that nothing could go wrong.
But we need to refrain from the silly notion of what data science is to thinking first about safety, then about legality, then about real-world performance, and then back to this notion of, how do I do on test data? So it's like how you do on test data is perhaps the fourth most important thing you should be thinking about.
The number one most important thing you should be thinking about is safety; number two, legality; number three, does it work right in the real world? Number four, did it work right in the lab? So I'll leave us on that note.
KIMBERLY NEVALA: Wise words indeed. Well thank you, Patrick, for shining what is a very timely light on the importance of both science and critical thinking in the application of AI. Not to mention the implications of these rapidly evolving regulations and legal implications Thank you so much.
PATRICK HALL: You're very welcome. My pleasure to be here.
KIMBERLY NEVALA: Excellent.
Next up, we are going to be joined by Mark Coeckelbergh to discuss the political underpinnings of AI and how political philosophy may help us better understand AI's influence on society today. Subscribe now so you don't miss it.
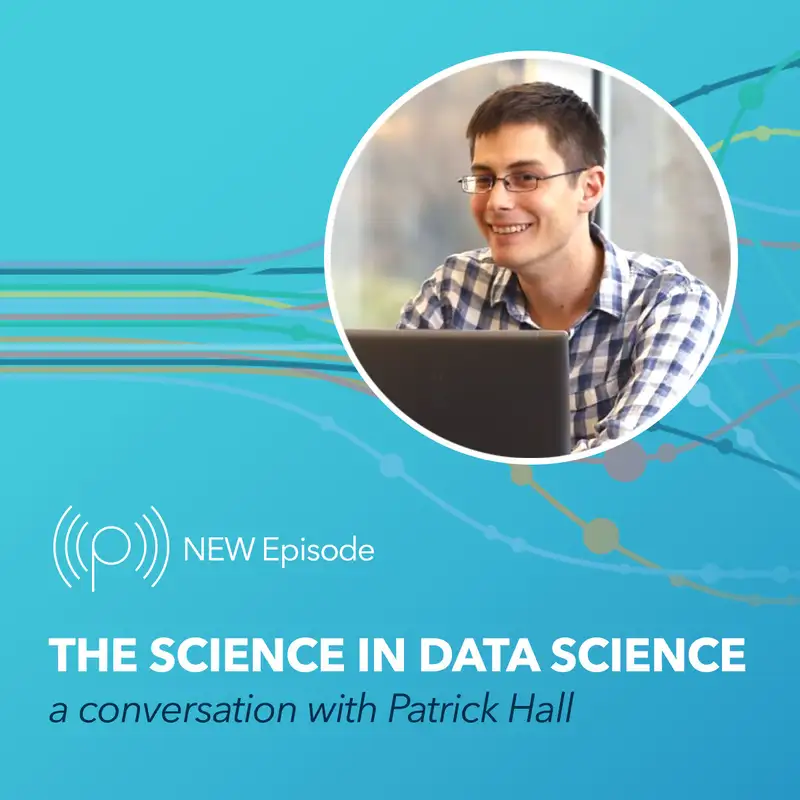