In AI We Trust with Chris McClean
KIMBERLY NEVALA: Welcome to Pondering AI. I am your host, Kimberly Nevala. Thank you for joining us as we ponder the reality of AI for better and worse with a diverse group of innovators, advocates, and data professionals.
Today, we're joined by Chris McClean. Chris is the global lead for digital ethics at Avanade. We'll be discussing the intersection of digital ethics and AI and the nature of trust, amongst other things. Welcome, Chris.
CHRIS MCCLEAN: Great to be here. Thanks for having me.
KIMBERLY NEVALA: Let's start by giving folks a quick recap of your professional journey through to your current role and studies. Or, in other words, how does one become a digital ethicist?
CHRIS MCCLEAN: Sure. It's a great question, I think, for people in this space at this time because we all have such a meandering journey. A very few of us, I think, went to school for this or have been in this space for a while.
I actually started off just over 20 years ago in marketing, of all things. And primarily, I was doing marketing for small technology startups, mainly in security, privacy, risk management, and compliance. So really, from the get-go, helping business and technology executives understand all the kind of risks and harms of technology. I moved from there pretty quickly into market research and then consulting. At that point, I was at a company called Forrester Research and helped scores of companies build their risk and compliance programs. And a lot of that started to bleed into enterprise risk and corporate compliance.
The part of that that fascinated me the most was corporate social responsibility, corporate ethics, basically, how do companies behave. How do they do the right thing around some of the decisions that they're making? So at the time, I got a Masters in business ethics and started to do a lot more research and consulting work around helping companies build ethics programs.
Then Avanade found me. They had done some work around digital ethics, some work with clients, and some thought leadership. But needed somebody to kind of pull all that together and really, play two roles, which is what I'm doing now. One is internally, helping our more than 60,000 people around the globe understand digital ethics issues and how to incorporate more responsibility into what we design, build, implement, and operate. And then also externally, I do a lot of consulting and advisory work with our clients around digital ethics.
And then another quick aside, about a year and a half ago, I started a PhD program in applied ethics, specifically looking at risk and trust relationships.
KIMBERLY NEVALA: This is excellent. If there's ever a time to be an ethicist in technology, it has to be now. Certainly, we see a ton of articles and speakers talking about the fact that ethics is no longer optional. And while I'd agree and like to believe that's true, it's not apparent to me - headlines to the contrary - that this is the case in practice. Some of that skepticism may come from an observation that many ethics practices are really risk management practices in disguise. So can you talk to us first about what are digital ethics? What does that encompass?
CHRIS MCCLEAN: Sure. So the way I think about it is the intentional practice of imparting values throughout the technology lifecycle. So from the very early stages of conception through design, development, implementation, and operation, you are imparting ethical principles into your decision making. How is data collected? How does this interface interact with people? Where is the data stored? And what kind of processes are we supporting? For me, a big part of that is thinking through the impacts on individuals, on society, and on the environment.
And once you understand all those potential impacts from the technology, making sure they're as positive as possible. So it's kind of like risk management in some ways, that we are trying to eliminate the harms and potential future harms. But we're also aiming for positive ethical outcomes. We're thinking through things like accessibility, inclusivity, and diversity, respect for privacy, and mental health, and well-being, and so forth.
KIMBERLY NEVALA: What are some of the key differences that we should be cognizant of between digital ethics and risk management and/or compliance, et cetera?
CHRIS MCCLEAN: Yeah, it's a really important question, I think. And again, I have tremendous respect for the disciplines of risk management and compliance. I did a lot of work with companies in both disciplines. I think anybody in, let's say, responsible innovation or responsible AI, any of those fields, they have a lot that they can learn from risk and compliance. Policies, procedures, controls, assessments, a lot of that sort of governance and oversight, all of those are very important to have in an ethics program, as well.
But for me, there are four key reasons why we should distinguish between ethics and risk management. I'll try to go through them relatively quickly.
The first is that as a discipline, risk management is almost always going to be inwardly focused. That is you talk about risks to the enterprise, whether they're legal, operational, financial, or reputational. So if you think of something like why should we avoid using an algorithm to screen out resumes, the risk to the enterprise would be, well, if there's bias in that algorithm, then we could get sued. Or there could be a regulatory enforcement action or maybe some reputation damage. But if you think about the ethics of it, you're thinking they're individuals who might be looking for a job after having not worked for months. Or maybe they're a single parent, and they have several children. And they're not working. And the ethical harm that we're doing to those individuals, it's very hard to calculate alongside the risk to the enterprise.
The second reason is actually because of that. The first reason is that risk management is, by definition, a prioritization scheme for remediation. You're trying to reduce uncertainties. If you try to compare those two different potential harms, a lawsuit against the company versus screening out somebody because of some protected characteristic, it's very hard to navigate which is the bigger risk that I should spend my remediation time and effort on. So that's number two.
Number three, a lot of the harms, a lot of the downside of the technology that we use, the things that we have to consider in tradeoffs are not risks. So if you think of AI, specifically, we know that there's a big investment from an environmental standpoint in the hardware that it takes to run these AI systems, in the energy cost, and so forth. We also know that a lot of these big models that we've been talking about in the news lately, they're built using a lot of cheap labor. People that are not paid well and not treated very well and are necessary for the creation of these AI engines. Those are, I would say, harms or downsides that we should be considering. They're not risks. So if we're thinking risk and reward of using an AI system, we're going to ignore some of those harms.
And then finally, the fourth reason is that risk management, because it's disciplined in reducing uncertainty, you're only ever going to be trying to strive for neutrality. That is removing uncertainty, removing that risk. You're not looking for ethically positive outcomes. So if you take, let's say, ChatGPT, if you're a University, and you're thinking through an ethical risk assessment, you're thinking, well, it could be used by students who want to cheat. There could be a risk to their ability to learn certain content. It's going to be difficult for the teachers because they're going to have a lot longer papers that they have to grade. Those are all risks that would be mitigated. But if you're thinking about an ethical impact assessment, you have the possibility of thinking on the positive side. So from a learning perspective, ChatGPT actually might be a great tool for learning in some scenarios. That's a positive ethical outcome of this new technology. It's not an ethical risk to be mitigated. It's a positive outcome to be approached or vied for.
So those are four reasons that I think we should distinguish between the two. Again, I think risk and compliance and ethics should be working very closely together, if possible.
KIMBERLY NEVALA: The idea of looking for a positive, or beneficial outcomes, or ethical impacts is interesting. Because, again, this is not something you can weigh on either side of the scale. So it struck me as you were talking that - I don't know if ironic is the right word – that this is one area, unlike risk management where we are trying to quantify what the risk or the harm may be, ethics may be an area where a data driven approach may not actually be warranted or appropriate. Is that fair?
CHRIS MCCLEAN: Yeah, I think that's right. And maybe not ironic but perhaps counterintuitive because we are looking for potential tradeoffs.
And the tradeoff might be I like the idea of protecting people's privacy. I also like the idea of making certain data available to support law enforcement and investigations. And those two things can sometimes be in conflict. We can't really quantify the two, but we can talk through those issues on an ethical scale and think, OK: how can we protect people's privacy but also do what we can to support law enforcement? It's not a tradeoff in the same way you might think of risk and reward.
KIMBERLY NEVALA: Yeah, Kate O'Neill has spoken about being able to hold two ideas seemingly contrary to each other: both/and not either/or but both/and (in your mind).
Do you make a distinction in your work in studies between digital ethics and AI ethics?
CHRIS MCCLEAN: I don't make a big distinction.
I think with the marketing background I had a long time ago, I used to spend a tremendous amount of time with technology vendors and other marketing people and executives, really trying to parse through the language that we're using to define a market category. And I think you can go crazy trying to figure out, OK, what's the difference between ethical innovation, or ethical AI, or responsible AI, or trustworthy AI. I think, for me, so many of these disciplines or these programs are trying to basically accomplish the same thing.
For me, my role is specifically digital ethics, but I also spend most of my time on responsible AI.
So I consider it part of my jurisdiction to focus on responsible AI. I'm also doing work around responsible metaverse implementations. Avanade actually does a tremendous amount of work around modern workplace and all the tools and technologies that we use in our office environment to get business done.
I spend a lot of time with those technologies.
It's all digital ethics, but AI ethics or responsible AI is a big part of it.
KIMBERLY NEVALA: Yeah, I would like to talk to you a little bit about the work in the workplace and the metaverse. Before we go there, are there any insights or observations you can share about how we're approaching - call it ethical AI, responsible AI, digital ethics - in terms of where we're doing well and where organizations may need to start expanding their focus?
For example, a lot of the conversations and the initial focus in programs tends to be around bias. I think this is a great, amenable place to start. It has the benefit of being somewhat concrete: which is not to say that defining an intended outcome is easy -- queue the inevitable disagreements about what is fair here.
But it's certainly not an unusual or risqué topic anymore and it's a worthwhile starting place. But what else or what might we be overlooking if we're focusing primarily or only on bias in these assessments?
CHRIS MCCLEAN: Yeah, it's a really good question.
I think as I mentioned earlier, a lot of my process starts with impact, thinking through what technology are we building, and how is it going to be used? How might it affect people? And then specifically, what are the potential ethical impacts of that technology, or system, or process?
So if you are working in a company that is building smart cars or driverless vehicles, the ethical impact of those AI engines that are driving that car. If you get that engine incorrect, if the AI is not working appropriately, there are major health and safety implications, right? Also, just the space, in general, has economic and labor force implications. So if you're thinking through the ethics of driverless vehicles, the impacts are very different than if you are thinking through the ethical implications of, let's say, a chat bot or a resume screening tool. Those will have implications related to fairness, or respect, or dignity.
So if you start from what are we building? And what is it hoping to accomplish, what are the ethical impacts? You're going to come up with a whole lot more potential areas of focus rather than just bias.
So the digital ethics assessment framework that I built actually looks at 30 possible categories of impact.
And we look at the domains of individual impacts, so things like privacy, accessibility, inclusivity, mental health, physical health, opportunity, and financial well-being. Those are all things that a technology could impact on an individual scale. We also look at societal impacts. I mentioned law enforcement. We have technologies that could impact education, health care, politics, military, and so forth. And then finally, we look at environmental impacts, so the hardware, the materials used, the energy used. You have potential impact on pollution or other operational impacts.
Then we have a 20-point assessment that's on the tail end of that impact assessment that will look at potential controls. So if we say, OK, we care about fairness as a value, we know that this technology has impacts around things like opportunity, and health, and privacy. Then we can use that set of impacts and say: OK, if we care about fairness, how do we make the resume screening process more fair? Or how do we make this chat bot more accessible?
So we use the values based on the impacts we've identified and then the controls as the back-end part of that assessment. It's complicated work, but I would say there are so many frameworks and guidelines out there that are basically just a list of values, fairness, trustworthiness, accountability, transparency.
And those are all good to keep in mind, but they don't mean anything unless you can apply them to the impacts that technology will have.
KIMBERLY NEVALA: So what is the reaction to that framework? Because as you said, it is complicated. Do you get the deep sigh and the subtle backing up? Or are folks finding ways to do this in a way that is manageable and operational? What's the general reaction?
CHRIS MCCLEAN: It's a very fair question. Again, having that background where I've worked a lot with people in security, and compliance, and risk management, they're very familiar with these very lengthy and complicated frameworks. You know ISO, and COBIT, and IEEE, and all these standards out there that are comprised of a lot of different controls, and policies, and standards, and things like that.
But you're absolutely right. In the space of responsible tech or responsible AI, we generally haven't gotten that far down the road yet. So for example, I will use this framework as the basis for the work that I'm doing with clients and internally. But I've also built tools to make this very accessible for people that don't think about ethics on a day-to-day basis.
For example, I built, using a Microsoft Power App, a 12-question survey. And it's very easy, kind of straightforward yes or no, that helps to triage a project based on its baseline characteristics. So is there or is there not personally identifiable information as part of this project? Yes or no? Is there a user interface? Will people be interacting with this system? So we provide this app, so people can very quickly go down this list of questions and say, yes, we're doing this. No, we're not doing this.
From my standpoint, it helps alleviate a lot of their potential concerns. Say, OK, we don't have to worry about privacy with this project. We do have to worry about health and safety concerns. And it also helps provide information for me about whether or not I need to get involved with that project. Or the degree to which our digital ethics processes have to be part of this particular engagement.
So yes, the detailed framework is quite lengthy and complex, but we're doing everything we can to make it accessible and make other kind of tools and processes accessible for the people that are having to code every day or people that are doing data science work on a day-to-day basis.
KIMBERLY NEVALA: Yeah, it's such an important point there. Which is not all domains or aspects that you could look at in the comprehensive framework are going to apply to any one application. So I like this idea of really pinpointing from principle to practice.
I wanted to take a slight -- I don't know if it's a turn, necessarily. Awhile back we had a really great conversation with Marisa Tschopp. And we were talking about these concepts: of the difference, for instance, between trustworthiness and trust, and so on and so forth.
Trust is a very funny thing. There's quite a bit of research and anecdotal evidence that show that we humans are often, against all logic and even evidence to the contrary, overly trusting in the outputs of digital systems. AI, in particular. We can see this right now with a lot of the breathless pronouncements and predictions around ChatGPT, for instance.
And I'm sure there's numerous factors. Is it naivete? Is it just an intense desire for it to be true, this sort of optimism about the future? It's exciting. Is it magical thinking? Is it other? What, in your experience and what you've seen, what lends to that tendency not to necessarily be skeptical but to be overly trusting? Which can cause its own set of harms.
CHRIS MCCLEAN: As I mentioned, I'm currently doing my PhD research on trust, specifically. So I think about this quite a bit. And we could talk for hours about the topic. It's tremendously fascinating, and there is a lot of research out there.
For me, I think the easiest and probably the most accurate way to think about trust is that it's a stance that some entity is a good steward of some power. So if I trust a driverless vehicle, or if I trust, let's say, a corporation to manage my finances or if I trust my neighbor to watch my house. I'm basically putting some power or maybe acquiescing some power into their hands because I deem them to be worthy of that power. So if I think about trust in case of, let's say, an AI system or a technology system, I'm saying I'm OK with this system doing a certain job for me. Putting potentially me at risk, but also potentially other people at risk. And that power could be beneficial for me. It could be beneficial for other people.
But I'm saying there are a lot of different ways that I might come by that stance. I might have a deep, emotional connection with a certain individual who built the system. Or I trust this corporation because I've worked with them a lot. I know or, at least I feel, that what they are doing is going to align to my expectations and values. But whatever it is that causes me to have that stance, I'm basically coming to a conclusion to say it's OK that this system or this corporation has a certain power.
The thing I get nervous about - and really what a big part of my focus is on the research - is that so much of the discourse on trust is related to interpersonal trust. I trust my phone. I trust this news article or this news outlet. And for me, I've been thinking in terms of more like extra-personal trust, something beyond interpersonal trust. Which is if I make a decision or I take a stance that some entity is worthy of some power, it doesn't just affect me.
There are a lot of other people that could be put at risk, based on my decision or my stance. So let's say if I trust this driverless vehicle: I'm OK getting in this vehicle and having it drive me across town. And that puts me at risk, but it also puts at risk all the other people who might be driving down the street or walking down the sidewalk. They are affected by my decision, as well.
There are two examples just in the last couple of years about how this really could play out on a much bigger scale. One is with the Michigan Unemployment Office and one is with the Dutch Tax Authority. Where these government agencies decided here is a company that will build us this set of algorithms to identify fraud in the people that are requesting this kind of government service: one for unemployment insurance and one for, I think, child credit for the tax authority.
In both cases, this set of algorithms, this AI system, misidentified tens of thousands of individuals as fraudulent. So it cut off payments. It enforced fines. It cut off other services. And the implications, if you read into this, are quite drastic for a lot of the families. This is the kind of thing that tears families apart and potentially worse.
And so this idea that an individual or a small number of individuals at these authorities trusted an AI system. They have that sort of interpersonal trust that says, I don't think I'm going to get fired over this decision. I think this company is pretty good. They're going to build me a good system. They have some personal risk related to that decision. But my guess is they did not do an assessment of all the various risks that they are imposing on these tens of thousands of individuals that were misidentified as fraudulent. And that kind of, I guess, expansive view of trust decisions, I think, is tremendously important. There's all kinds of implications that I don't think fit into our current schematics for trust.
KIMBERLY NEVALA: And would it be fair to say that part of the problem too is sometimes, as creators of these systems (or even in how the systems themselves provide an outcome, or an answer, or suggestion) they're done very -- I think you've said with an authoritative tone or with a high degree of confidence either implied or inferred in how something is presented. Is that adding to the confusion?
CHRIS MCCLEAN: Yeah, I think so. That is largely a desire by the creators of AI systems, right? There's the Turing test, and people are trying to build something that's going to sound like it's human and have the kind of authority and interpersonal confidence of a human. So I think a lot of it is on purpose. It's meant to sound authoritative and have that kind of deep expertise behind it.
And I do think another concept of trust is that it flows back and forth. It increases and decreases. It's not like a one-time thing that you can say, yes, I trust, and that's it for my lifetime. There are ways to reevaluate trust or monitor performance to determine, should I still have trust in this system?
So if you, let's say, decide, OK, this system is great. I think it deserves some power. I don't mind if it runs this process for me. I'm still going to monitor the outcome. I'm still going to look and see, is it really providing the performance that I was expecting? It sounds like it has the authority and the expertise to do this kind of thing. But if I take my actual experts, my human experts, and watch over the result of this analysis, we're going to identify maybe some places where this machine is not working as well as it's intended. And my trust level is going to be reduced a bit. So I'm going to have more monitoring and oversight. Maybe I'm going to have that human in the loop that might override some of those decisions.
So this interplay of trust, I think, should help determine, again, what level of trust we have. And that will dictate the level of oversight, the level of involvement, and the risk that we're willing to take, based on the decisions made by these machines.
KIMBERLY NEVALA: You've written about the interplay between what I believe you termed digital makers and digital takers. And a lot of what we focus on is how can we, organizationally, look at practices and processes to ensure that the makers - the organizations and the teams developing these systems - are stepping up to their responsibility.
But in reading some of your work, it was really important, I thought, to underscore the need for us to level up as individuals, as well. Or at least, that's what I took from it. I don't know if that was what was intended. And that requires an increased level of, again, another term I haven't necessarily seen in this context, digital intelligence.
So can you talk a little bit about the thinking behind the makers and the takers, and what we may need to do from an individual standpoint? Especially for folks who might be consuming some of these systems or applications, and not be in tech, and not be thinking about these things more broadly.
CHRIS MCCLEAN: Yeah, I think it's a really great topic.
I will give credit to my colleague, Eunice Kyereme, who actually came up with the idea of the digital makers and takers. She's a great consultant in our modern workplace line of business.
The idea is that really, whatever your role is -- you might be a designer, you might be a software engineer, a data scientist but also you're a user. And maybe you help influence technology decisions. Maybe you're in procurement or finance or HR. In any of these roles, we all have a part to play in making the digital universe or the digital ecosystem.
So certainly, as an individual who's designing, developing, implementing, or operating these technologies, you can make decisions on a day-to-day basis to consider your impacts. And think through, OK, how can we improve these impacts. You'll reduce the negatives. You aim for the positives and so forth.
But even as what we might consider a digital taker - someone that's using these systems or maybe influencing these purchase decisions - you can also influence the digital ecosystem. So let's say, as a consumer, you think it's a really good idea to have one of those connected doorbell cameras. You're getting benefit as a sort of taker, right? But you're also contributing to a landscape, where data is being collected on people who are delivering things to your home. Or, actually, there's been studies that showed those cameras can pick up sounds from 30 feet away. So you're actually contributing to the digital environment in that you are recording your neighbor's conversations. And that data is going to be stored on some servers that are owned by some company that they never have interacted with or certainly haven't consented to have their data stored by. So you are helping make the digital ecosystem with some of those decisions.
And if you think just in the news recently, these big engines like ChatGPT. We're learning more about the people that were behind the making of these systems. Not just the big brands that we’re very aware of, but also these workers in Kenya that were apparently treated very poorly, that were paid very poorly.
And without their work, these engines would not be fit for purpose. They would not be showing up in our office environment. They would not be suitable for using in, let's say, college exams or papers. So their work was tremendously important, but are they compensated as much as they should be? Whereas, let's say, I might be a taker. I might be using one of these systems without considering what went into it.
The last thing I'll say there is that if you are influencing these purchase decisions or use decisions. If you are working at a large organization. People in procurement have a tremendous ability to influence the vendors that are trying to sell into their companies. So asking simple questions like, how do you make sure the people that are contributing to this technology are fairly compensated, fairly treated? We have all kinds of third-party audit standards and guidelines that you can easily fit some of these questions into. And big companies do this all the time. I would say if you are part of that process, there's a whole lot you could do to expand the conversation about responsible innovation and responsible tech.
KIMBERLY NEVALA: Now, we could go down a long conversation on all of those aspects.
You mentioned the folks, for instance, who are inadvertently picked up on a Ring camera. Or are starting to use something like ChatGPT. But you also do a lot of work in the workplace. And there are certainly aspects of this where folks can feel like they are - and sometimes really are - just the object of the system. And (they) don't necessarily have a lot of agency.
If we look at some of the trends right now - and I would call it surveillance - and I'm not just talking about cameras, here. But if we think about the digital and the data footprint that we're putting in the workplace today to monitor at a really detailed level what people are doing, how they're working…I'll just call it employee surveillance, in general. There's this relentless push for productivity and efficiency and it's not at all clear is going to lead to the outcomes the business would like. Because what can be seen and what can be measured or captured from data may not actually, ultimately, be what's important and what matters. How do we navigate this kind of circumstance? Let's look at the case of surveillance or monitoring for purportedly performance purposes in the workplace.
CHRIS MCCLEAN: Yeah. It's a tough question, a very complex landscape.
For me, the distinction between monitoring and surveillance, surveillance usually has a connotation of suspicion. You are surveilling people that you think are going to be acting inappropriately.
Monitoring in the workplace, I think, has very legitimate use cases. We have monitoring all the time for protecting corporate, or employee, or customer assets. You think of information security, trying to monitor who's accessing certain systems and why. Are people downloading massive files over the weekend from the CRM system? That kind of monitoring, I think, is very important for risk management purposes.
I think the tougher argument would be that the kind of monitoring I think you're talking about, where it's looking at employee's behavior, how many emails are they sending, and who are they chatting with.
There have been use cases that have been either tried or hinted at that are looking at your face during a meeting and saying, are you happy, or sad, or upset, or impatient during this meeting. And I have a really hard time trying to articulate a use case that doesn't feel like suspicion coming from the employee. If I'm being watched all day, and the number of emails, and the number of files, and what my eyes are doing during the day, if all of those things are being tracked, I am going to feel like I am a suspect, right? That at any point, the company is going to say, we don't think you're doing the right thing.
So I would say that there probably are use cases where employee monitoring is going to be helpful for the employee. Where if it's from a performance perspective, maybe you are looking at your, let's say, customer relationship or customer service team. And you realize that after about three hours being on the clock, they start to have a little bit of a low. Maybe we need a new policy that says we should have them take a break every 2 and 1/2 hours. That's a legitimate use case and a good outcome. It requires some monitoring. But even in that case, I would say it's important to be very transparent about what data you are collecting and aggregating, how it's being aggregated, who's using it, who's accessing it.
I always think of privacy in three different categories. One is data collection. One is data control, and one is data use. So let's just take that one use case. You want to look at data collection, what data is controlled and how. Data control, who's going to see that data? How is it stored, accessed, secured? Do the employees have access? Can they see it? And then data use. Is it being used to improve policies, to improve, say, work life balance, or the number of rest breaks, which could contribute to mental health and wellbeing? Or is it being used for very strict performance management, which says something like, we are going to let go the 5% of people that are not spending as much time sending emails or on the phone. And if you start to get into those other use cases, like we are looking to fire people, or we're looking to punish them in some other way, that is going to feel like surveillance, like we are under suspicion of acting inappropriately. And I think employees in the long term are not going to operate well in those environments.
KIMBERLY NEVALA: Yeah, I suppose that ties back to your previous point. Which is ensuring that when we're looking at the systems - and maybe this is where ethics, again, comes in outside of compliance or risk management or performance management. Which is looking for what you call ethically positive or mutually beneficial aspects of systems and making sure that actually exists for something that we're going to deploy.
CHRIS MCCLEAN: Absolutely. So much of it comes down to intention.
KIMBERLY NEVALA: Yeah, for sure. Now, you are doing some work today around the metaverse. And I'd love to have just a short chat about that before we let you go. I think this is a really interesting case study or, perhaps better stated, an experiment in progress.
Because the metaverse is, by digital design, a maximally - I'm going to use surveilled - but also monitored ecosystem. It's entirely encapsulated. All of your actions are there in a digitized form to be seen and analyzed. So it's an interesting proving ground in some ways, I think, for these concepts around providing true agency, managing privacy, this idea of mutual beneficence or ethically positive outcomes, which I love. (I might start using that, fair warning.)
How are you seeing the metaverse being instantiated in the corporate or business world, first? And then maybe we can talk a little bit about some of the ethical risks and issues your clients and workers are grappling with as a result.
CHRIS MCCLEAN: Yeah, certainly very interesting times.
I think there are ways to use metaverse-type environments or experiences, again, in ethically positive ways. I see all kinds of really interesting cases, where let's say an individual has a health challenge. They are having a tough time getting in-person treatment. They can at least get into a virtual environment with a physician or a doctor. They can talk through some of their symptoms, creating that sort of a relationship or establishing a relationship that they can trust this doctor. And they can maybe get better health treatment because of that. Or one of the best cases I've seen, or at least hypothetical cases, would be, let's say, a child is sick. Maybe they're in the hospital bed, but their favorite band is in town and they want to attend a concert with their friends. They can do so in a metaverse environment whereas in real life, it would be a major challenge.
So I think if we think through what the metaverse could mean for some people, there are great benefits around accessibility, giving people with mobility challenges greater access to in-person services, whether, let's say, financial or health care. Great use case, right? If we think through how we are using these kinds of environments and experiences, I think there's a lot of good that can come out of it.
I will say we know for sure that there are current tradeoffs. A lot of them are environmental cost: the amount of energy it takes to support these environments and simply the hardware. Some of which is going to be obsolete in a year or two and has to be thrown away. So we should be very practical about some of these tradeoffs. But think through some of the benefits of being able to see somebody in a virtual environment from a customer service, from a patient perspective, from a mobility perspective. There are all kinds of benefits we should be thinking through.
Once we have those use cases that we're pursuing, that's when we start to talk about impact. We can say, OK, what is appropriate with regard to collecting private data? If you think through the cases where we've seen, on social media, the amount of data that's collected, not just on our PII, our standard personally identifiable information, but our behavior, our attitudes, who our friends are, who we interact with. Maybe some of that data being used for political purposes, that's scary enough. But then if you think in a metaverse environment where something is watching your facial expressions, where your eye is lingering. How much better are marketers going to be at helping really create advertising that's very, very effective for an individual - based on where their eye lingers in the commercial setting? There's so much more possibility to abuse private data. There's an expansion of what it even means to have private data. What constitutes private data is going to be much, much bigger in a metaverse environment.
So yeah, all kinds of potential performance and, certainly, ethical issues to think through there. I think it's really, really interesting. We do have customers that are moving in that direction. And we're working with them on some of those decisions. Still early on in a lot of cases, but there's so much there to go through. It's really fascinating work.
KIMBERLY NEVALA: Yeah, and over the years, we've thought about the idea of personally identifiable or sensitive information. We tend to think about that as attributes: where do I live, where do I go. But the information that we are deriving about you, the analytically derived information, is, in fact, a lot more sensitive and potentially private than anything you might know about just point places where I show up in my car. Versus when and where I do that or who I talk about. Looking forward to us finally expanding our definition of what should be considered private, sensitive, personal, moving forward.
CHRIS MCCLEAN: Yeah, absolutely.
KIMBERLY NEVALA: Before we let you go, I love this idea of, again, trying to always orient around an ethically positive perspective or trajectory. But it's certainly easy for those things to run aground or run afoul of our day-to-day processes and practices.
So what advice or practical actions would you leave with folks in the corporate realm? And would that be any different for folks working in public spaces?
CHRIS MCCLEAN: It will be similar.
I think the big thing for me is that when I talk about ethically positive outcomes, a lot of people are going to automatically assume that I'm talking about something like tech for good, which is technology that's used strictly for an altruistic purpose. We do a good deal of that work. We actually have a whole discipline in Avanade that is just tech for social good.
But what I'm talking about is more like our day-to-day technology that we interact with. Whether it's as a consumer or as an employee in the workplace, we can still aim for ethically positive outcomes in the technology that we're using for, let's say, health care, finance, HR, or our general performance management systems. All of these can still have ethically positive outcomes.
If you take the case of, let's say, accessibility. Making apps more accessible, mobile apps or things that we're using on our computers, accessibility is a good idea, ethically. It also increases your adoption and your user base. It allows more people to enter into the workforce and engage with the company in different ways.
So when you think about this idea of ethically positive outcomes, it's not just about altruism. For example, we had a company that we worked with that built a sort of a tracking system that was built specifically to keep people safe. That was helping them keep track of their hours so that they get paid more accurately. A lot of really positive outcomes could come from that. But nobody wanted to use it because they were nervous about the privacy implications. So by helping them walk through what does this system collect, how is it being used, how is it being controlled, that was a way to help increase adoption of that technology. We do that a lot.
We worked earlier this year with a mental health app that would allow employees to really help them achieve goals around mental health. And again, a big concern is that I'm interacting with the system as an employee. I'm providing very intimate details about how I live my life, my behaviors, my relationships and so forth. I'm not going to use that system if I think that data is going to be abused in any way. So in this case, creating good policies, good practices, good communication is going to help things like adoption, engagement, maybe grassroots discussion: helping get other people using that app because you've had a good experience.
Those are all good, I would say, tech outcomes. People that are building apps, they want better adoption and better engagement. And they want more people to talk about it with their friends.
If we do ethics right, a lot of times your business or technology objectives are more likely to be successful. So that's one way to get over that barrier of people being maybe put off by the idea of ethics. Or not wanting to spend that extra time or money to do that impact assessment. If you do it right, it's going to be better technology that people will want to engage with, that people will trust.
KIMBERLY NEVALA: Yeah, this is excellent. I want to thank you for joining us and providing these perspectives that help us expand our understanding of what the boundaries and even reason for digital ethics is. And frankly, for challenging us all to become more informed and ethically positive (my new favorite phrase) digital makers and takers. Thanks again for your time.
CHRIS MCCLEAN: Yeah, really great to be here. Terrific questions, and I really enjoyed the conversation. So I hopefully, we do it again sometime soon.
KIMBERLY NEVALA: Yes, we have a number of items we could spend another 40 to a couple of hours on, following up. So we'll definitely have you back.
In the meantime, next up, we're going to talk to Professor Mark Bishop for some plain talk about talking AI. This is going to be a frank discussion about generative AI. Including, of course, but not limited to, the hot app of the moment ChatGPT. Subscribe now, so you don't miss it.
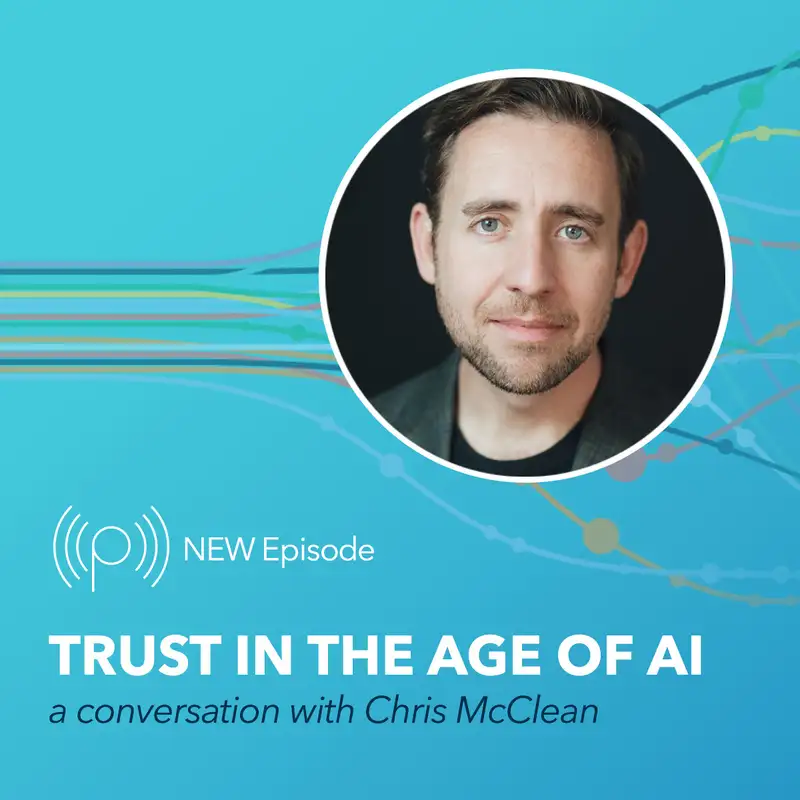