Power and Peril of AI with Michael Kanaan
[MUSIC PLAYING] KIMBERLY NEVALA: Welcome to the Pondering AI podcast. My name is Kimberly Nevala. I'm a strategic advisor at SAS. And I'll be your host this season as we contemplate the imperative for responsible AI. Each episode, I'm joined by an expert to explore a different facet of the ongoing quest to ensure artificial intelligence is being deployed safely, fairly, and justly now and in the future.
Today, I'm joined by Michael Kanaan to talk about the power and the peril of AI. Michael is the former chairperson of AI for the US Air Force, headquartered at the Pentagon. He's won numerous accolades for his work in AI. And because he's not one to sit on his laurels or, I think, to sit still, he's also the author of the must-read book T-Minus AI. Michael, thank you for sharing your time with us today.
MICHAEL KANAAN: Kimberly, it's a pleasure to be with you.
KIMBERLY NEVALA: So let's start by learning a little more about your background and how your mission, so to speak, in AI came about.
MICHAEL KANAAN: Sure. I'm Mike Kanaan. As you mentioned, I'm the author of the book T-Minus AI and formerly chaired AI at the Pentagon. And for myself, I think we all have individually unique artificial intelligence journeys. My journey began upon graduation from intelligence school, which is in Texas.
My first assignment was at the National Air and Space Intelligence Center. It was there we had this new mission. It was a thing called a hyperspectral imager. And one would ask, well, what the heck is a hyperspectral imager? And how could that inspire you--
KIMBERLY NEVALA: It sounds so obvious.
MICHAEL KANAAN: --working on artificial intelligence? Well, for you and myself, we see in three color bands. A mantis shrimp sees in somewhere around 12 color bands. One might philosophically ask, what does a mantis shrimp see that we do not? But a hyperspectral imager-- it sees in hundreds.
And what we could do is we could fly planes in the Middle East during the daytime there, nighttime where I was, back in the United States, of course. And we would use and collect the scans and images from the ground, from the air. And it would reflect sunlight. And looking across these various color bands, we could identify what the materials were.
So if you imagine, if you will, we're sitting back, and we're sending these massive data sets back from the Middle East into Ohio. And our responsibility was to, at a moment's notice, be able to tell a sailor, soldier, an airman, a marine on the ground, hey, there's some material there, sometimes seconds, minutes, feet away from a convoy. Stop. You need to be concerned about that.
Now, this was back in 2011. And if you were talking about artificial intelligence then in commercial and academia and business, you would say, well, but of course-- image net. Machine learning is here. Computer vision is here. But within the government, we sometimes move slower and, in some ways, more thoughtfully or diligently. And we weren't quite there yet.
But we weren't the only ones wondering, well, how could we have used machine learning and these capabilities to do better, to see more? And it was that that began this groundswell movement of inspiration to bring these capabilities about in the government and move forward in terms of IT and the rest that we're still on this journey now about a decade later.
KIMBERLY NEVALA: I think that's so interesting. And your book really did an excellent job, I have to say, laying out the path by which AI has evolved more broadly, as well as some of the opportunities and challenges ahead. In your research, did you learn anything that really surprised you or challenged your thinking in a fundamental way?
MICHAEL KANAAN: In a very meta sort of sense, it was an exposure to one's own biases. So we worry about bias and data-- in AI, worry about bias and data. And I had to worry about that in the case of writing the book.
I wrote the book not to be an excited portrait of some utopian future or dystopian sketch of all we should fear. I wanted to undertake it to objectively address the current realities and realistically true future implications of AI so to explain the technology on the journey in an engaging narrative and provokes a common call that AI be implemented only ways with consistent with fundamental human dignities and, of course, only for purposes consistent with democratic ideals, liberties, and laws so that we could inspire a common and grounded conversation.
Now, what's difficult about that? One would say, well, whose biases? Whose view of the world? So in the course of writing the book, it was an exposure to what that looked like and then undoing it. The first step to recovery is recognizing a problem. We all have this problem. So how do you do that for 270 pages, was the goal.
KIMBERLY NEVALA: Well, I think you actually-- there's lot of probably humility, both personally and for us as a society, that comes about going through that journey. Now, you and I have talked before about the tendency to anthropomorphize-- I can never pronounce that word, so I'm kind of proud of myself right now, but-- our technologies and to ascribe, to some extent, some human level of agency or even awareness.
And the book draws this really interesting distinction between intelligence and consciousness. Can you elaborate on that for us a little bit and why you felt it was important to differentiate those concepts?
MICHAEL KANAAN: I'd love to. We really only know of one example of advanced intelligence in the universe, this difficult word that we have a hard time conversing about. We're speaking past, below, around, and through one another. And since the time of the dawn of our reign on this planet, we've never seen an intelligence that hasn't arisen with consciousness as a prerequisite. And we really don't know any level of consciousness that occurs outside of animal life.
And even as humans, even early on in life, we tend to understand consciousness only comes with heartbeats and blood. So tellingly, to this point, it's rare that some child doesn't impute a sense of fictional consciousness into a stuffed animal. You have when you were young. We all did.
On the other hand, think about it this way. It would be pretty strange for a child to be talking to some nondescript, round, brown piece of plastic, right? But if other pieces of plastic, ones that look like perhaps eyes and ears and everything else, for instance, are pushed into that, well, voila. Consciousness suddenly becomes a thing that we eagerly assume. And in fact, this is the original design genius behind the Mr. Potato Head toy. If you give a kid ability to construct something that looks biologically alive and capable of sensing animal input, then look, consciousness.
So despite what we might intuitively think consciousness is, we really don't know how it arises at any level. And maybe we never will. There is no consensus in any branch of science that explains how it arises from the pure physiology of a biological brain, all those electrical processes and chemical things taking place.
Moreover, and until recently to this point, we used to think that consciousness, again, is a prerequisite from intelligence. And the latter only arises from the former. We never saw anything that was intelligent that wasn't consciousness. The phenomena always go together.
With that all said, though, it's important for our businesses for us, as we explain it to both professionally and with our neighbors, to explain that machines can exhibit some characteristics we've normally attributed only with biological consciousness. So for the purposes of our workforce, we need to set some definitions.
The first is, let's just define intelligence nowadays as something doing something for a purpose. Surely, in this case, I could say that a thermostat has at least a unit of intelligence. It can change the temperature.
And as for learning, which is another key word that we're discussing here, we can define that is changing over time given a set of inputs or, in the case of machines, data. Big foot stomp here that I think we'll get to. If we take that as the example, then a Nest in our home has both intelligence and learning involved. I have a Nest in my home. And it knows that I like the temperature at 67 degrees and, before pandemic life, I wasn't really in the house from 8:00 until 5:00.
The point being is when we're discussing artificial intelligence, we have to move consciousness, which was at the bottom of the stack-- consciousness, intelligence learning. And we got to put that all the way on the other side. We can have things that exhibit intelligence. And they can learn without the soft mushy stuff.
What we really need to do is get beyond the whole scary terminator or what is AI conversation. And the definition for most of the community and in business is defined as the ability of machines to perform tasks that normally require human intelligence. And look, it's correct and precise in a certain way. But I think you would agree we can really unpack a problem with this definition in practice for the workforce-- frankly, for society's collective understanding-- to the point we're making here.
The problem is it's a rolling definition. Under that, an abacus was AI. TI-83 calculators, which I know we love from our student days, those were AI at some point. Excel files were AI. And now Google search is AI.
And in that definition, like with consciousness, we anthropomorphize it with a nod towards the human domain. And we keep living in the cycle of disillusionment with statements I bet you've heard often, Kimberly-- well, that's not real AI, which I've never understand what people mean by that-- when otherwise missing the boat to capitalize on the ways it can, does, and will profoundly impact us and our efforts now.
So for the purposes of this conversation for your workforce that is out there, let's be practical and pragmatic. Simply put, artificial intelligence is designed to analyze data and formulate predictions without any overall guidance from us. That's it. Regardless of the technique, the semantic games that might be played, that's all we're talking about here. And hopefully we can get beyond that conversation with two better foundations in mind.
KIMBERLY NEVALA: Yeah, I really like that. And that point really struck me because the instant we start to ascribe, I think, human consciousness or a human level of consciousness to these systems is often when we start to subscribe them or start to think of them almost as having a conscience and the ability to act with independent intent. And this, I think, is in a lot of cases where things can really start to go awry. Before we dive into some of those circumstances and talk about AI's inherent dualities, are there any other common misconceptions or popular concerns you think need to be addressed more broadly as we continue adoption of AI?
MICHAEL KANAAN: I think that's an interesting notion to sort of double down on. And it's this idea or notion that we're just going to magically do AI, just sprinkle it on top. I like to think about artificial intelligence as more not a thing. But it's a journey and the end state of doing a lot of other things well in business-- your hardware, your software, your architecture overall, and furthermore, our ability to then deliver that stuff.
So I think something we miss out on is not just espousing the notion that we will have artificial intelligence. It is asking ourselves, if I had the most elegant, performative algorithm in the world, could I deliver it if I tried? And the answer, far and beyond, tends to be no. And that is exactly where, then, a dogged focus and investments should be.
In addition to that, I think many think that AI is going to replace people or the worker bees per se. And AI is actually going to have the greatest impact with your subject matter experts or the top of your workforce. So as leaders, when we're framing an AI problem, we really want to look for three criterion of stuff we have.
The first is high volumes of data. So think about as that a ton of XML files. We want to have high velocity of data. So think requiring decisions to be made quickly, or we are making decisions quickly. And the third-- and this is the twist-- is highly accurate data or examples of what we do.
The third criterion is where most organizations fall into that disillusionment, never quite deliver it, and never gets to the point where we are using it to illuminate new insights that we didn't see before. Without many examples of what you do, AI is wholly ineffective in practice in business nowadays. So I think that's another piece that we kind of missed the mark on.
KIMBERLY NEVALA: Yeah. And I want to dive just for a minute a little more deeply into that idea of, how do we apply AI productively? And then we'll go back and look at some examples of where it may be applied for good and for ill.
A lot of times, I think, when we think about it or having this conversation about how humans will engage with AI and these systems in the future, the most common lens that we are using is this question of automation, which in its furthest extension is replacing human work, or augmentation as an assistant or an accelerator. And it seems to me that you're implying also there might be a different way to frame the problem or a lens we can apply here to think about where and how AI can be applied most productively.
MICHAEL KANAAN: I think that what we really need to do is separate the terms "automation" and "artificial intelligence." And we really don't do that very well. We use them interchangeably. But they're really not whatsoever interchangeable words. So what do I mean by that? And I think that it is our responsibility to be very precise in this mechanism.
Automation comes with, for a lot of people, some rules in the road. And in addition to that, we're talking more at the worker bee level. We're talking about tasks, not jobs. Automation performs tasks. AI informs jobs. So here's the point. Not all AI is automation. And not all automation is AI. Automation sometimes uses AI, and AI sometimes results in automation.
So as AI informs and drives more, it's going to move our automated tasks from simple rules-based systems, which are good in their own way, to integrating learning technologies that might automate an action or a prediction afterwards. So what we need to do is have real discussions tomorrow driven by our understanding today and know where presenting change or that learning mechanism is good or where we need to stick to rules in the road and business.
KIMBERLY NEVALA: So I know you've talked in the past as well about thinking about AI as enhancing decision-making, using it to observe and orient ourselves instead of making, necessarily, decisions on our behalf. Can you talk a little bit more about that and what the implications, then, are from a business decision-maker and thinking about applying AI to that side of the spectrum?
MICHAEL KANAAN: We want to be smart about where humans are good at human things and where machines are good at machine things. Stemming from a guy named John Boyd, actually, from the Air Force, he created a concept called the OODA loop. And the OODA loop is this circle that encompasses Observe, Orient, Decide, and Act.
And it was funny. I was at a VC a number of years ago having a conversation. And he said, do you know about the OODA loop? I said, well, most certainly I know about the OODA loop. But this is a concept that goes into business, too. That is, if we can shrink any of those functions, we perform faster and we get ahead of our competitors. It's kind of self-licking ice cream cone.
When we think about where machines are really good, I think that what we want to talk about is the "what" of our lives, the observe and orient functions that we do. So what does the "what" look like? Well, think about it. The most common examples of AI that we use that people understand is, did you unlock your phone today? Well, it used thousands of infrared dots to scan your face compared to what is stored in order to ID you.
Have you ever received a fraud detection notice from your bank? Well, that's machine learning automatically using your history to determine what is usual and then notifying you of anomalies that don't quite work. Or, for instance, writing an email that we all see on Gmail nowadays with finishing off your sentences, it's finishing with what words seem to be most likely and it sees most often, so examples of things.
These are all incredibly beneficial to us. They support us. And they serve this observe and orient function. That way, we take the humans out of too much of that task-saturated stuff. Tasks are not jobs. And we put them into decide and act kind of point of the OODA loop itself. And I think binning this in these two ways makes it easier for us to start AI projects smartly and then also to dispel some of the concerns that we have of scary AI making decisions.
With that said, though, we can very quickly understand, for instance, if you hop on Facebook or LinkedIn, it provided you news on what it learns you like from everything you engage with, looked at longest, and what your friends like, et cetera, and filters out what doesn't resonate with you. So if we're not smart about paying attention that that's representative, then in our decide and act portions, we're not making smart choices. I think everyone talks about AI on LinkedIn. But that's just because that's all the algorithms give me.
KIMBERLY NEVALA: Yeah. It is interesting. And I have a tendency to want to read things, although I get quite angry and my partner sometimes suggests that I should not do that, that I fundamentally don't agree with. And I'll see my feeds really flop between this focused stream of things that I really fundamentally don't agree with. And then I have to be very deliberate about going and looking at the other things and trying to get balance. It's really fascinating.
MICHAEL KANAAN: Do you ever think the algorithms are too responsive? Here's a company idea for someone out there.
KIMBERLY NEVALA: Yes.
MICHAEL KANAAN: Just because I watched one YouTube video or read one article that was maybe outside of my norms, please don't mess up my entire homepage for the next two weeks because I watched one video.
KIMBERLY NEVALA: Yeah. I think it was Ganes Kesari. And it was a LinkedIn post a while ago. And he said, I've had to go back to tracking some of the people that I really want to follow and whose ideas I think are interesting outside of my LinkedIn and Facebook and social media feeds because those algorithms are so good at targeting and getting so differentiated and focused on the one last thing that I looked at that I'm actually losing track of everything else. And so in a weird way, this very advanced technology has forced him to go back to a very manual process from before, which I thought was really interesting. So I'll find the link and send it to you.
MICHAEL KANAAN: I'm glad I'm not alone in thinking.
KIMBERLY NEVALA: No, you're not. So you've talked about some examples of AI that are probably, for the most part, fairly innocuous. But one of the things when we have these conversations I think can be difficult to wrap our minds around a little bit and to think about how to deal with is there's this dichotomy and a level of uncertainly, I think, that come with AI.
I don't believe there's a way for us to develop the underlying technologies, sort of the core capabilities in ways that just will fundamentally preclude their use by bad actors. In a lot of cases, the same technology or the same base capabilities can be applied to deliver great good can also be applied to deliver great bad or outcomes we would not agree with. In fact, in some cases, in the development, for instance, of some of the natural language generation and so on and so forth, the mechanisms we use to improve those systems are in and of themselves-- can then in and of themselves be used in ways that are nefarious.
Can you talk a little bit about that inherent duality and maybe provide some examples of where this comes into play? And then I think I want to talk a little bit about how we think about better due diligence around these systems. But let's talk about some examples where the core capability can really be used for great good and great ill.
MICHAEL KANAAN: Well, now that we've gotten out of the way the consciousness concerns and some evil-minded machine intent to destroy us, those aren't the concerns to have. But what concerns we should have and what should we pay attention to is a question. Upfront, as has always been the case and will remain, the narrow and specific uses to which we ourselves put our machines will be where the solutions reside and the problems arise, too. We have to worry about how to regulate and control it the right way. It's a powerful tool.
But historically, in our past, powerful tools were in the hands of only a few or only accessed by a few, ones I mean that could really compromise hurt or disenfranchise people, a nuke, for example. Well, it's pretty tough to go watch the first chain reaction underneath the University of Chicago in the '40s or steal it from an underground cave in Tennessee or go find the fissile material.
But here's my point. I'm not comparing it to nukes. I'm comparing the process. We have to let research do its thing-- advance the science, chart the unknown, discover new. And that whole process is not so much regulated or has oversight intentionally. It has to do its own thing. But with this new technology, though, it's open. It's available. And to your point, it has an inherent duality.
Deepfakes are something we talk about often. In fact, the other day-- I don't know if you saw it, the new deepfake technology or just release of the application that could bring portraits of people who passed away back to life.
KIMBERLY NEVALA: I find this incredibly creepy. But we should not go down that rabbit hole now. But anyway, yes, I did.
MICHAEL KANAAN: Right. So that's a whole different conversation. But I didn't take it as that. I took it as, wow, I can finally have that living Harry Potter Hogwarts portrait I always dreamed of having. And I hope it hangs in the Pentagon or something one day.
Anyways, the point is and what I'm trying to showcase is the accessibility to this technology. So at a technical level, it's quite impressive. And the research community largely drove these advancements, by the way. In a different conversation, the research community primarily funded and included as the people who sell the technology nowadays. That's kind of a new issue that's a little bit arising.
But the underlying techniques to make and use deepfakes are pretty advanced. And they've been democratized to everyone. They're also the leading technique and what we use to detect, for instance, breast cancer in mammograms, which is great. That's why we have technology. That's why we want to do this for society, though we also all know that deepfakes create myths and disinformation.
So the question is, well, what do we do about that? Or how about, most recently, too, the leading team play and strategy algorithms and techniques associated with a subset called reinforcement learning? They happen, like many other AI advancements, in games. But those games right now are literally openly available. And they are real-time strategy, top-down look with even the fog-of-war war games, like StarCraft and Dota.
So it's very cool, the advancement of the science and research. And we need to freely move that forward with creativity from the best minds in the world. But again, now it's digital.
And you might be creating something that-- back to the point of, who uses it, is the real question. So how do we regulate use without stepping on research is a very difficult conversation to have. But we need to do so in an informed, thoughtful way that requires not trying to fit square pegs in round holes, but carving out some new square holes.
KIMBERLY NEVALA: Yeah. And if we take this maybe down a level to organizations that are looking to apply-- so getting a little more tactical and practical-- I wonder sometimes if, in the interest-- because we are excited about our creations. We're excited about the potential and the positive potential of what we can do with these solutions, whether that's something we're trying to deliver out to better serve our customers or our consumers or something in the public sphere, for instance, better predicting and protecting mental health or distributing services.
But I do wonder if the processes we have in place today-- if we spend too much time focused on what our positive intent is at the expense of some really mindful critical thinking, building that into the process about the implications of the solutions that we're deploying. What are your thoughts on that?
MICHAEL KANAAN: I agree with you. I think that what it demands and calls for is more conversation between public service, commercial companies, and the rest that-- the question of, who are you serving? Governments serve the people of the nation. And I want to celebrate all the companies doing so much good. And you can do well by doing good. But they have a fiduciary responsibility to subsets of groups.
So what it demands is more conversation at hand. And also, I think, another piece is that we cannot allow for those who are focused on its use in the future-- so existential risk side of the conversation-- not to communicate with those who are on, perhaps, the modern-day, current machine learning side.
So really often, when we get to this conversation which makes me think we're not having the progress we need, there will be people who are raising their hands and saying, see? We must be careful with artificial intelligence. This stuff isn't working. It's not like our brains. It's having bad effects. And they're leaders in the world on these positive, important, important messages.
But then they say, and because of that, I don't want to talk to the modern-day machine learning people. Why would I? What's the point? And modern-day machine learning people who are putting out product and working on the things that are at hand will say, well, I've got stuff to worry about tomorrow. So I'm not going to worry about 10 years from now what's going on.
So what I think you're inspiring here is this demand from big business and big government to not look past each other, to have shared knowledge. And that's on both sides, right? Government has to be able to communicate contemporarily and in ways that are effective, speaking the same language.
And then those who are developing the technology-- you can't absolve yourself and say, well, I made it for only this purpose. So when it was used for bad, that's not my problem. Or those who are just paying attention to the ways it can infiltrate or hurt our lives can't blow off the people making it right now. So it demands this really multidisciplinary conversation.
KIMBERLY NEVALA: So what does due diligence, then-- so we're talking about some of these broader conversations that need to happen and that back and forth. But for a company, for instance, that's looking to deploy AI to drive whatever that might be-- we've got insurance companies trying to utilize this to better predict patient needs and, I think with good intent, be proactive in getting in front of you with services that will support you and help health, et cetera, et cetera.
What kind of due diligence and thinking lens can they apply to that in the day-to-day, actual, practical application of AI today? Are there practices and controls we need to really lean into at that more day-to-day applications today?
MICHAEL KANAAN: Responsible AI has to be a foremost concern. And we have to recognize that mistakes will be made, period. It's just going to happen. So let me give some compelling reasons for the ethics conversation. Or we'll call it "responsibility." I think that's a nice, commonly used colloquial term.
And it's best crystallized by imagining dilemmas, whether you're a company or in government, that you will most assuredly face. The first is-- beyond the fact it's just the right thing to do in line with our values-- is that whether there's a mistake of our own doing or stems from someone with some bone-to-pick agenda item on the public-facing front, executives and businesses must be ready to answer the following very simple question from a comprehensively defensible public position.
My question is this. What due diligence did you perform on AI when the mistake was or is made or an individual life was or is disenfranchised as you, of course, continue to integrate AI into all aspects of your business because that's what you tell me every quarter? The answer now would probably be insufficient. In fact, I think for many, it'd be nearly unanswerable.
And it exposes leadership and credibility and potential negligence on a huge scale, one that's truly international. It exposes arguments we make about that whole "we can do well by doing good." We couldn't point to enough informed leaders and offices with the appropriate authorities, knowledge of operations, or even understanding of AI to defend diligence across the lifecycle.
And that lifecycle is way bigger nowadays. You can't call up your attorney the night before you release a product because it stretches from the companies you work with, the solicitation, the data acquisition you have, the testing and evaluation you do, the operational use over and over and over again in perpetuity, to the policies and oversight guidance you have, and all of those steps and everything in between you don't even realize.
So by necessity, we'll need to account for each of those simultaneous processes, unlike all the advancements and technologies of the past. So hopefully, in real conversations, what we do is that we're prepared for or hold it a priority to make this a holistic effort, then to institute, as we have done so many times before, responsible, ethical, and moral development into the lifeblood of any department, any group, any service you're offering.
And I know that this seems a little esoteric, fanciful, or intangible. But it exposes us. And we at least need to pay attention along the way and do due diligence. And if you're in business, the question is, well, if you didn't perform due diligence, what kind of damages do we have to do to compel you to never do that again? They're going to be pretty high.
Now, on the flip side, knowing we're going to make mistakes, when you make a mistake, you certainly pay for them. But you don't pay for the rest of the chain to never do it again. And I think that could at least be a reason outside of, shoot, let's just make it embody the best of us that we should do.
KIMBERLY NEVALA: Yeah. And I think there's an emergence today. We've certainly seen a profusion of ethical principles, for instance. But now we're looking at practices where we can start to ask these questions about not just, what am I intending to do, but will the solution actually affect that outcome, and basic things like, is the population or the environment I intend to deploy this in actually represented in the data or the-- which is essentially the data that this thing is trained on.
One of the other things I'm thinking a lot about now is I'm observing a tendency towards, I think, perhaps an overreliance on the idea of explainable AI-- so techniques that allow us to really approximate an algorithm's internal logic. One of the criticisms that's thrown out, I think, a lot around AI is that we don't know how these systems really work. We don't know the internal logic. We don't know the rules or path by which they come to a bet.
And so this idea of explainable AI is a silver bullet for some of these very vexing concerns. And I think it's a tool in the toolbox, but it's certainly not the only one. What's your perception of explainability? And are there other things such as fairness and other things that we need to be considering more broadly?
MICHAEL KANAAN: Well, I think what you raised, which is kind of a good point, that-- even though we're talking about artificial intelligence, you can't just throw not just the kitchen sink, but the whole kitchen out the window. There are certain ways just from the sheer standpoint where you said, hey, was the data representative of the environment or people in which it was deployed to?
Just that in an email is a huge deal because it signals thoughtfulness. And that's the kind of thing, that's the kind of business conversation, I think, that we need to always have. And it's centered on being educated about the topic and understanding how it works.
So I might get a little shellacked for this. But I do not like the word "explainability." It's anthropomorphized. The most complex neural network is the bias that's in our own brains. Explain to me how you know this. And if an artificial intelligence could talk to you, I think it would actually just say much like we would.
So if I asked you, Kimberly-- because you're sitting in front of a microphone right now-- how do you know that's a microphone? And if you sat back for, I don't know, 10 minutes and really got to the root of it, you would say, well, after my 29 years of life, I would sit back, and I've seen a lot of microphones. And I've seen things that aren't microphones. I know what they do. And listen, I'm pretty convinced that's a microphone.
AI would probably literally tell you the same thing. So what does that really mean? I wish that when it came to explainability or something that looked like this thing that embodied our views, I wish I could encapsulate human dignity and just say, look at that AI that defends human dignity or is in line with it. That's too hard to solidify.
So when you think about it, I think key words are responsibility, equitability, traceability, reliability, controllability. But most of all, I think about fairness and representativeness. So in this case, we'll do an imagining, like a thought experiment. Imagine an x- and y-axis. And on the y-axis, you have this thing called "data" or world "view" because that's what data is to a machine, which is experience for us. That defines our world view.
On the x-axis, let's place it scope, reach, or number of individuals or groups that are affected. Any of those terms works just fine. And then let's start plotting things that we know of.
Alexa-- well, I don't want an Alexa in my home that has pretty broad scope, reach, and use that's only trained on Southern white gentlemen or, alternatively, just people in Northern California. It's too broad. It affects too many people. That would be unfair and misrepresentative.
So I think once you start plotting these things, you start to at least have the conversation. Well, does that world view seem fair to who it affected? And how could we see this play out? A computer vision algorithm at a local police department. Well, let's ask the question. Were the people of that community reflected in the data?
And was it diverse and representative and inclusive and the rest? Or was it only from people who had pictures of their face on the internet, which, vis-a-vis, if they had the internet, it's probably a smaller group of people. So at least when you do that, you start to see things that are like-- maybe some regulatory action comes from that or at least conversations we can handle.
KIMBERLY NEVALA: Yeah. And I really do believe fundamentally just having those conversations and, as you say, assessing whether the world view that we are ascribing to these systems actually represents the world view of where they're applied. And to some extent, I think really just making sure that we bring a lot of people into that conversation and asking the question is the most important first step.
So certainly there's just a lot to think about here. And I think we could probably-- or I could yammer on with you for a long, long time. But some final thoughts-- what are you most concerned about over the next few years in this space?
MICHAEL KANAAN: OK. Upfront, the community can't keep looking like me. The proverbial boardroom that looks like every other boardroom I've ever been to can't look the same. The problem is we're in a very vicious cycle of keeping the same old going.
And there will be a day where those behind us might refer to this generation or this inflection point as you're asleep at the wheel collectively-- if we don't try to change it, those who have an ability to change it. So each of us, as I mentioned before, should have our uniquely own individual and meaningful journey towards understanding the AI. The problem is that wherever we are, whether it's at home or at work, we think it's just for tech people or engineering, which is predominantly small groups of people who all look the same.
But AI, robots, automation, algorithms, all those buzzy things you hear so much about-- they're going to infiltrate and empower our lives much like electricity does. But unfortunately, that will also disenfranchise those not represented in data or just generally aware. If there wasn't a single advancement today, it'll still profoundly impact human opportunity and experience.
And furthermore, in just this year alone, the US is going to graduate somewhere around 50,000 people for STEM-like degrees. We have 500,000 open jobs in this market. But we also live in a world where the barriers or at least the availability of education have never been lower. That doesn't mean people can access it.
So I don't care if you have a college degree or not. Have you done it? Can you do it? Do you have a passion to learn it? And how do we as a nation help drive down the gap in this space is what matters. Because in only a few short years, that jumps to 1 million. So we need to reimagine what the job space is and who can fill it.
It's 2021. The digital divide still separates our youth from education and our urban and rural communities, which have a lot more in common than they might think, from opportunity. These technologies are infringing on human rights across the globe. Yet our society doesn't discuss it or at least are generally aware of the conflicts and potential compromises we face. We all can influence the path and provide opportunities for our kids, our friends, and our neighbors.
And mark these words. The future rock stars in tech-- they're teachers. They're sociologists. They're dancers. They're artists. They're psychologists. They're parents and so many more. So just ask this. What are you doing to better understand and help others do the same is what matters.
Do you love dance? Help people dance with a robot. Are you a writer? Show them a language transformer. Are you a psychologist? Help us deal with how people handle life change driven by tech. Are you a wildlife or a nature preservationist? Well, why don't we use computer vision to track and predict concerns or lions migrating across the savanna? Are you a political representative? Then help us bridge the digital divide and present it to more people.
The point is AI is for everyone. So we, us, what you so much lead, Kimberly, the people listening now, we can be a part of at least changing that. So whatever comes in the future, we're totally ready for.
KIMBERLY NEVALA: Yeah. I think this is so awesome. And paradoxically, I think we like to poke at the sore points and the weak points because we are so excited about the potential. And you've just shown this potential across this massive spectrum of applications and passions that people have.
So I think that's just fantastic. And I really like the way that you've helped us conceptualize some of these things. And if you haven't already read the book, you can find a link to Michael's book, T-Minus AI, and some of the other resources mentioned in today's episode in our show notes. Thank you again, Michael. This was a fascinating conversation. And I so appreciate your time.
MICHAEL KANAAN: It is always awesome to be with you, Kimberly.
KIMBERLY NEVALA: Awesome. And you've actually set us up very, very well because in our next episode, we're going to continue the conversation with Tess Posner.
[MUSIC PLAYING]
And Tess actually is an educator. She's an advocate for diversity and inclusion and equality in tech and the CEO of AI4ALL. So Tess, who, along with Michael, is a personal inspiration for me is going to share just a wealth of knowledge about how we can ensure the AI-enabled future is bright for all. So make sure you don't miss it by subscribing now to Pondering AI in your favorite podcatcher.
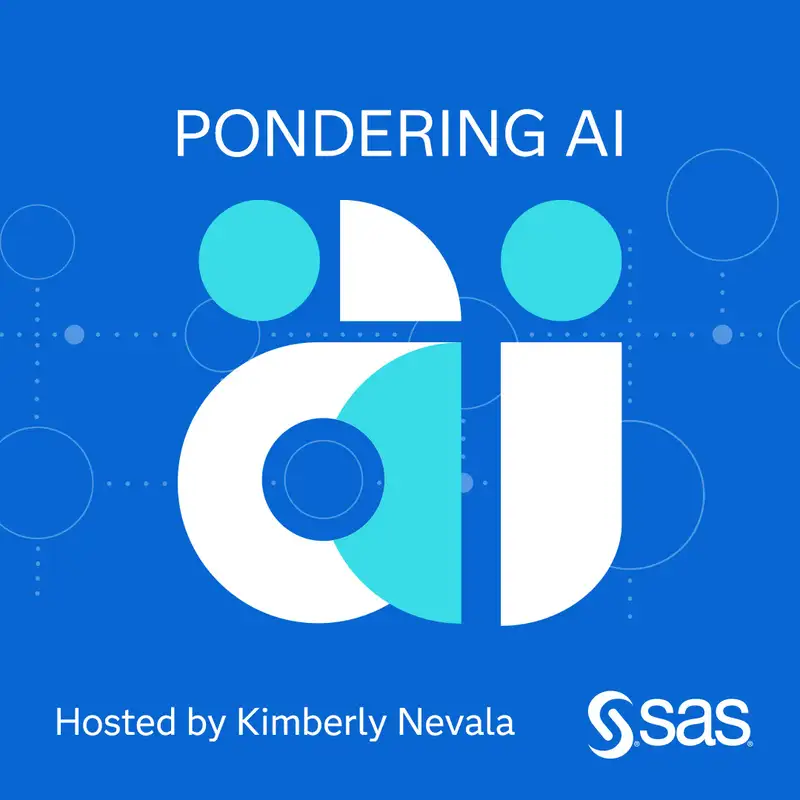