AI’s World View with Dr. Erica Thompson
KIMBERLY NEVALA: Welcome to Pondering AI. My name is Kimberly Nevala, and I'm a Strategic Advisor at SAS. This season, I'm so excited to be joined by a diverse group of thinkers and doers to explore how we can all create meaningful human experiences and make mindful decisions in the age of AI.
In this episode, we welcome Dr. Erica Thompson. Erica is a Senior Fellow in Ethics of Modeling and Simulation at LSE's Data Science Institute. She also holds fellowships in several other prestigious programs. Erica is passionate about the realistic evaluation of model-derived information. And, as you're going to hear, she is a master at clearly explaining the issues at hand here. Welcome, Erica.
ERICA THOMPSON: Hi. Thank you, Kimberly. It's great to be here. Thanks for having me.
KIMBERLY NEVALA: Absolutely. So I'd love to get started by having you give us a snapshot into your research and how you came to the field.
ERICA THOMPSON: Ooh, OK. So let's go back about 10 years then. I have a background in maths and physics: I did a undergrad science degree and then a master's in mathematics. After that, I was looking around for something interesting and useful to do and I ended up in a climate change program.
So I have a PhD in climate physics. And in that PhD, I looked at North Atlantic storms and how they would change in a changing climate. And so I did some work on mathematical models. I did some sort of look at statistical models, dynamical models, and sort of compared the two.
And at the beginning of that work, you see, what I did was a literature review. I did a literature review which looked at previously published results and found that you could find almost anything. You'd find a study saying that these storms were going to become more northerly, more southerly. They'd get stronger, they get weaker, and basically disagreeing outside the range of their own error bars.
So I suppose what I realized at that point was that it wasn't telling me all that much about North Atlantic storms. But it was telling me a lot about how we make models, how we use models and maybe the kind of problems that we have when trying to interpret information that we get from models.
And so my research since then, I still have a strong interest in climate change and climate modeling - but I've broadened out to look more generally at predictability in models and the problems of statistical uncertainty quantification from mathematical modeling results. What kind of methods can we actually use to get a handle on the real-world uncertainty, not just the variability in model land, as I call it?
So I've been looking at economic models, I've been looking at sort of short-term weather models and longer-term climate models. It's been really interesting to see over the last couple of years the interest in public health models. Obviously, epidemiological models with the spread of COVID-19 have been incredibly topical. So lots of people have opinions on them, and it's been really interesting to see that and compare it with modeling in other domains.
KIMBERLY NEVALA: Excellent. So let's start with a basic definition. You've coined the term - or at least you’re one of the first I've seen use it - this term model land. So can you define for us this popular but mysterious and I think often misunderstood destination that you call model land?
ERICA THOMPSON: Yeah. So I suppose I have to acknowledge that my colleague, Lenny Smith, was the first to use the term, I think. Maybe other people have used it before.
KIMBERLY NEVALA: Excellent, Lenny. [LAUGHS]
ERICA THOMPSON: But I suppose what I mean when I say model land is kind of when you're actually inside that model, when you are-- it's that sort of space you're in when you are inside your model, when all of your assumptions are true, everything works, you can do the maths, and you don't have to think about whether or not it actually relates to the real world. So that's what I call model land.
When you do your model, you draw a graph of the results, and you don't stop to think whether or not this actually applies to the real world. So there's the gap between model land and the real world. It is the question of: are my assumptions correct? How would I evaluate the model? How do I know that if I'm making predictive statements based on this model that it could relate, that it's actually reliable, in terms of the real-world outcomes?
KIMBERLY NEVALA: Yeah, there's been a lot of almost orthodoxy around this drive to become data-driven. And that push to become data-driven is often couched as an or: it's either you are data-driven or you are operating on gut instinct. Prone to all the vagaries and limitations we all know come with being human.
And implicit in that framing is an assumption that algorithmic outputs reflect an objective fact. They accurately reflect “reality” and that an expert view is a subjective opinion. Is that characterization part of the problem? Is that leading us astray? And what would be a better framing for this discussion?
ERICA THOMPSON: I think it's definitely part of the problem, yeah. I mean, I guess we're seeing more and more discussion around this. And I think as models become better, you start thinking, well, actually, are they really doing what we wanted them to do or maybe not?
But yeah, I mean, to go back, data never speak for themselves, right? So in order to get any result out of data, you have to sort of put it into a model and wrap it with some other assumptions about what the data are and how you're going to analyze them and what kind of results you want to present.
So if you're talking about models that kind of generate some sort of prediction, you know, yes, you can do your statistics of the past data and you can make a prediction about the future perhaps based on that model. But you always have an expert judgment question, which is, to what extent do I think the past is a reliable guide to the future?
And so I think you can draw a kind of spectrum there. You can say, well, in some cases, we have a system where actually, the past really is quite a good reliable guide to the future. Maybe it's a relatively simple linear system. Maybe it's something that we're only trying to project a small distance in the future, and therefore, we can say that we're not going out of sample, if you like. We're going-- the underlying conditions are remaining the same. And so we think that we have enough data to be able to check how reliable our model is.
So like a weather forecast. You look at the weather forecast. Anybody on the street will tell you how reliable the weather forecast is. You can say that tomorrow's weather forecast is probably pretty good, you know? Not perfect, but pretty good, and three days' time is all right. You would look at it. Next week, you maybe wouldn't even bother looking at it unless it was a special event that you were interested in. And in three weeks' time, they don't even bother to show you the weather forecast, because we know that the skill has decayed to such an extent that it's basically useless for any practical purpose. I mean, and then all right. There are a few applications where you might use that very marginal skill on the slightly longer term. But everybody sort of knows.
And then when you're talking about other models, that question of that time scale of applicability -- we just don't know. There is no intuition about it. So you can refer back to data. So the other end of the spectrum would be a climate model, I suppose, to take that particular example.
So when we're going from weather models to climate models, they're based on the same kind of physics. They're based on the same physical principles and our understanding of the system, this complex Earth system and the atmosphere and the way that the wind blows. And so we have that same sort of physical confidence, but obviously, we are trying to predict something on a much longer timescale than a week or two. So how far should we have confidence that we are getting useful information out of it?
Well, that's actually an incredibly complicated question, right? Because you can't just look back at thousands of years of past climate and say, we've got thousands and thousands of years of data and we did well in everything, and therefore, we're going to do well in the future. We have that to some extent. We do have climate models which have done well in the past, and therefore we expect that they are simulating well the kinds of processes that were important in the past.
But in order to transfer that into a judgment about the quality of the model in the future, we have to kind of step outside of model land and say, well, what is my expert judgment here about if the model can get those processes right in a pre-industrial climate. Or in a boring climate that we've had for the last few hundred years. What is my expectation that it will therefore be able to perform well to the same degree in the climate of the next couple of hundred years, which we expect to be hugely dramatically different to what it has been?
And so that's where the expert judgment comes in. And this is the same in other fields as well. In some, it's quite trivial. We just say, we refer to data and we can kind of expect that the underlying conditions are not changing that much, and therefore, past performance is a reasonable guide to future success. In others, the expert judgment is a much bigger component. And then you see, then I think we have to consider very carefully what we mean by that expert judgment. Who are the experts? What is the basis of their understanding here?
KIMBERLY NEVALA: Yeah, and one of the things that then comes out of that is being able to embrace, I suppose, a level of uncertainty, or at least confront uncertainty in that.
So some of what I hear you implying, perhaps, is that to use information that we're taking from models in an appropriate way - whether that's to automate something or to inform a decision or to make a decision - (and we can talk a little bit more about the optimization objective in a minute) we need to develop an environment where we are comfortable asking a lot of questions and not necessarily getting definitive answers. Is that a true statement?
ERICA THOMPSON: Yeah, absolutely. So I mean, if you have more than one expert, then you'll get more than one expert judgment, right? And so these questions of who is an expert become really important. You know, then it becomes kind of firstly, a question of authority and expertise, but also a question of trust.
Do we trust that an expert is making the appropriate judgment on our behalf when they're making kind of purely scientific judgments about the degree to which the future is like the past? But also the other kind of judgments about the kind of things that we're going to put into this model. What is important?
I think that's really well illustrated over the past couple of years with the debate about COVID models. You know, models, simulations of how things might change in the future based on different assumptions, different kinds of policies that could be put in place to reduce the spread of the virus.
And obviously, they have hugely different impacts on different groups of people. And so when you're talking about who should be making those assumptions and putting these assumptions into the models, you've got to think about what the impact is on different people.
KIMBERLY NEVALA: Yeah. And part of that debate was also not just looking at what the output of the models was, because certainly, we had models that gave us different outputs. And so this idea that there's one model to rule them all or one model that's going to definitively depict current or future reality is fraught.
But that's also about interpreting the information and the data as well. So we saw the same information cast in very, very different lights -- whether that was with good or ill intent. I won't opine here. But how do we, especially in the business realm or those of us individually more broadly, think about and break down those different perspectives and come to a reasonable understanding of what the information is that we're being presented with?
ERICA THOMPSON: Well, I mean, this is something I've been exploring recently. I think-- yeah, it's a great question. And I think a useful perspective is the sort of sociological view of models as being, quote, "not a camera," but an engine. As in, we don't use models to kind of take a picture of what some reality out there looks like. That actually, they become engines of co-creation. They are something that we use to exert an influence on the situation, right?
So I think the kind of virus propagation models are a really good example. The point of making a model of the spread of the virus was not to get it right. It was not to get the right answer. It was to inform policymaking so that something else might happen. If you predict two million deaths, then obviously, that would be a catastrophe, and you don't want it to be right. So you want to inform policymaking to be able to reduce it.
So really, you're not talking about the model being purely a predictive tool. It's actually something that you are kind of in dialogue with. You say, well, if I do this, what would happen? If we did that instead, what might happen? What are the kind of main drivers of this process? And can I kind of use it as a thinking tool to understand what's going on here?
And then not just use it to understand the situation, but then also to communicate it. So you say, well, somebody else says what do you think we should do? And you might say, oh, we ought to close the schools or we ought to implement a national lockdown. Well, that's a big thing to do. You've got to have some justification for that. And so the model also becomes the justificatory tool, right? So it's something that you are creating in order to be able to have a conversation with somebody to say, this is the best course of action for the following reasons, and then to explore that.
And so, you know - I think that you could go too far down that route - and you could get into the realm of conspiracy theories. And saying, well, people are only producing these models in order to force their own opinions and their own values and their own preferred political course of action onto a population. I think we see that as well in the climate debate. You can see conspiracy theories sort of bubbling up with these sorts of things.
So it is certainly not the case that we construct models solely in order to get a sort of scientific picture of what's going to happen. It is also a tool for thinking and a tool for deciding. And as such, I think it's that which makes them kind of particularly difficult. You know, particularly sort of tricky objects to study and to think about and to understand.
You can be solely in model land and you can say, well, here's the model. You know, it's perfect. We do our statistics and we get these answers. But that question of how you then jump back into the real world with all of the messy realities of incorrect assumptions, oversimplification, and the politics of the different decisions and differing opinions about value judgments, that is super difficult.
And that's the key step. And yet, it gets lost a lot of the time from academic papers that really are just operating in model land. And I think that's a big gap between academia and real policymaking or business decision making in that the academic can go away and kind of do something in model land. They come back and they give you an output. And well, it's completely useless, because the assumptions aren't realistic, and there's no sort of understanding of what the context is here. The context is always important.
KIMBERLY NEVALA: And so you have said also that because of the importance of that context that a model gives you an outcome. It doesn't make a judgment necessarily. Why can't or shouldn't we be strictly or solely looking to AI or more specifically algorithmic systems to make value judgments?
ERICA THOMPSON: I mean, so data can't make value judgments. Only humans make value judgments. And so yes, you can program your value judgments into an algorithm or into a model and use that to make decisions. And you can delegate your decision making to an AI, but that doesn't mean that it doesn't contain values.
They're there. I mean, they are in the optimization function. If you say, we are going to maximize financial profit or we're going to maximize social well-being or we're going to minimize suffering or whatever, all of those are value judgments.
And so I suppose what I'd identify as the problem with AI in general is that it is-- you have to define that optimization function upfront. So this is OK. Not necessarily a problem as long as we have a discussion about it and we're clear about what that optimization is and everybody sort of agrees, or at least democratically can agree to something, even if not everybody agrees in detail. There has to be some answer.
So you could do that, but then it won't be flexible. You know, how does it move with the times? What happens if something unexpected comes along? How is it able to trade things off on the fly when it-- or if value judgments change, right? If people sort of thought that something was important 100 years ago-- there are many things that our societies thought were important 100 years ago that now we don't, and vice versa.
So I think-- I don't think it's impossible for algorithms to be entrusted with decision making in some contexts. It can be a good thing. But we have to be really careful about, what are the value judgments that are implied by doing that? And whether we expect them to stay the same. And what happens in the edge cases? Are these troublesome? And we can think about that.
KIMBERLY NEVALA: And are there specific steps, whether those are questions we should be asking or processes or practices we should be adopting within organizations when we are looking to… Either looking to these models for what are very often highly impactful decisions, life decisions or business decisions, social decisions, or even minute decisions to ensure that we're having that rich and robust and often very nuanced conversation?
ERICA THOMPSON: Yeah, I think that's really difficult. I mean, it's sort of easy to just tell your engineer to go away and come back with an AI that will make this decision. And I mean, the trouble is that it's always possible to do that. You're not going to run into a situation where a computer says, no, I'm afraid I can't make this ethical judgment for you, because that's outside of model land.
The computer will make that ethical judgment for you if you tell them to. But is it the right one? Do you agree with it? Well, I mean, you've said from the kind of highly impactful all the way down to the minute.
And so the minute ones, maybe we don't care. Maybe it doesn't matter. Maybe the benefit of making a quick decision outweighs the possibility that it might make, in some sense, the wrong decision. And maybe it doesn't matter if it made the wrong decision. The outcome would be trivial. But for the ones where you're actually impacting real people's lives, for the ones up to sort of large-scale social decision making, I think it's incredibly important. And I am-- no, I'm not sure how you do it.
[LAUGHS] I mean, we have a system of democracy, I suppose, which says you vote for the party that you like, and they have some sort of system of government which puts in place the policies that you are then kind of deemed to agree with. But there isn't detailed consultation on things. There's just a kind of informal feedback mechanism where people sort of protest if they think things are going wrong.
But yeah, I think that is actually quite problematic, but it's a much wider problem than just mathematical models, right? It's a sort of social engagement with politics and decision making more generally.
And especially when we're getting into the realm of politicians making really large-scale decisions like action about COVID or action about climate change or action on the economy, actually, these are huge decisions that literally alter the course of history. And the opportunity to kind of feed into those decisions perhaps is very unstructured. I don't know. That's a very big question.
[LAUGHTER]
KIMBERLY NEVALA: Well, and maybe that's a smaller takeaway, at least within organizations and companies which is (the need to) build the mechanism to have that deliberate solicitation and consideration of input. And not having an unrealistic expectation that these are thorny issues that data and models somehow are going to spit out a magic answer and the key that's going to unlock it. It gives more information so that we can make a better-informed decision. But it's not always or shouldn't be considered the answer or the only way of looking at something.
ERICA THOMPSON: Yeah. Yeah, yeah. And not hiding those value judgments underneath the mathematics, I think. It's easy to say that something-- when something gets written down in maths, you know, it becomes an equation or it becomes sort of in computer code, and it's easy to kind of imagine that that means it is objective and that it doesn't contain values.
But I think the outcome of my research really is that values are present when we construct models all the way through: from the decision of somebody to fund an activity to the decision of the individual business or organization or scientists of what they're going to actually work on. Through to the kind of decisions they make about what is part of the problem and what is beyond its boundary. What the outputs should look like, who it is designed to inform. What kind of actions can be represented within the model, and what can't. Before you even get to the point of saying that outcome x is more valuable than outcome y, it's all there already.
KIMBERLY NEVALA: Is it fair also then to say that the best way we can develop and create confidence and grow that sort of data-driven, if you will, or model-driven decision-making muscle is not to try to unhide or eliminate the uncertainty, but to openly and honestly expose it and talk about it so that we are, in fact, neither overconfident or underconfident? Because it does strike me that there are real germane impacts from either overconfidence or under-confidence. And I'm interested in your thoughts on that.
ERICA THOMPSON: Yeah, absolutely. I mean, I think the tendency is for sort of-- when we are in model land, if you like, to become overconfident, because there is a tendency to leave things out and to simplify.
And the things that we've left out are the things that we don't understand. They're the messy things. They're the difficult things. They're the edge cases. They're the tricky bits that we didn't quite work out how to put in, and we thought we could safely get away with not putting in.
And so I think certainly not all the time, but much of the time model results are overconfident in an outcome. And so if we take that outcome directly from the model back to reality, then we're making an implicit expert judgment that the model is perfect.
And we know that no model is perfect. All models are wrong. All models contain simplifications. Otherwise, they wouldn't be useful. They'd just be a duplicate of the real world, which is not helpful. So that kind of overconfidence, I suppose, results in risk. And it results in us optimizing for the wrong things potentially.
KIMBERLY NEVALA: Yeah, optimization is such a -- it seems to be a primary objective a lot of times when we're talking about algorithmic or AI-driven systems these days. And it began with this idea of automation, and automation looking at fairly routine and mundane repetitive tasks. But is quickly moving up the stack to automating more substantive, I would think, decision making functions or tasks. With some sort of expectation that better, faster, and everything can be optimized. Or that we can always find the right answer, I guess, through optimization. Is that an unrealistic expectation?
ERICA THOMPSON: Definitely, yes. I mean, I suppose it depends on what you think the right answer is. If you are happy to sort of encode all of your value judgments into model land and then optimize and just take that to be the right answer, then by definition, it's the right answer.
But I think some of the exciting sort of research that's been done recently is around the question of whether these actually are the right answer. If you construct a statistical language model or something which is running and can generate indistinguishably human text, is it right for it to be accurate, or is there something-- is there something else that we're interested in?
Do we want something that reproduces the views of the average human, or do we want something which can be imaginative, something which can be creative, something which has care for the people who are going to be impacted by whatever it is that you're using it for, whether it's a legal judgment or a newspaper article or whatever? Thinking about the end users or the end sort of community that will be actually influenced by the outputs of these models.
Statistical accuracy probably isn't the top of the list, right? So say we're translating something from one language to another, and we translate something with gender-neutral pronouns-- they are in the kitchen-- and it comes out as, she is in the kitchen. Is that a good thing? It's probably accurate. It probably scores best. It probably wins on the optimization. But do we end up reinforcing stereotypes? Do we end up constraining people's opportunities and what they see purely for the sake of this statistical accuracy?
I think we don't want to be doing that, because obviously, there's more to life than statistical accuracy. But if you are in that sort of very narrow AI ecosystem, it's easy to lose sight of that and think that statistical accuracy is the best, the highest good and the only kind of target of optimization.
KIMBERLY NEVALA: Yeah, we had an interesting conversation just recently with Sheryl Cababa, who is the Chief Design Officer at Substantial. And she also pointed out that very often, the way that we're using these algorithms is… Well, the algorithms themselves are inherently homogenizing, and they're driving us towards - she used the example of autofill - a place where we all sound the same and we respond the same. And there's something a little icky, for lack of a better word, about that. Even though in the moment, it's kind of nice to really quickly respond to an email.
ERICA THOMPSON: Yeah. And it's definitely homogenization. I mean, in pursuit of statistical accuracy, if everybody responded in exactly the same way, then the models would perform better. So we shouldn't be surprised that they are trying to push everybody to be the same, because that is when they will perform the best.
So if the optimization target of the model is accuracy, then it would like us all to be the same. It would like us all to be predictably buying the same pair of shoes after you bought the jumper. It would like everybody to be predictably replying in the same way. It would score better if we did that.
KIMBERLY NEVALA: So you do research into this area. What are the emerging best practices for how people can really ensure that they're first getting a realistic evaluation of a model, and that we're both understanding and then applying the information that's being derived from these models in appropriate ways?
ERICA THOMPSON: Yeah. So I suppose it starts by defining what the purpose of the model is. Because we can't evaluate a model, we can't evaluate the success of a model or a decision making system or anything else, unless you define what the purpose is. So you have to start by saying, what is it for?
And that defines, that determines how you will design it and how you will sort of implement it. And it also determines how you evaluate it afterwards to say, was it successful? So I think you have to start by saying what it's for.
And then I think making the distinction between model land and real world is really important. It's so easy to get sucked into -- even just trivially, because you've given the same variable name to the thing in the model as you would refer to it in the real world. So you say, this is the wind speed in the model, and that is the wind speed in the real world. But that one is a kind of-- it's some sort of representation of the average wind speed on a 100-kilometer grid. And this one over here is the wind speed measured by a little thing going around two meters up in the air at a point in the physical world. And these are actually completely different things, even though we call them both wind speed.
So just thinking about the difference, thinking about if we are-- maybe we have a model of COVID spread, and we have an average sort of individual agent who is walking around and interacting with others and spreading it to others. Thinking about how they are different from the person to whom you want to communicate your results.
If you are going to say how people should change their behavior maybe based on that model, what is it that is similar, and what is it that is different? What are the essential insights that are being taken from this mathematical model and transferred by a process of expert judgment into the real world? So making that distinction.
And I think there's making that distinction within the model and then there's also making that distinction when we communicate our results. So you could come, having sort of done all your nuts-and-bolts research, you come back to the person that commissioned it. You know, your boss or of the funding agency or whatever. And you say, well, here's our results. They're interesting. So don't say, we ran our model 42 times and we got the following distribution of results. Who wants to know that? Actually, I don't care. That's in model land. That's in your model land, which I don't understand, because I haven't spent years working on it.
So if you're going to present results to someone who's a non-expert, I think that there's a responsibility on the modeler to be the one who is able to make that expert judgment and own the results and say, I am making a statement about the real world. I have done my modeling and I have found the following outcomes in the model, the following relationships. And I believe that they apply to reality in the following way.
So that might take the form of adding error bars. It might mean that you take a range of uncertainty from your multiple model runs. And you might extend that range a bit to make it relevant to the real world. You might say, in order to compensate for the fact that I know that my models are imperfect and not fully adequate, I will just add in an ad hoc way a little bit of extra uncertainty onto it to account for that. And, therefore, to give me a chance of not being overconfident in the real world.
Of course, they still might be. I'm making what might seem like quite an arbitrary judgment in doing that. But the only place that that judgment exists is in my head. I am the only one who's able to make those decisions about the difference between the model land and the real world.
So I think maybe when policymakers or the business consumers of this kind of information are looking at a report, picking out the sentences that are in model land and deleting them. Going in with a red pen and saying, I don't care about that - tell me what you actually think the real world is going to do, I think that's really informative, actually, because that starts the discussion about, how good is this model? What are the possible failure modes have? You got kind of an understanding of where it's going to go wrong? And that would be really useful to know if you're the decision maker.
Or alternatively, maybe the modeler comes back to you and says, nope. This is my absolute best guess. I put everything I can think of into this model. I have no further expert judgment to incorporate. The model literally is my best guess. Whatever it says, I'm going with. And that's also really useful because it gives you an accountable probability distribution. It gives you an accountable forecast of the future.
And you can go back to somebody and say either you got it right or you got it wrong. Why did we get it wrong? What was wrong with the model? And I mean, there's a nice phrase about models, chameleon models which are ones that are sort of presented as being policy-relevant or decision-relevant when they're sort of before the fact. But then after the fact, when it turns out to be wrong, somebody goes, oh, it was only a model.
KIMBERLY NEVALA: [LAUGHS]
ERICA THOMPSON: So you sort of take credit for the successes, but you deny responsibility for the failures, that kind of chameleon move. I think that's really, really cheating. That's the sort of thing we have to get away from. There has to be accountability for model judgments. And I think the best way to do that is to expect communication to be in the real world and not in model land. Not settle for model land results.
KIMBERLY NEVALA: Yeah, and very often, I think we do (settle). And it's incumbent also then on decision makers or policymakers or business consumers to perhaps redefine accountability. So accountability is not about a guarantee of certitude. It's about a statement of uncertainty: which is, we're not asking you to make a judgment or a perfect judgment. We're asking you to tell us what the judgment is with sort of all of the warts involved in it.
And that's a pretty fundamental shift in how we think about it. But I imagine that for a lot of modelers out there, there would be a sigh of relief as well if they were also being actively encouraged to discuss their findings in this way.
ERICA THOMPSON: I think there'd be a sigh of relief, but I think there'd also be a gasp of horror.
[LAUGHTER]
I think that it's sort of-- it's easy to be in model land and to be able to present a report that just says, the model said, you know? I ran my model and I got the following results. You know, you can kind of shove it away. You know that nobody's going to really come back to you on that, because if they do, you can just say, oh, it was only the model. We know the model is wrong. All our assumptions are written down there. And so you can kind of slide away from that accountability.
And I think it is actually quite a big ask in a lot of cases to ask the modeler to make the judgment about the gap between model land and the real world. And I think it needs to be incentivized. And yes, some people certainly would breathe a sigh of relief at being kind of given the license to do that.
But I think some would certainly find it difficult to make those judgments. I've certainly had a lot of discussions with people who, if they're asked to make those judgments, they say, well, that's not really-- I don't feel comfortable making it, or that's not really my expertise. Well, that shows you that they sort of live in model land. If they are producing a model of something and they don't have the expertise to say whether that actually corresponds to their best guess of the real world, that's interesting. Maybe concerning.
KIMBERLY NEVALA: Well, and it also underscores the point of discussion that data science or modeling or algorithmic decision making is a team sport. And putting all of the onus for all of these judgments and decisions on a singular person - who can by definition only have their point of view and will have blinders in certain areas - is just absolutely unrealistic.
ERICA THOMPSON: Yeah, and I think that's a really good point. It is a team sport, all of it. Yeah.
KIMBERLY NEVALA: [LAUGHS] So as you look forward, what are you looking forward to in your research, and what's on the horizon in this area?
ERICA THOMPSON: I guess I think that there's a lot more to come in this area.
I think that, especially with all the debate about COVID and the sort of policy decisions that were taken over the last two years, I think that is really relevant to all of these questions, and it's going to rumble on for a long time.
I think that climate change is also a really big one where we are seeing a lot of discussion about the role of models and the difficulty of interpreting models, and all of these questions about value judgments and about how the models embody political choices and political values.
So I think there's a huge amount to be done here, and I think we are only at the beginning of this kind of exploration of getting away from maybe the naive sort of very-- sort of maybe over-scientific or over-mathematical-- the kind of mathematical wannabe, if you like. Where we think, well, if only the real world was like model land and everything was wonderful and ideal, we'd be able to just put everything in and make the best possible decision.
And I think in the 21st century, maybe what we're realizing is that decisions have to be value-driven, not data-driven. We need the data. We can't throw away the data. And we absolutely can't throw away the models either. But we need to understand how we are using the models as a tool, and the way that they can sort of mislead us, and the things that we might have to be really careful about when we're implementing these kind of decision processes. So I think there's a lot to look forward to. Yeah. I'm excited about the research in this area.
KIMBERLY NEVALA: Well, thank you so much. It's been an absolute pleasure traversing the limits of model land with you. And there are certainly interesting times and interesting debates ahead, so we look forward to seeing your future work as well.
ERICA THOMPSON: Yeah, thank you. Well, it's been really nice to discuss with you. Thanks for having me.
KIMBERLY NEVALA: Building on the foundation Erica has just given us, Marisa Tschopp, a human-AI interaction researcher, will help us understand what AI systems think we think. You won't want to miss this fascinating discussion, so subscribe now to Pondering AI.
[MUSIC PLAYING]
Creators and Guests
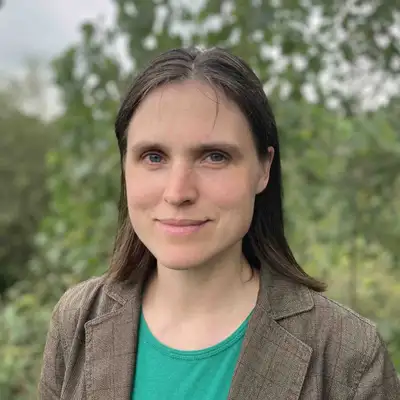
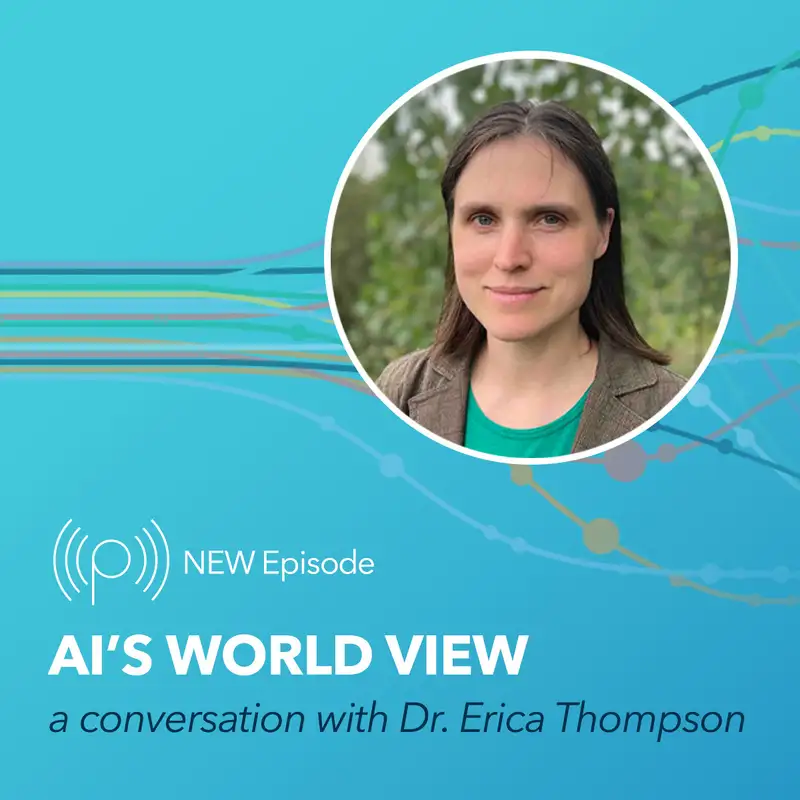